The predictive power of yield spread evidence from wavelet analysis
Post on: 16 Март, 2015 No Comment
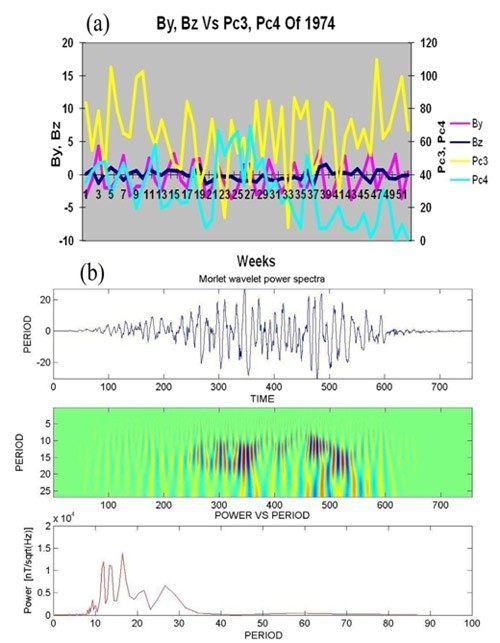
Page 1
123
Empirical Economics
Journal of the Institute for Advanced
Studies, Vienna, Austria
ISSN 0377-7332
Empir Econ
DOI 10.1007/s00181-013-0705-6
The predictive power of yield spread:
evidence from wavelet analysis
123
all rights are held exclusively by Springer-
Verlag Berlin Heidelberg. This e-offprint is
for personal use only and shall not be self-
archived in electronic repositories. If you wish
to self-archive your article, please use the
accepted manuscript version for posting on
your own website. You may further deposit
Arif Billah Dar · Amaresh Samantaraya ·
Firdous Ahmad Shah
Received: 12 June 2012 / Accepted: 5 February 2013
© Springer-Verlag Berlin Heidelberg 2013
Abstract This paper examines whether the spread between long- and short-term
interest rates contains information about future economic activity in India. Using
the yields on securities with maturities ranging from three months to ten years, we
1 Introduction
Information about a country’s future economic activity is important to consumers,
investors, and policy makers. A large portion of the recent macro-finance literature
suggests that financial variables have useful information content in predicting future
economic activity. The advantage of forecasts based on financial variables is based on
the fact that these forecasts are simple to implement, the data are readily available and
less prone to measurement errors. One financial variable that has been particularly
becoming popular in forecasting real economic activity is the difference between
long-term and short-term risk-free interest rates, usually known as the yield spread.
Yield spread has an edge over simple interest rates because short-term rates do not
contain all the information that can help to predict future economic activity. The
yield spread has been found useful for forecasting such variables as output growth,
inflation, industrial production, consumption, and recessions, and the ability of the
spread to predict economic activity has become something of a stylized fact among
macroeconomists.
Considerable research has been carried out examining the relationship between
yield spread and future economic activity. Stock and Watson (1989) found that yield
spreads were important to be included in their newly constructed index of leading
economic indicators. Estrella and Hardouvelis (1991) have thoroughly studied the
leading indicator property of the yield spreads for the economic activity in the United
States (US), and according to them, the slope of the yield curve is positively related
to future economic activity in the US. According to the influential paper in this line
of research by Estrella and Mishkin (1997), the term spread has significant predictive
power for both real activity and inflation in four major European countries (France,
Germany, Italy, and the UK). Bonser-Neal and Morley (1997), after examining eleven
developed economies, found that the yield spread is a good predictive instrument for
future economic activity. In the same vein, Venetis et al. (2003) reached the same
conclusions, as did Hamilton and Kim (2002). Ang et al. (2006), after modeling
regressor endogeneity and using data for the period 1952–2001, conclude that term
spreadhaspowertopredicteconomicactivity.BordoandHaubrich(2008)examinethe
predictive power of the yield curve in the US over the period from 1875 to 1997. They
findthatrealgrowthcanbepredictedmoreaccuratelyusingboththelevelandtheslope
of the yield curve. Other studies that link yield spread and future economic activity
such as Peel and Taylor (1998), Fisher and Felmingham (1998), Dotsey (1998), and
Stock and Watson (2003) also confirm the ability of yield spread to predict economic
activity.
While most of the studies find yield spread as good predictor of economic activity,
Page 5
The predictive power of yield spread
premium in yield spreads (see Rosenberg and Maurer 2008) have also been cited as
reasons for not finding the predictive power of yield spreads.
The empirical validity of predictive power of the yield spread is therefore still an
unsettled debate. Much of the work has focussed on developed countries, whereas its
empirical verification has received least attention in developing countries like India.
This is partly because, till recent past, many developing countries did not form a suit-
able test case owing to their regulated interest rate regimes which did not allow the
emergence of market determined yield curve. In India, after the emergence of yield
curve, attempt has been made to establish the predictive power of yield spread. Kana-
gasabapathyandGoyal(2002)arguethatIndianyieldspreadpost1996issuccessfulin
predicting future economic activity. Using probit estimation procedure, Bhaduri and
Saraogi (2010) have shown that yield spread in India is able to time stock markets.
Notwithstandingconsiderableresearcheffortsonanalyzingthepredictiveabilityof
theyieldspreadforeconomicactivity,fewquestionsstillremainopentodebate.Some
of such questions are flagged as follows: Are there other reasons besides government
regulations, asymmetric monetary policy effect and time varying term premium in
spreads that diminish the predictive power of yield spreads? Does the informational
content depend on time patterns? In other words, is there any variability in the predic-
tions, when time periods of short-run, medium-run, and long-run are considered? Are
their switching signs of the coefficients and does their significance change, when one
regresseslaggedtermspreadsonoutputintheshort-,medium-,andlonger-timescale?
Empirical analysts have paid scant attention toward the role of time scales in estimat-
ing the predictive ability of term spreads. Standard econometric methods like vector
error correction and co-integration models generally employed in economics have
been designed to deal with just two time frames: the short and the long run. However,
recent developments in statistical techniques have made it possible to think of more
than two time scales. In this direction, studies like Zagaglia (2006), and Tabak and
Feitosa (2009, 2010) have used the wavelets to test the multi-scale predictive ability
of yield spreads.
Inthispaper,weemploywaveletmethodologytotesttheabilityoftheyieldspreads
to predict economic growth in India. The present study improvises upon the previous
studies in several respects. Previous studies have used discrete wavelet transform
(DWT) to decompose the yield spread and output; we use maximal overlap discrete
transform (MODWT) to decompose them into different time scales. MODWT comes
with extra benefits which are discussed in Sect. 4. Secondly, following Tkacz (2004),
wetestthepredictiveabilityofyieldspreadsconstructedatshorterend,longerend,and
policy relevant areas of yield curve. The results stemming the aggregate (unfiltered)
A. B. Dar et al.
in the study. In Sect. 4, the results of the analysis are discussed, and finally, Sect. 5
gathers the main conclusions.
2 Motivation and methodology
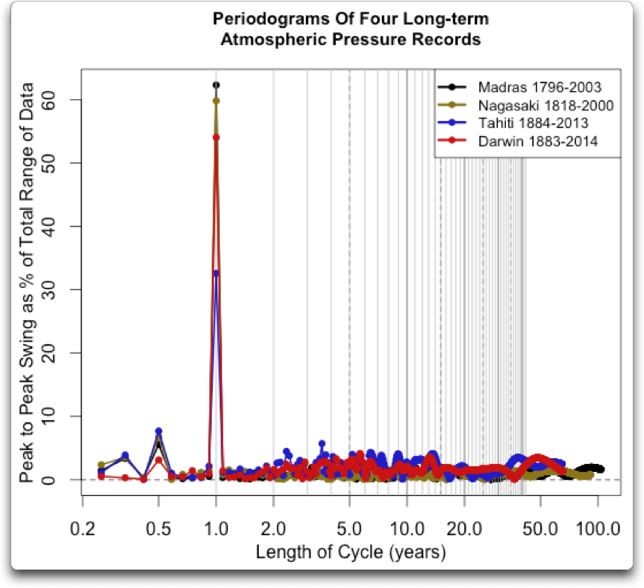
Until recent past, the predictive ability of yield spread has been estimated using con-
ventionaltimedomainapproach,andunderlyingtimescaleshavealmostbeenignored.
Nevertheless,itisclearthatthestrategiesusedbybothemployersandemployeesdiffer
by time scale; that is, employers, for example, may adjust hours worked in the short-
run, to redesigning the plant in the longer-run, to moving manufacturing abroad in the
longest run (see Gallegati et al. 2011). This leads to heterogeneous frequency con-
tents in the production time series. Similarly, monetary policy, which drives the most
predictive power of the yield spread, is also a heterogeneous process. Central banks
have different objectives in the short and long run, and they operate at different time
scales separately (see Aguiar-Conraria et al. 2008). This operation of central banks
at different time scales also leads to heterogeneous frequency contents in the yield
spreads. Essentially, the actual time series of both yield spread and output growth are
generated by the combination of different frequencies or time scales. Therefore, when
the relationship between output and yield spread is modeled in time domain frame-
work, it leads to time or frequency aggregation bias, with true relationships remaining
veiled under frequencies or time scales.1
In the existing literature, it has been a common practice to utilize Fourier analysis
to uncover relations at different frequencies. In spite of its utility, under the Fourier
transform, the time information of a time series is completely lost. Because of this
loss of information, it is hard to distinguish transient relations or to identify structural
changes. Moreover, these techniques are only appropriate for time series with stable
statistical properties, i.e. stationary time series. Unfortunately, typical economic time
series are noisy, complex, and strongly non-stationary. To overcome the problems of
analyzing non-stationary data, Gabor (1946) introduced the short-time Fourier trans-
form (STFT). The basic idea of this transform is to break a time series into smaller
sub-samples and then apply the Fourier transform to each sub-sample. However, this
approachhasbeenfoundinefficientbecausethefrequencyresolutionisthesameacross
alldifferentfrequencies.AnalternativetotheSTFTforanalysisofnon-stationarysig-
nals is represented by the wavelet transform.
Wavelet analysis shares several features in common with Fourier analysis but has
the advantage of capturing features in the underlying series that vary across both time
and frequency. Wavelets are mathematical functions that decompose data into differ-
ent frequency components, after which each component is studied with a resolution
matched to its scale. These functions are generated by the dyadic dilations and inte-
ger shifts a single function called a mother wavelet. The key feature of wavelets is
the time-frequency localization. It means that most of the energy of the wavelet is
restricted to a finite time interval and its Fourier transform is band limited. When
1Since time and frequency are inversely related, higher time scales represent lower frequencies of time
series and vice-versa.
123
Page 7
The predictive power of yield spread
compared to STFT, the advantage of the time-frequency localization is that wavelet
analysis varies the time-frequency aspect ratio, producing good time resolution and
poor frequency resolution at high frequencies, and a good frequency resolution and
poor time resolution at low frequencies. This approach is logical when the signal on
hand has high-frequency components for short durations and low-frequency compo-
nentsforlongdurations.Fortunately,thesignalsthatareencounteredinmosteconomic
applications are often of this type.
The application of wavelet theory in modeling and analyzing economic data is a
recent phenomena and its applications in economics began in the late nineties by the
contribution of Ramsay and his collaborators (See for example Ramsay and Lampart
1998a,b). Since then wavelets have been used in many areas of economics and finance
(see Percival and Walden 2000; Gençay et al. 2002; Ramsey 2002; Crowley 2007),