The market value of debt market versus book value of debt and returns of asset Online
Post on: 15 Январь, 2016 No Comment
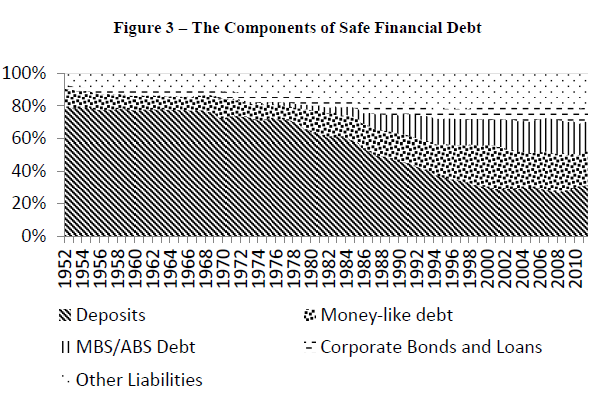
Page/Link:
Page URL:
HTML link:
Citations:
- MLA style: The market value of debt, market versus book value of debt, and returns of assets..www.thefreelibrary.com/The+market+value+of+debt%2c+market+versus+book+value+of+debt%2c+and. -a019717277
- Chicago style: The Free Library. S.v. The market value of debt, market versus book value of debt, and returns of assets..www.thefreelibrary.com/The+market+value+of+debt%2c+market+versus+book+value+of+debt%2c+and. -a019717277
Empirical research typically relies on book rather than market value of debt, though theory is virtually always in terms of market values. This paper documents how book value measurements of debt distort debt-equity ratios and cost of capital calculations. We focus on three key issues. First, mismeasurement can influence cross-sectional studies of capital structure, though the errors introduced may not be important because the cross-sectional correlation is very high each month between book and market-based measures. Second, mismeasurement can influence time-series studies of capital structure; this influence can be quite important. Third, mismeasurement can importantly influence calculations of cost of capital.
Empirical research typically relies on book rather than market value of debt, though theory is virtually always in terms of market value. This paper measures the market value of debt and assesses how using book value of debt as a proxy for market value of debt may have serious effects in empirical work. Book values sometimes, but not always, seriously mismeasure market values of debt.
We focus on three key empirical issues to explore how the use of book values of debt affects empirical results. We find that mismeasurement can influence cross-sectional studies of capital structure, that it can influence time-series studies of capital structure, and that calculating the costs of capital requires estimates of the value of debt.(1)
We measure the market value of debt by examining firms that have high-quality price data on a significant portion of their outstanding long-term debt. We show how some empirical results are sensitive to using the market value of bonds, but others are not, depending on the time period and questions considered. As an example, we compare estimates of capital structure that use book versus market value of debt. In the early part of the 1978-1991 period covered here, estimated long-term debt-to-value ratios based on book and market values of long-term debt diverge substantially.(2) As expected, differences in book and market capital structure are determined by changes in the level of interest rates, which implies biases in time-series studies. However, in cross-sectional studies intended to explain capital structure, use of book rather than market value of debt as the dependent variable introduces measurement error, but the associated problems may not be severe. Across 15 industries, the correlation of debt-to-value ratios using book and market values for bonds is over 0.95 for virtually every month. However, work with panel data using cross-sectional time-series methods can contain substantial measurement error, because time series of the book value of debt often deviate substantially from time series of the market value of debt. These deviations take place in major part because changes in yields affect the market value. (The market value can of course change for other reasons. For example, changes in a firm’s outstanding debt can affect the market value of the remaining old debt.)
The risk of equity or debt depends on how the two are mixed for financing the firm’s assets. In this sense, rather than looking separately at its debt and equity, the risk of the firm’s assets is likely to be the important factor in understanding the combined risk the firm faces. For example, the expected rate of return and volatility of a firm’s assets are more likely to be time-constant than those of either equity or debt alone, because equity and debt’s expected rates of return and volatilities vary not only with those of the firm’s assets, but also with changes in the firm’s debt-to-value ratio. Further, the majority of studies that consider equities and bonds together use book rather than market values of bonds, another possible source of error.
One illustration of these problems uses estimated costs of capital for 15 industries, determined in a capital asset pricing model (CAPM) approach using estimated market-model betas. The cost-of-capital estimates use either a constant beta fit for asset rates of return, or constant equity and debt betas fit separately. Differences in results across estimation methods can be large, ranging from 29% to 46% relative to asset-market sample risk premia, with differences in the estimates depending strongly on time variation in capital structures. In practice, bond prices are often not available. Bond betas are then guessed rather than estimated; on average, this leads to even greater discrepancies. If there are major changes in the capital structure over time, it is probably better to first estimate the asset cost of capital rather than starting with separate estimates of time-constant equity and debt cost of capital.
In Section 1, we discuss the bond data used and present our approach for estimating the market value of a firm’s debt. Section II presents two estimates of aggregate debt-value ratios for large firms that on average issue investment-grade debt. One estimate uses our measure of market values of debt, the other book values, and both use market values of equity. We also provide summary statistics describing the time series behavior of capital structure for 15 industries. Section III discusses and evaluates alternative ways of estimating asset betas and required rates of return to total assets. Section IV concludes the paper and provides suggestions for future research.
I. Bond Data and the Measurement of the Market Value of Debt
To estimate the market value of debt, we use a database of bond prices and other bond information available in the Lehman Brothers Fixed Income Data Base.(3) For the period 1978-1991, this database contains monthly information on almost all nonconvertible publicly traded debt issues that had a face value of at least one million dollars, and which were originally issued with an investment-grade rating (fallen angels, those bonds that have fallen to noninvestment-grade levels, are also included). Our approach is to estimate the end-of-month total value of a firm’s long-term debt by extrapolating from the market value of the portion of debt for which we can obtain good price data.(4)
About two thirds of the price data are trader bid quotes, which are the prices a Lehman Brothers trader is prepared to pay for lots of 500 or more bonds with a maturity value of $500,000 or greater. The remaining prices are so-called matrix prices, estimates of prices for infrequently traded instruments. These estimates are based on the prices of more liquid bonds (especially US Treasury issues) with similar coupons and maturity.
Warga (1991) and Warga and Welch (1993) discuss the various sources of bond-price data, including both trader quotes in the over-the-counter dealer market (e.g. Lehman Brothers) and transactions prices in the New York Stock Exchange’s electronic market. NYSE bond-price data are much more limited in coverage of firms than the over-the-counter dealer market; further, trading in the NYSE market is very thin for investment-grade bonds. Only 14% of publicly traded corporate debt is listed on the NYSE; of that debt, investment-grade issues comprise 73% of the listings but only 43% of the trading volume.(5) Our database gives both broader coverage than the NYSE and regular month-end pricing. Warga (1991) concludes that for valuation of broad-based portfolios, bond pricing is consistent across the dealer market (e.g. Lehman Brothers data) and the exchange market.
In each month of the sample period, we restrict ourselves to firms for which information is available on at least 50% of the outstanding issues of long-term debt. We obtain a firm’s book value of long-term debt from Value Line and compare this to the book value of that firm’s debt available in the Lehman Brothers’ bond-price database. For 70% of the resulting sample of firms, the bond-price data base contains prices for at least 75% of each firm’s long-term debt. For 42% of this sample, the database contains information on over 90% of each firm’s outstanding long-term debt. The firms meeting any of these screens show a strong tendency to continue to meet them month after month; there is little tendency for an individual firm to seesaw in and out of the sample. As an added precaution, we restrict the sample to firms that have at least 12 consecutive months of data.
On average, short-term debt accounts for about 5% to 6% of total capitalization.(6) Calculations here ignore variations in the market value of short-term debt for two reasons: First, data on market value are not available and would have to be estimated with methods much less precise than those used here for long-term debt. Second, because of short-term debt’s limited duration, its market value is less sensitive to interest-rate changes than is the value of long-term debt.
Financial accounting data and stock returns must be available from Value Line and CRSP, respectively. This gives a final sample of 401 firms with a minimum of one year of data available from all required sources.(7)
For the three cut-off percentages of long-term debt; if at least 50% of debt are required, there are 401 firms. If 75% are required, 275 firms. And with 90% required, 170 firms.
This sample is biased towards larger firms. Part of this bias is created because large firms are the major issuers of publicly traded debt. The bias is strengthened by restricting the sample to firms whose bonds have 12 consecutive months of price data; this filter is correlated with liquidity, and larger firms tend to be issuers of the most liquid bonds.
The sample firms are divided into 15 industry portfolios.(8) Table 1 shows the yearly average number of firms in the sample by industry; Panel A shows the 50% sample, Panel B the 90% sample. Table 1 also lists our industries and the SIC two-digit codes that go into each industry; all but three of our industries correspond to a single two-digit SIC code industry. (Mining includes five SIC codes; Other Retail Trade, nine; and Banking, Finance, and Real Estate, eight.)
[TABULAR DATA 1 NOT REPRODUCIBLE IN ASCII]
The scheme for estimating a firm’s market value of long-term debt each month is to form a portfolio of all of that firm’s bonds using trader-quoted prices;(9) we then adjust the portfolio’s duration to match the duration of all the firm’s debt shown in the database, regardless of whether the prices are quotes or matrix-based. Two bonds (or portfolios) with the same duration and identical default risk will have returns that are approximately the same. Thus we can obtain a return for a firm’s long-term debt that is restricted to trader-quoted prices and yet is representative of most of the firms’ long-term debt, in the sense that the return should be the same. A firm’s return to debt can be sensitive to the type of price that is employed. It is important to use only trader quotes for returns so that firm-specific events that are not implicitly captured by matrix prices do not mask the firm’s actual debt return.(10) The scheme is detailed further in Appendix A.
We repeat much of the analysis employing the 90% sample, which has data on virtually all long-term debt issues; results reported for our 50% sample are consistent with those for the 90% sample. For example, the observed differences in our measures of book and market value capital structures with the 50% sample slightly understate those with the 90% sample. Appendix A discusses these sensitivity tests.
II. Capital Structure: Book versus Market Value of Debt
This section examines estimates of capital structure for a sample of 15 industry portfolios, using both market and book values of debt. We form 15 industry portfolios by taking simple averages each month across all firms in a given industry.
A. Empirical Analysis
For the 1978-1991 period, Figure 1 plots two monthly measures of the simple average across industry portfolios of capital structures (ratio of long-term debt to total capitalization). One series estimates capital structure using book value of debt, as traditional studies have had to do because of lack of data on the market value of debt. The other series uses our estimated market values of firm debt (Section 1); both series use the market value of equity.(11)
[FIGURE 1 ILLUSTRATION OMITTED]
Total capitalization includes short-term debt valued at book: Short-term debt rarely comprises more than 6% of a firm’s debt capitalization;(12) its short duration means that book closely approximates market value. Preferred equity is rare in our sample and is not included in our measure of capitalization.(13) Contingent claims on the firm are not included because it is difficult to uncover such claims and estimate their values.(14) For example, we ignore the value of unexercised options that management holds in various incentive schemes,(15) the capitalized value of unfunded pension liabilities, and the capitalized value of actual and potential claims for product or other types of liability.(16)
In Figure 1, the difference between the book and market measures of capital structure ranges from just under zero to 7%; the 7% difference occurs in 1981 when book capital structure is under 30%. Thus, the difference in the two measures, relative to the book value measure is 23.3%. There is a break in the data from December 1984 through February 1985 caused by the break in bond-price data in December 1984.(17) Much of the work here is for the two sample periods created by this break.
In the period before the data break, the average book and market measures are 30% and 27%. Relative to the average book measure, the difference between the book and market average ratios is approximately 10%. In the period from March 1985 through in December 1991, both capital structures move together, and average about 30%.
There is substantial variation in corporate use of debt over the sample period. Figure 2 plots the average level across industries of book and market debt (both normalized relative to January 1978 values), along with the ratio of market-to-book debt. In Figure 2, this ratio ranges from 71% to 108%. Our sample covers a period in which the market does not often exceed the book value of debt. Because of scaling it is difficult to see in Figure 1 that market in fact exceeds book value in 20 of the 82 months represented in the 1985-1991 period.
[FIGURE 2 ILLUSTRATION OMITTED]
From the end of 1984 to the end of 1991, long-term debt levels increased by roughly 100%; from the start of 1978 to the end of 1991, long-term debt levels increased by roughly 200%. Of course, these firms were growing over these periods. Note that in comparison, gross domestic product rose 40% from 1985 to 1991,and by 152% from 1978 to 1991, suggesting firm growth does not fully account for debt growth. This suggests that corporate reliance on debt (for our sample of firms) increased over time. (In their 1995 study, Roden and Lewellen document a trend toward a heavier reliance on issues of debt employed in LBO financing over a sample period similar to ours.)
As expected the market value is less than the book value of debt in periods of high interest rates. Figure 3 plots the difference between Figure 1’s measures of book- and market-based capital structures, along with the yield on the Lehman Brothers index of long-term government bonds.(18) In large part, discrepancies arise when yields diverge from the coupon rates on bonds. If the yield rises, but thereafter remains constant, and if bonds carry coupons that make bond prices close to par when issued, then over time changes in the composition of debt bring market and book values together.
[FIGURE 3 ILLUSTRATION OMITTED]
For two subperiods, January 1978 to November 1984, and March 1985 to December 1991, Table 2 gives sample statistics for each industry for both the book and market value measures of capital structure, and also shows the ratio of the market value relative to the book value measure of capital structure. The book value measure must lie between zero and 100%; for the industries in this study, the book measure ranges from 6.95% to 64.05% (Industry 14, Banking, Finance, and Real Estate; and Industry 11, Electric Utilities, both in the first subperiod). For the second subperiod, the minimum and maximum book value ratios are 10.36% and 56.01% for the same two industries. The ratio of market value to book value capital structure is as low as 69.03% (Industry 5, Petroleum, in the first period), and as high as 109.09% (Industry 9, Appliances and Electrical Equipment, in the second period). For any one industry, the market-to-book ratio shows substantial differences between the industry’s maximum and minimum values. For example, in the first period, the petroleum industry’s (Portfolio 5) max-min range, relative to the mean ratio, is 35%; Industry 15’s (Gas Utilities) relative difference is 18.2%. These gaps are not the result of a large change in sample size. The maximum value is attained in April 1978 when there are 15 firms, and the minimum is in October 1981 when there are 17 firms.
[TABULAR DATA 2 NOT REPRODUCIBLE IN ASCII]
B. Implications for Capital Structure Studies
Many studies provide empirical evidence on firm capital structure, highlighting its importance for corporate tax purposes, signaling management intentions, equity rates of return, and more. Almost all of these studies use book values of debt. Using book values where market values of debt are appropriate introduces measurement error in the debt values. The severity of problems that measurement error causes depends in part on the size and variability of the error relative to movements in the theoretically appropriate variable.
The large differences sometimes detectable in our analysis (Figure 1, for example) occur in examining time series. Many tests of capital structure theories(19) are cross-sectional and may be insensitive to using book rather than market values for debt. Across the 15 industry portfolios, the cross-sectional correlations between book and market values of debt are almost always above 95% in each month of our sample period. We note that because the measurement errors discussed here are in the dependent variables, these errors by themselves reduce efficiency, but do not cause bias in coefficient estimates. Expected t-statistics are smaller with book rather than market data. Measurement error biases t-statistics against significance and thus does not undercut significant results reported in the literature. Of course, if the measurement errors in the dependent variable are correlated with independent variables, estimated slopes and t-statistics are biased, but in directions that can be predicted only through detailed analysis.
As an example of how book values may give information quite similar to market values, consider our portfolios 7 through 10 correspond to those firms that manufacture machines and equipment whose products require the availability of servicing and spare parts (SIC codes 3400-4000.) Titman (1984) argues that these firms will find liquidation especially costly, and lower debt structure can mitigate the problem bankruptcy poses to purchasers of the firms’ goods. While Portfolio 9 (Appliances and Electrical Equipment) has the lowest total market value-based debt structure in the first period (0.202 when adding in short-term debt), it is only third lowest (total debt structure 0.297) in the second period, with portfolios 2 (Food and Beverage, 0.25) and 4 (Chemicals, 0.284) besting it. Portfolios 7, 8,-and 10 are in the middle of the pack in both periods. We do not test Titman’s model here. Rather, our point is that employing book value for long-term debt would produce capital structure estimates that almost always result in the same rank ordering of capital structure as market value-based estimates; cross-sectional rank correlations between book and market capital structure are almost perfect. Studies similar to Titman’s (1984) may well be insensitive to use of book or market values of debt. However, research employing cross-sectional time series methods could suffer because errors in book measures of capital structure can be large and persistent (for example, the first periods in Figures 1 and 3).
III. Alternative Estimates of Industries’ Required Rates of Return on Assets
Having obtained time series on debt, equity, and (from the last section) market-based monthly capital structure data, we can now examine the return on assets to our industry portfolios. By calculating the cost of capital based on the returns to assets, we show that lack of data on the market value of firms’ long-term debt forces the compromises in empirical work that can result in misleading cost-of-capital estimates.
A common requirement is to find a firm’s cost of capital for its assets in place. For example, this method is used for valuing the firm in a take-over bid, or for judging the profitability of overall firm expansion. This is done most often by finding estimates of a firm’s equity and debt required rates of return and then taking a weighted average using debt-to-value weights. Often, the required rates of return are based on separate estimates of equity and debt betas that explicitly or implicitly assume a fixed capital structure over time. Most often, these approaches assume time-constant equity and debt betas, even though most observers argue that changes in leverage should have an important effect on these betas.
An alternative approach that we explore in this section is to find a single beta for the firm’s asset rate of return relative to an asset market index. If the asset rate of return is measured with appropriate capital structure ratios that change each month, then nonstationarity of equity betas generated by changing leverage is dealt with automatically.
Table 3 provides estimates of the cost of capital by industry, based on three models of required rates of return and over our two subsample periods. The large differences across the two subperiods, for any industry and for any one method of estimation, arise in part because risk-free rates of interest were substantially higher in the first than in the second subperiod (an average of 0.825% per month in the first, 0.515% in the second subperiod, or 60% larger in the first subperiod).
[TABULAR DATA 3 NOT REPRODUCIBLE IN ASCII]
Each model uses a CAPM-based approach to required rates of return, with estimated betas from market model regressions. Our interest is in using a model capable of responding to changes in capital structure. We choose the CAPM for its simplicity. Many papers argue that the CAPM is misspecified: Misspecification is not a problem here as long as it does not affect estimates of the effects of changing capital structure; indeed, complicating the model might add factors that confound the effects of changing capital structure. Fama and French (1994) estimate industry cost of equity (as opposed to asset cost of capital). They argue that based on previous work (Fama and French, 1993), a three-factor model should be employed: One factor is the market; a second is the difference between the returns on a portfolio of small-cap stocks and a portfolio of high-cap stocks; a third is the difference between the returns on a portfolio of high book-to-market equity (BE/ME) stocks and a portfolio of low BE/ME stocks. Because our sample has a large-firm bias, forming a portfolio of the returns to asset of large vs. small firms is not feasible. (Note that in a study of the cost equity, this paper’s results show the need to adjust for changing capital structure; even for industry portfolios, capital structure changes are important empirically.)
In Model A, we calculate time-constant equity and long-term debt betas [Beta.sub.e],[Beta.sub.db] in separate market models where the index is an equity market index (an equally weighted average of the 15 industries’ equity rates of return, discussed in Appendix B). Model B is the same as Model A, but the index is an asset market index (an equally weighted average of the 15 industries’ asset rates of return, discussed in Appendix B). In Model C, we calculate asset betas ([Beta.sub.asset]) for each industry’s asset rate of return in a market model where the index is the asset market index. The three models are shown in Table 4.
We estimate market premia as the sample mean rate of return to a market index over the 30-day T-bill rate for the entire 1978-1991 period. Monthly sample premia are: equity premium (EMIDX minus T-bill rate) = 0.00563/month; asset premium (AMIDX minus T-bill rate) = 0.00417/month; and 30-day T-bill average return = 0.0067/month. We calculate the required rates of return for models A and B according to the equation:
(1) E([r.sub.i]) = E/V E([r.sub.E]) + D/V E([r.sub.DL] + D/V [r.sub.f]
where E([r.sub.(.)])=expected rate of return, i=industry subscript, E=equity, [D.sub.L]=long-term debt, [D.sub.S]=short-term debt, V=E+[D.sub.L]+[D.sub.S], and [r.sub.f]=30-day-bill rate. As before, this assumes that firms’ short-term debt earns the riskless rate. Use of after-tax corporate debt rates of return to measure the firm’s cost of capital, as opposed to an investor’s required rate of return as in the text, leaves the results qualitatively unchanged. In these calculations for Models A and B, the values for E, [D.sub.L], and [D.sub.S] are those at the end of each sample period (November 1984 and December 1991). The industry expected rates of return for Model C are calculated from the CAPM, with asset betas developed directly from the market model of asset rates of return on the asset index.(20)
Note that Models A and B have better chances in Table 3’s comparison than can occur in actual practice. In both models the debt betas are estimated with ordinary least squares (OLS), rather than relying on analysts’ guesses, and both use the end-of-sample estimates of the market value of capital structure rather than the book value as happens in actual practice. Further, Model B uses an asset market index rather than an equity index as is usual in practice (and in Model A). Ordinarily, then, the usual methods of estimating firms’ asset betas are subject to larger errors than the Table 3 comparisons suggest.
Given the large variations in debt-value ratios discussed in Section I, and the general view in finance that equity and debt betas vary with changes in capital structure, Model C is preferable to Models A and B. Deviations from Model C are greater for Model A, which assumes stationary rates of return to both equity and debt, and also employs a market index that contains only equities.(21)
It is not surprising that the discrepancies between Models A and C are substantial relative to those between Models B and C. These differences arise from using EMIDX in Model A, AMIDX in Model B. Evidently, despite the close correlation of EMIDX and AMIDX (0.98, see Table 2), using an equity index can produce results that might be viewed as importantly different from those using an asset index. Absolute deviations average across industries are 0.5% and 0.37% in the first period, 0.95% and 0.55% in the second period, or Model A’s discrepancies relative to Model B’s discrepancy with Model C are 1.3514 in the first period and 1.7273 in the second.
Relative to estimates of the risk premium on the market, the range between the estimates from Model C and those from either Model A or Model B is substantial.(22) On a yearly basis, the sample excess rate of return on the asset market index is 5.004% [=(0.00417) (1200)]. Relative to this sample risk premium, the range of the difference between the Model A and Model C estimates is 28.98% [=(100) (1.45/5.004)] for the first subperiod and 45.56% [=(100) (2.28/5.004)] for the second subperiod. For Model B and Model C, the estimates are 30.78% [=(100) (1.54/5.004)] for the first subperiod, 40.37% [=(100) (2.02/5.004)] for the second subperiod. Relative to the estimated risk premium, the average absolute errors for Models A and C are 9.99% [=(100)(0.5/5.004)] for the first subperiod and 18.98% [=(100)(0.95/5.004)] for the second; for Models B and C, 7.39% [=(100) (0.37/5.004)] and 10.99% [=(100)(0.55/ 5.004)]. In other words, the risk-adjustment component of hurdle rates differs across estimates by an (absolute) average between 7.39% to 9.99% in the first subperiod and from 10.99% to 18.98% in the second subperiod. If the discrepancies are measured by range or average absolutes, then for both Models A and B the discrepancies are larger in the second subperiod than the first. In this sense, not taking account of time-varying leverage has more severe consequences in the second subperiod.
Table 5 shows estimates of equity, debt, and asset betas for both the equity and asset-market indices (EMIDX and AMIDX). By construction, the average equity beta for EMIDX equals unity, and the average asset beta for AMIDX equals unity. The average bond beta for AMIDX is substantially larger than for EMIDX (0.54 vs. 0.28 in the first period, 0.18 vs. 0.11 in the second). This is to be expected, because AMIDX includes bond rates of return and EMIDX does not.(23) For either index, bond betas are substantially larger in the first period than in the second; this may arise because the variance of bond rates of return was larger in the first period.(24)
[TABULAR DATA 5 NOT REPRODUCIBLE IN ASCII]
To evaluate whether the A-C and B-C discrepancies in Table 3 arise from changing capital structure, we correlate the size of the discrepancies with the distance of the capital structure from its average value (in each period) on the two dates employed to calculate the required return (November 1984 and December 1991). For each subperiod, Table 6 reports regressions of the squared deviation of each industry’s capital structure (for 11/84 and 12/91) from its mean value against the squared discrepancies of Models A and B from Model C. All four regressions show highly significant correlation between the variables, suggesting that the observed discrepancies in Table 3 arise in part from time-varying capital structures.(25)
The calculations in Table 3 may understate the differences in the three models of required rates of return. The analyst seldom has access to bond rates of return to use for calculating debt betas, and thus must guess debt betas, and often does not have market indices that include corporate bond market data and thus must use an equity market index, for example, the CRSP equally or value-weighted indices. Thus, as usually implemented, Model A can be thought of as inferior to Model C by using the wrong market index, by not having data on debt rates of return, and by estimating time-constant equity betas. Similarly, in practice, Model B can be thought of as inferior to Model C by not having data on debt rates of return, and by estimating time-constant equity betas, though with the right asset market index.
IV. Conclusion
Empirical research typically relies on book rather than market value of debt, though theory is virtually always in terms of market value. This paper assesses how the use of book rather than market value may have serious effects in empirical work. To do this, we construct time series on the market value of debt. Book value sometimes, but not always, seriously mismeasures the market value of debt; this mismeasurement is associated with changes in bond-market yields.
This paper focuses on 15 industry portfolios over the period 1978 to 1991. We estimate monthly time series of firm capital structure, based on book and market value measures of debt, and use these to estimate industry and overall debt-to-value ratios. We find important differences in the time series behavior of book- versus market-based capital structure. We show how use of book value capital structure may cause few problems in cross-sectional studies of capital structure theories. Cross-sectional correlations between book- and market-based capital structure are always very high for any month. We argue that time-series cross-sectional studies may be subject to more serious problems. We also illustrate how conventional approaches to estimating asset cost of capital can result in serious mismeasurement.
Results in this paper suggest a number of directions for research. First, many important issues in finance deal with the value of a firm’s assets, not just its equity or debt, or deal with distribution effects across equity and debt claims on the firm’s assets. An example of questions about distribution is whether takeovers that enhance equity value do so by reducing debt value by the same amount (Warga and Welch, 1993). Similarly, analyzing the strengths and weaknesses of Chapter 11 bankruptcy proceedings requires analysis of both debt and equity (Eberhart et al. 1990; Eberhart and Sweeney, 1992 and 1995).
Second, a substantial part of our knowledge of empirical finance turns on event studies that use market-based abnormal returns to equity. Some of the theories tested actually make predictions about firm value, not just equity value; other theories have implications for both debt and equity values. It is important to know which theories are supported by market-based bond price data.
Third, time-series data on firms’ equity rates of return are likely higher quality than their debt rates of return. Debt data add considerable cross-sectional variation, however. Studies using both types of data may well be more informative than those using only one type.
(1) See Alderson and Betker (1996) for an example of the potential for mismeasurement of liquidation costs when using accounting rather than market-based measures.
(2) Past work on this issue tends to focus on a single period. Bowman (1979), for example, provides detailed measures of market-based capital structure for 90 firms for year-end 1973.
(3) Obtained from the Fixed Income Research Program at the University of Wisconsin-Milwaukee. See Warga (1995).
(4) The Lehman Fixed Income Data Base provides the amount outstanding of listed bonds. We obtain the total amount outstanding (book value) of long-term debt from Value Line. Brainard, Shoven, and Weiss (1980) estimate annual market values of firms’ debt with methods that are somewhat similar to those used here. Importantly, they never use observations on bonds’ market values. They infer the market value of bonds by assuming that in each year the firm’s bonds are distributed by maturity as reported in Compustat (amount maturing in each of the first five years and one figure for all debt maturing in more than five years); the structure remains the same way as in the initial year of the sample. All bonds are priced at par when issued and all are initially of 20 years maturity with constant coupon payments; all bonds are rated Baa; and the market value of short-term debt is equal to took value. Bernanke and Campbell (1988) and Bernanke, Campbell, and Whited (1990) use procedures quite similar to those of Brainard, Shoven, and Weiss.
(5) Based on 1993 NYSE data.
(6) These figures exclude firms in the banking and finance sector. In their total capitalization, banks and finance companies in our sample averaged about 64% short-term debt.
(7) Under the 50%, 75%, and 90% criteria. the major restriction on firms comes from the bond database. Financial data from the Value Line database provides us with information on total debt outstanding; employing Compustat results in similar restrictions on the final sample. Firms that do not appear in Value Line generally are not large enough to issue publicly traded debt. Value Line covers roughly the largest 1600 firms. Using Compustat NASDAQ tapes, or NASDAQ companies for that matter, will not affect our final sample size very much because these firms rarely issue debt that qualifies for the bond database. We check for firms that are included once in the Value Line database and then subsequently drop out; in this study the number of such firms is trivial.
(8) Originally, we formed 20 portfolios. Five were eliminated because they averaged less than two firms per month in the 50% sample, and had almost no firms available in most months for the 90% sample. These portfolios, with SIC codes in parentheses, were Textile and Apparel (22, 23); Stone, Clay, and Glass (32); Miscellaneous Manufacturing (38, 39); Railroads (40); Other Transportation (41,42,44,45,47).
(9) See Chatterjee et al. (1996) for a recent example in which bond prices are restricted to trader quotes when feasible. Our final estimate of a firm’s market value of debt (detailed in Appendix A) inflates/deflates the book value of long-term debt recorded in Value Line (which includes long-term bank debt) by whatever factor the market value of available debt is relative to the book value. We chose Value Line over Compustat partly because of the fact that quarterly (as opposed to annual) changes in book value of debt was available beginning in 1985 (earlier than Compustat).
(10) In this paper we assume that the data we have on long-term debt maturity for all our instruments (both matrix- and trader-quoted) are representative of a firm’s long-term debt maturity structure. Detailed analysis of the maturity structure of firm debt is beyond the scope of this paper. Stohs and Mauer (1996) analyze the debt maturity structure of 328 firms for the sample period 1980-1989. Much of the data listing the maturity dates of individual debt instruments must be hand-collected.
(11) See Raad and Ryan (1995) for an example in which employing the book value of equity may be the preferred method.
(12) Again, the exception is the Banking, Finance, and Real Estate sample, which averaged about 64% short-term debt in total capitalization.
(13) The database contains book values of preferred, but this type of security can be option-laden. For example, a frequent option is convertibility into common stock subject to various triggers. Valuing such options is outside the scope of this paper.
(14) Scholes and Wolfson (1992) examine bond-like contingent claims and their links to changes in capital structure induced by changing tax rates. Petersen and Rajan (1995) discuss trade credit as a substitute for debt financing.
(15) These values are usually small relative to the market values of assets that we use for the relatively large firms in this paper’s sample.
(16) These values can be large relative to the market values of debt and equity, even for the large firms in the sample used here. General Motors had unfunded pension liabilities with an estimated value of $22 billion at the start of May, 1994. Johns-Manville went into Chapter 11 bankruptcy over its liability for asbestos health claims. Texaco went into Chapter 11 after losing a civil suit concerning a takeover agreement.
(17) Returns for January 1985 cannot be created because of the absence of bond prices in December 1984. A lag in capital structure necessitated by the use of beginning-of-month figures produced the February 1985 missing data. See Warga (1995) for documentation of the missing data in our bond database.
(18) The Lehman Brothers Long-term Government Bond Index includes all US government guaranteed bonds and notes with a minimum outstanding principal of $25 million and a minimum maturity of one year, excluding flower bonds and foreign-targeted issues.
(19) See Titman and Wessels (1988) for a survey of previous tests and a comprehensive empirical examination of capital structure theories.
(20) Autocorrelations for industry equity and asset rates of return are almost all insignificant. In contrast, industry bond rates of return exhibit significant first-order autocorrelation in the first subperiod, and some in the second. In the 1978-1984 period, ten of 15 industry portfolios have first-order autocorrelation coefficients significant at the 0.05 level, and 14 at the 0.10 level; the 15 coefficients’ values range from 0.17 to 0.252. In the 1984-1991 period, only one portfolio has significant autocorrelation at the 0.05 level, and five are significant at the 0.10 level; values range from -0.04 to 0.227. This raises the possibility that econometric adjustment to bond beta estimates might affect this section’s conclusions. Rank correlation between the magnitude of the discrepancies reported at the bottom of Table 3 and the degree of autocorrelation is negative and insignificant (-0.27 in the first period); the firms with the largest discrepancies are the ones that probably do not require any econometric adjustment.
(21) Model A deviates from Model C on average by 0.5% (absolute deviation) in the first subperiod, with a range of 1.45%, from +1.14% to -0.31%; on average (absolute) by 0.95% in the second subperiod, with a range of 2.28%, from 1.9% to -0.38%. Model B deviates from Model C on average (absolute) by 0.37% in the first subperiod, with a range of 1.54%, from 1.05% to -0.49%; on average (absolute) by 0.55% in the second subperiod, with a range of 2.02%, from 1.40% to -0.62%.
(22) If we assume that the risk premium does not vary importantly or systematically with the risk-free rate, comparison to the risk premium is more appropriate than to risk-adjusted industry hurdle rates that include the effect of the level of the risk-free rate.
(23) The correlation of the long-term bond rate of return is 0.43 with AMIDX but only 0.3 with EMIDX (see Table B.1).
(24) A question often asked in the asset pricing literature is whether beta is correlated with expected return as predicted by theory. Some authors have found that empirically, equity betas are not correlated with expected equity returns. When we correlated asset betas from Table 5 with our estimates of expected returns to assets from Table 3, we also find that there is no significant relationship. Unlike the asset-pricing literature, our experimental design does not maximize dispersion in asset betas, so there remains the possibility that a significant relation might still exist. This will be explored in future research.
(25) Employing absolute deviations instead of squared deviations produces similar results, as does the use of rank correlation. (See Table 6.)
(26) For example, if 95% of the bonds are present, we multiply the total value of debt for observed instruments by 1/0.95. Recall that this subsample of firms has at least 90% of a firms’ bonds available.
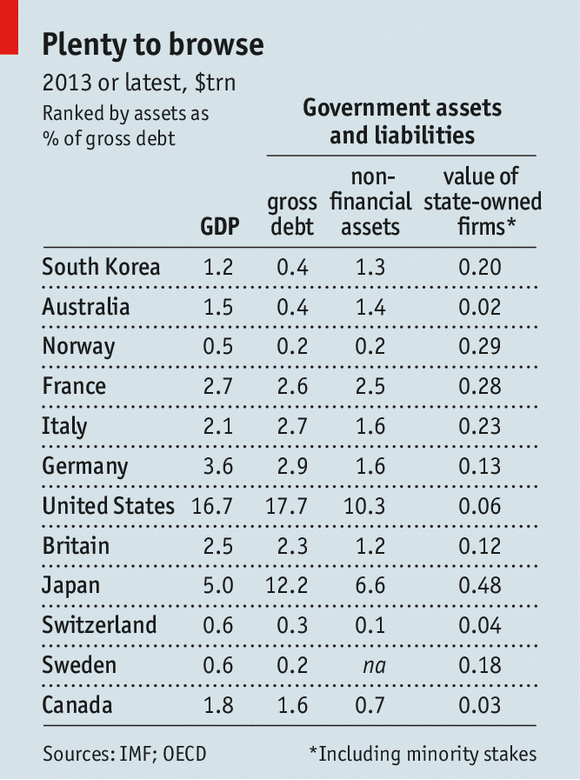
(27) Based on bid prices from the CRSP Government Bond Data Base. Use of bid-based returns maintains consistency with corporate bond returns, which are calculated with bid quotes (Section 1).
(28) We use equal weighting of firms within an industry both to create an industry portfolio, and also across industries to compose the market indices. In both cases, equal weighting helps avoid some of the biases inherent in the database. As Table 1 shows, the number of firms that qualified for each industry varies substantially, and it is not clear that this is in proportion to firms’ actual representation in the economy. We omit from this study firms with no long-term debt because they would be disproportionately represented (they do not have to pass the 50% or 90% data filters): the bulk of such firms appear in the Banking, Finance, and Real Estate sector.
(29) For each month and each of the 15 industries, we find an estimate of the industry asset rate of return as an equally weighted average of firm asset rates of return for each firm in the industry. We include this in the month’s 50% sample, as defined in Section I. This average is the industry asset rate of return. The asset market index is the equally weighted average across the 15 industries of the industry asset rate of return. In symbols, the asset rate of return on firm j in industry i in period t is
[R.sub.j,i,t] = ([E.sub.j,i,t]/[V.sub.j,i,t])[R.sub.j,i,t] + ([D.sub.L,j,i,t]/[V.sub.j,i,t])[R.sub.dl,j,i,t] + ([D.sub.S,j,i,t]/[V.sub.j,i,t])[R.sub.ds,j,i,t]
where [E.sub.j,i,t] is the market value of firm j’s equity at the start of period t and [R.sub.j,i,t] the rate of return on equity over the period, similarly for [D.sub.L,j,i,t] and [R.sub.dl,j,i,t] for long-term debt and [D.sub.S,j,i,t] and [R.sub.ds,j,i,t] for short term debt, with the firm’s market value [V.sub.j,i,t] = [E.sub.j,i,t] + [D.sub.L,j,i,t] + [D.sub.S,j,i,t]. The asset rate of return for industry i in period t and the asset market index for period t are
[MATHEMATICAL EXPRESSION NOT REPRODUCIBLE IN ASCII]
where [N.sub.i,t] is the number of firms in industry i in t. For comparison purposes, the industry equity rate of return for industry i in period t and the equity market index in period t are
[MATHEMATICAL EXPRESSION NOT REPRODUCIBLE IN ASCII]
(30.) Serial correlations for the CRSPVW, CRSPEW, EMIDX, and AMIDX indices are similar to each other and largely insignificant. An exception is in the second sample period, when CRSPEW exhibits significant first-order serial correlation, but EMIDX does not. This probably reflects the large-firm bias in EMIDX relative to CRSPEW.
References
Alderson, M.J. and B.L. Betker, 1996, Liquidation Costs and Accounting Data, Financial Management (Summer), 25-36.
Bernanke, B.S. and J.Y. Campbell, 1988, Is There A Corporate Debt Crisis? Brookings Papers on Economic Activity (No. 1), 83-125.
Bernanke, B.S. J.Y. Campbell, and T.M. Whited, 1990, US Corporate Leverage: Developments in 1987 and 1988, Brookings Papers on Economic Activity (No. 2), 255-287.
Bowman, R.G. 1979, The Theoretical Relationship Between Systematic Risk and Financial (Accounting) Variables, Journal of Finance (June), 617-630.
Brainard, W.C. J.B. Shoven, and L. Weiss, 1980, The Financial Valuation of the Return to Capital. Brookings Papers on Economic Activity (No. 1), 453-502.
Chattetjee, S. U.S. Dhillon, and G.G. Ramirez, 1996, Resolution of Financial Distress: Debt Restructurings via Chapter 11, Prepackaged Bankruptcies, and Workouts, Financial Management (Spring), 5-18.
Eberhart, A.C. W.T. Moore, and R.L. Roenfeldt, 1990, Security Pricing and Deviation from the Absolute Priority Rule in Bankruptcy Proceedings, Journal of Finance (December), 1457-1469.
Eberhart, A.C. and R.J. Sweeney, 1992, Does the Bond Market Predict Bankruptcy Settlements? Journal of Finance (July), 943-980.
Eberhart, A.C. and R.J. Sweeney, 1996, A Note on Noise in Bankrupt Firms’ Securities, Journal of Banking and Finance (March), 401-416.
Fama, E.F. and K.R. French, 1993, Common Risk Factors in the Returns on Stocks and Bonds, Journal of Financial Economics (February), 3-56.
Fama, E.F. and K.R. French, 1994, Industry Costs of Equity, Center for Research in Security Prices, University of Chicago Working Paper.
Petersen, M.A. and R.G. Rajan, 1995, Trade Credit: Theories and Evidence, Northwestern University Unpublished Manuscript.
Raad, E. and R. Ryan, 1995, Capital Structure and Ownership Distribution of Tender Offer Targets: An Empirical Study, Financial Management (Spring), 46-56.
Roden, D.M. and W.G. Lewellen, 1995, Corporate Capital Structure Decisions: Evidence From Leveraged Buyouts, Financial Management (Summer), 76-87.
Scholes, M.S. and M.A. Wolfson, 1992, Taxes and Business Strategy, Englewood Cliffs, NJ, Prentice-Hall.
Stohs, M.H. and D.C. Mauer, 1996, The Determinants of Corporate Debt Maturity Structure, Journal of Business (July), 279-313.
Titman, S. 1984, The Effects of Capital Structure on a Firm’s Liquidation Decision, Journal of Financial Economics (March), 137-151.
Titman, S. and R. Wessels, 1988, The Determinants of Capital Structure Choice, Journal of finance (June), 1-19.
Warga, A. 1991, Corporate Bond Price Discrepancies in the Dealer and Exchange Markets, Journal of Fixed Income (December), 7-17.
Warga, A. 1995, A Fixed Income Data Base, University of Wisconsin-Milwaukee Fixed Income Research Program Working Paper.
Warga, A. and 1. Welch, 1993, Bondholder Losses in Leveraged Buyouts, Review of Financial Studies (December), 959-982.
Appendix A. Construction of Industry Market Value Bond Indices
We use all available long-term debt information to calculate each firm’s weighted-average debt duration. The term duration here refers to Macaulay duration, or the weighted average of time to receipt of a bond’s cash flows. The weights are defined as the percentage of the bond price in present-value terms represented by each cash flow, and where it is assumed that the bond’s yield to maturity provides the correct discount rate. The weighted-average duration of a portfolio of bonds is a close approximation of the portfolio’s duration. Two bonds (or portfolios) with identical default risk will have returns that are approximately the same if they have the same duration.
A firm’s return to debt is sensitive to the type of price that is used. It is important to use only trader quotes for returns so that firm-specific events that are not implicitly captured by matrix prices do not mask the firm’s actual debt return. Most prices reported in Moody’s or Standard and Poor’s bond guides are matrix-based, as are the quotes from commercial pricing services that offer data at daily or weekly frequencies.
The level of debt is not as sensitive to the use of matrix versus trader quotes. Nevertheless, our scheme uses only trader quotes for this quantity. The sensitivity tests described below reassure us that our chosen method produces conservative measures of debt value relative to book value. The logic of our approach is to create a weighted average of trader-quoted bonds that have the same duration as the weighted average of all of a firm’s available debt (i.e. including the matrix-priced issues). Like debt level, the duration of a bond is not very sensitive to the matrix/trader-quote price issue (again, relative to return).
After a firm’s target duration (weighted-average duration) is calculated by using all available information, we use a weighted average of the prices and returns to bonds with trader-quoted prices to calculate our final estimate of the firm’s monthly debt value and return to debt. We calculate the equally weighted average of all trade quoted bond durations that are above and below the firm’s target duration, respectively. There is a unique set of weights that, when applied to these two figures, match the firm’s target duration. This weighting scheme is applied to the trader-quoted prices and returns to produce the final estimate of a firm’s return to debt and debt value. We exclude from the analysis any bonds that could feasibly be called.
To illustrate how this scheme works, suppose a firm has seven bonds outstanding and that in a particular month three of them are matrix-priced and four are trader-quoted. Table A.1 provides each bond’s characteristics in the given month. The value-weighted average duration of all seven bonds is 5.275.
Table A.1. Illustration of Construction of Bond Value Estimates
M = matrix price, TQ = trader quote. The bond price is full price, or price including accrued interest. Weighted average duration of all bonds is 5.275 years. Equally weighted average duration of trader-quoted bonds below 5.275 is 2.5 (one bond). Equally weighted average duration of trader-quoted bonds above 5.275 is 6.5 (three bonds).
The equally weighted average duration of trader-quoted bonds above 5.275 is 6.5 ((6+6.5+7)/3) and the equally weighted average duration of trader-quoted bonds below 5.275 is 2.5 (only one bond). From this we get a weight by solving 2.5 x+6.5 (1-x) = 5.275, so x=0.30625. Equal weighting of bonds below (above) the weighted-average duration of all bonds is chosen at this stage because the trader-quoted bonds may not have value weights that are representative of all of the firm’s bonds below (above) the weighted-average firm debt duration.
At this point, we determine the estimate of the market value of the firm’s debt by taking the weight 0.30625 and multiplying it by the price (100) of the only trader-quoted bond below the firm average duration. We add that to the average price of the three trader-quoted bonds with above average duration ((110+95+105)/ 3=103.33) times the weight (1-.30625), which yields a price of 102.31. The resulting price now represents the price per bond of all of the firms’ debt. The firm’s total debt value would be calculated by taking the number 1.0231 and multiplying it by the total book value of debt outstanding. We use the same scheme to calculate the firms’ return on debt in the given month, using returns to individual trader quoted bonds instead of price where appropriate.
Our weighting scheme is a first-order Taylor series approximation of a firm’s return to debt (by the properties of Macaulay duration). While there is justification for using this scheme to determine the return to debt, it is an ad hoc procedure for determining the level of debt. We therefore run a variety of sensitivity tests to assess whether we may be over- or understating the true market value relative to book value for debt levels. Consider the following, one of the tests that reassure us that we are understating the true discount to book value in most of our sample period. We restrict ourselves to the 170 firms (42% of total sample firms) for which we have information on at least 90% of the long-term debt. We use both matrix and trader-quoted prices and compare the resulting total firm debt value to the one produced with the scheme described above (using trader quotes). All figures are grossed up to adjust for whatever percentage of bond data is available.(26) For this portion of the sample, we find that the trader-quote-only estimation scheme produces measures of market value of debt that understate the alternative approach by less than 10% on average. In other words, in the work reported in the text, we are understating the degree to which the true market value of debt should be discounted relative to the book value.
Appendix B. Market Indices
The market indices most frequently used in empirical studies in finance provide averages of rates of return on equity: the CRSP equally and value-weighted equity market rates of return, the S&P 500 rate of return on equity, and others. The main justification for focusing on equity market indices is that it is difficult to find market values of corporate debt. Appendix B discusses the construction and properties of an asset market index that explicitly incorporates market value data on both equities and corporate debt. For comparison, the Appendix also discusses an equity market index that uses the same firms as does the asset market index.
For each finn for each month, we gather estimates of market values of the rates of return on both its (long-term) debt and equity, and market values of its debt and equity. The rate of return and the market value of the firm’s equity are from CRSP; the rate of return and the value of the firm’s long-term debt are calculated as discussed in Section I and Appendix A; the firm’s short-term debt is included in the asset weights, is valued at book and is assumed to earn the risk-free rate of interest, measured as a 30-day T-bill yield.(27) These data are used to calculate a time series of the value-weighted average rate of return for the firm, which is the firm’s asset rate of return. For each month and each of the 15 industries, we find an estimate of the industry asset rate of return as an equally weighted average of firm asset rates of return for each firm in the industry. We include this in that month’s 50% sample, as defined in Section I. The asset market index is the equally weighted average across the 15 industries of the industry asset rate of return.(28) The equity market index is an equally weighted average of industry equity rates of return, with each industry index an equally weighted average of equity rates of return.(29)
Table B.1 provides descriptive statistics for the asset and the equity market indices (AMIDX and EMIDX); the CRSP equally and value-weighted equity market indices, including dividends (CRSPEW and CRSPVW); the Lehman Brothers Long-Term Treasury bond market rate of return index (LBLNGTR); and the risk-free rate measured as the 30-day T-bill yield. The three equity market indices and the asset market index are all highly cross-correlated, with the lowest correlation 0.943 (CRSPEW and CRSPVW.)(30)
Table B.1. Descriptive Statistics for Alternative Market indices
CRSPEW and CRSPVW: CRSP equally and value-weighted equity market indices, including dividends. AMIDX and EMIDX: Asset market and equity indices, calculated from CRSP, Value Line, and Lehman Brothers data, as described in Appendix B. LBLNGTR: Lehman Brothers long-term bond index (rate of return). RISKFREE: Risk-free rate, measured as a 30-day US T-bill yield. The table period is 1978.01-1991.12. N=165.
The authors wish to thank two anonymous referees, the Editors, and seminar participants at Case Western Reserve University, University of California-Berkeley, Stanford University, and the 1995 Financial Management Association Annual Meeting in New York for their helpful comments.
Richard J. Sweeney is Sullivan/Dean Professor of Finance in the School of Business at Georgetown University. Arthur D. Warga is Sheldon B. Lubar Professor of Finance at the University of Wisconsin-Milwaukee, Drew Winters is an Assistant Professor of Finance at the University of Southern Mississippi.