EDHECRisk In Defense of Optimization The Fallacy of 1
Post on: 5 Июль, 2015 No Comment
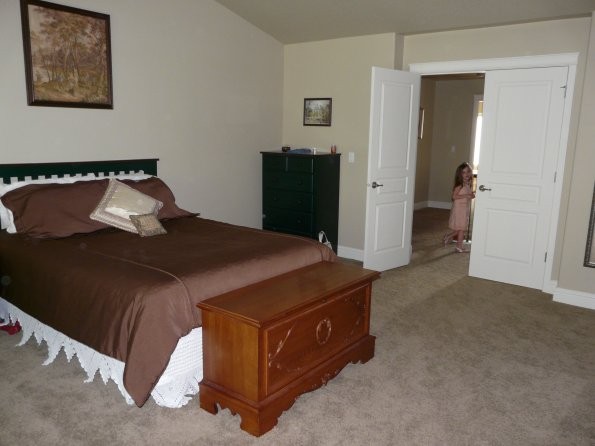
In Defense of Optimization: The Fallacy of 1/N
Authors: Mark Kritzman, Sйbastien Page, David Turkington
Source: Financial Analysts Journal, vol. 66, n°2
Date: March/April 2010
This article received the Graham and Dodd Scroll Award from the Financial Analysts Journal .
Several studies have shown that equally-weighted portfolios outperformed portfolios constructed using optimisation techniques (cf. for example, Jobson and Korbie, 1981; DeMiguel, Garlappi and Uppal, 2009; Duchin and Levy, 2009). This result can be explained by some characteristics of equally-weighted portfolios including, among others, non-concentration and over-weighting of small cap stocks. In addition, equal-weighting is easy to perform as it assumes no specific information about asset risk and return. On the contrary, the use of optimisation techniques requires reliable input data. However, lots of sophisticated techniques have been introduced to avoid the drawback of maximising input errors (cf. Jorion, 1986, 1991; Michaud, 1998; Black and Litterman, 1992; Chow, 1995; DeMiguel, Garlappi, Nogales and Uppal, 2009; Chevrier and McCulloch, 2008).
In the present article, Kritzman, Page and Turkington have a different point of view. They argue that optimised portfolios based on naпve inputs may outperform equally-weighted portfolios. Previous research had reached an opposite conclusion, unless informed inputs were used in portfolio optimisation. According to Kritzman, Page and Turkington, data input error maximisation is marginal when explaining the poor performance of optimised portfolios compared to equally-weighted portfolios in those studies. Rather, the authors stress that the length of historical data serving to compute expected returns – usually between 60 and 120 months – was too short in a vast majority of these studies. Thus, for the present study, they choose to base their estimations on long-term data history, using data starting as far back as 1926 for some asset classes.
They build up to 50,000 optimised portfolios gathered in three categories – asset class portfolios, beta portfolios and alpha portfolios – depending on the investment process. Those portfolios were derived to maximise the expected Sharpe ratio and hold over a five-year period with an annual rebalancing. No constraints were used other than long only ones and expected returns were estimated using simple approaches rather than econometric models, based on long data history including out-of-sample data. The expected volatilities and covariances were estimated using rolling period of five, ten and twenty years, as well as the whole sample data. They compared the performance of optimised portfolios with that of the market portfolio and the equally-weighted portfolio. Optimised portfolios include the minimum variance portfolio, the constant risk premium portfolio based on asset risk premium estimated over a long term period.
Their results show that optimised portfolios significantly outperform the market portfolio and the equally-weighted portfolio in terms of their Sharpe ratio. The results are obtained whatever the length of the data history used to estimate volatility and for all kinds of portfolio (asset class portfolios, beta portfolios, alpha portfolios). The authors mention that transaction costs only marginally affect the performance results, as all strategies considered have a low turnover. The authors are conscious that it may sometimes be difficult to have sufficient data to evaluate long-term risk premiums. In those cases, they recommend using a “reasonable” set of expected returns, rather than estimations based on samples that are too short, as they do not necessarily anticipate the future economic context.
In order to appreciate the diversification of the portfolios produced by optimisation, they evaluate the risk concentration as the portfolio volatility divided by the weighted average of individual asset volatilities. They obtain a risk concentration of 56%, on average, for optimised portfolios, versus 63% for the equal-weighted portfolio. The authors conclude saying that the results they provided with relatively naпve techniques for optimisation and input data estimation, should further be improved with more sophisticated approaches both for estimation and optimisation. Concerning data estimation, they suggest partitioning data samples in turbulent and quiet regimes, following Chow, Jacquier, Kritzman, and Lowry (1999) and Kritzman, Lowry, and Van Royen (2001) and to use the right combination of those two regimes depending on the expectation of future market conditions. They also suggest computing portfolios using full-scale optimisation, instead of mean-variance optimisation. This technique allows for the consideration of not only normal return distributions, but also all sorts of return distributions, as well as any description of investor preferences. According to them, all these techniques will further improve the dominance of optimised portfolio performance over equal-weighted portfolio performance.
References
Black, F. and R. Litterman. 1992. Global portfolio optimization. Financial Analysts Journal 48(5): 28-43.
Chevrier, T. and R. E. McCulloch. 2008. Using economic theory to build optimal portfolios. Working paper, University of Chicago (April).
Chow, G. 1995. Portfolio selection based on return, risk, and relative performance. Financial Analysts Journal 51(2): 54-60.
Chow, G. E. Jacquier, M. Kritzman, and K. Lowry. 1999. Optimal portfolios in good times and bad. Financial Analysts Journal 55(3): 65-73.
DeMiguel, V. L. Garlappi, and R. Uppal. 2009. Optimal versus naive diversification: how inefficient is the 1/N portfolio strategy? Review of Financial Studies 22(5): 1915-1953.
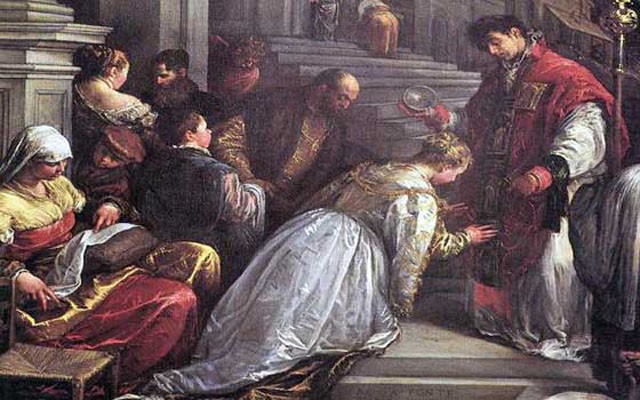
DeMiguel, V. L. Garlappi, F. J. Nogales, and R. Uppal. 2009. A generalized approach to portfolio optimization: improving performance by constraining portfolio norms. Management Science 55(5): 798-812.
Duchin, R. and H. Levy. 2009. Markowitz versus the Talmudic portfolio diversification strategies. Journal of Portfolio Management 35(2): 71-74.
Jobson, J. D. and B. Korbie. 1981. Putting Markowitz theory to work. Journal of Portfolio Management 7(4): 70-74.
Jorion, P. 1986. Bayes-Stein estimation for portfolio analysis. Journal of Financial and Quantitative Analysis 21(3): 279-292.
Jorion, P. 1991. Bayesian and CAPM estimators of the means: implications for portfolio selection. Journal of Banking & Finance 15(3): 717-727.
Kritzman, M. K. Lowry, and A.-S. Van Royen. 2001. Risk regimes, and overconfidence. Journal of Derivatives 8(3): 32-42.
Michaud, R. O. 1998. Efficient asset management: a practical guide to stock portfolio optimization and asset allocation. Cambridge, MA: Harvard Business School Press.
URL for this document: