THE MOMENTUM TRADING STRATEGY (Finance)
Post on: 16 Июнь, 2015 No Comment
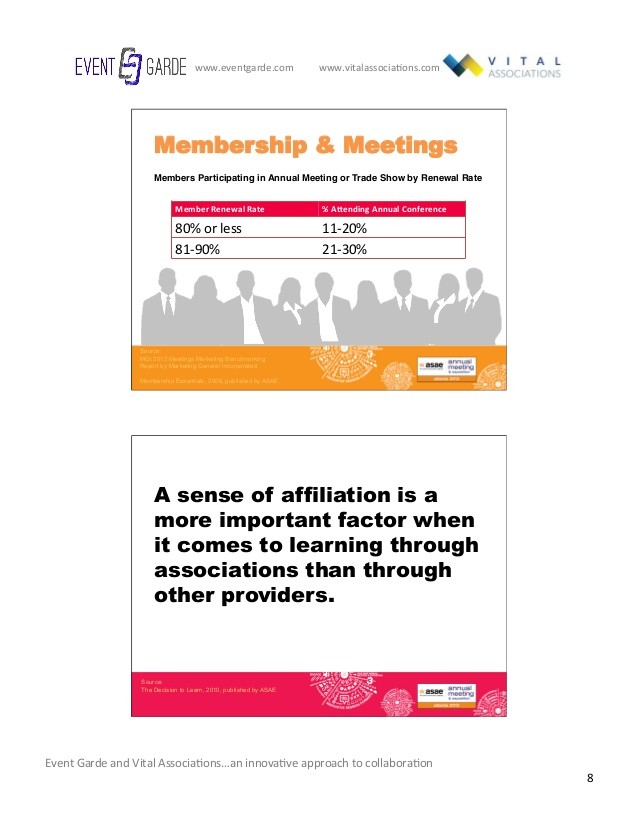
Abstract
A strategy that buys past winners and simultaneously sells past losers based on stock performance in the past 3 to 12 months is profitable in the U.S. and the European markets. This survey paper reviews the literature on the momentum strategy and the possible explanations on the momentum profitability.
Keywords: past winners; past losers; momentum strategy; individual momentum; industrial momentum; international momentum; underreaction; over-reaction; overconfidence; self-attribution; valuation uncertainty; conservatism; representative heuristic; gradual information diffusion
Introduction
Trend is your friend is a very popular saying in Wall Street since the inception of stock markets. However, whether this momentum trading strategy that is based on buying past winners and selling past losers is really profitable was controversial until recently. Jegadeesh and Titman (1993) were the first to comprehensively test the profitability of the momentum trading strategy based on the past 3-to 12-month performance. They document that momentum strategies implemented in the U.S. market from 1965 to 1989 generated a positive profit of about one percent per month over 3-to 12-month holding periods. In their recent follow-up study, Jegadeesh and Titman (2001) find that momentum strategies continued to be profitable after 1990 with past winners outperforming past losers by about the same magnitude as in the earlier period.
Rouwenhorst (1998) studied individual stock momentum with a sample of stocks listed on 12 European exchanges during the period from 1978 to 1995. The results demonstrate that momentum profits of about one percent per month are not limited to a particular market, but instead they are present in all 12 markets in the sample. Rou-wenhorst (1999) also finds that momentum strategies are profitable although not to the same degree in 20 emerging markets. Chui et al. (2002) examine the profitability of momentum strategies in eight different Asian countries: Hong Kong, Indonesia, Japan, Korea, Malaysia, Singapore, Taiwan, and Thailand. Their evidence indicates that the momentum effect is present in all of the Asian countries except Korea and Indonesia but it is generally weak and is statistically significant only for Hong Kong, Malaysia, Singapore, and Thailand for the pre-crisis period. Interestingly, they find that the Common Law/Civil Law distinction provides an indicator of whether or not a market exhibited a momentum effect prior to the financial crisis. Asness et al. (1996), Chan et al. (2000), and Richards (1997) document that momentum strategies are profitable when implemented on stock market indices.
Recently Moskowitz and Grinblatt (1999) find that industry momentum strategies, which advocate buying stocks from past winning industries and selling stocks from past losing industries, appear to be highly profitable. This industry momentum accounts for much of the profitability of individual stock momentum strategies in the United States. Once returns are adjusted for industry effects, momentum profits from individual equities are significantly weaker, and for the most part are statistically insignificant. However, Grundy and Martin (2001) have a different view on the contribution of industries to individual momentum profits. They argue that a one-month interval between the ranking period and the holding period has a pivotal role in the conclusion that industry momentum strategies are profitable. Industry momentum strategies are significantly profitable only when the ranking period is contiguous to the holding period as documented by Mosko-witz and Grinblatt (1999). However, given a one-month interval between the two periods, industry momentum strategies cannot earn significant profits. Grundy and Martin (2001) conclude that industry effects are not the primary cause of the individual momentum profitability. Liu and Wei (2004) document that industries in 12 European markets, like their counterparts in the U.S. market, also explain the profitability of individual momentum strategies. Specifically, past winner industries outperform past loser industries by more than one percent per month. However, unlike their counterparts in the U.S. market, industries cannot solely explain the profitability of individual momentum strategies in 12 European markets. In addition, industry momentum strategies can still earn significant profits even with a one-month interval between the formation and holding periods.
The Implementation of Momentum Strategies
To show how to implement a momentum strategy, we use a momentum strategy that is based on the past six-month performance with a six-month holding period an illustration. Specifically, to form momentum portfolios, at the end of each month all securities in each of the samples are ranked in ascending order based on the past six-month cumulative returns with dividends. The securities in the bottom 10 percent (or 20 percent or 30 percent) are assigned to the loser (denoted as L) portfolio, while those in the top 10 percent (or 20 percent or 30 percent) are assigned to the winner (denoted as W) portfolio. These portfolios are value-weighted using the market capitalization of the security at the end of the ranking month as the weight. Each of these portfolios is held for six months.
To reduce the effect of nonsynchronous trading and the bid-ask bounce, Jegadeesh and Titman (1993) suggest that we measure returns on these portfolios one month after the ranking takes place. If a security has any missing returns during the holding period, we replace them with the corresponding value-weighted market returns. If the returns on the security are no longer available, we rebalance the portfolio in the month the security is deleted from our database. Excess returns on a security are calculated as the returns on that security minus the risk-free rate, which we assume is equal to the one-month government short-term rate, such as the U.S. Treasury bill rate.
To increase the power of our tests, we construct overlapping portfolios. The winner (loser) portfolio is an overlapping portfolio that consists of the W (L) portfolios in the previous six months. The returns on the winner (loser) portfolios are the simple average of the returns on the six W (L) portfolios. For instance, the January return on the winner portfolio is the simple average of the January returns on the W portfolios that are constructed from June to November in the previous year. The momentum portfolio we examine is the zero-cost, winner-minus-loser portfolio.
Explanations of Momentum Profits
Jegadeesh and Titman (2001) discuss three potential explanations for the profitability of momentum strategies and examine the performance of momentum portfolios over longer horizons in order to differentiate between these hypotheses. The three explanations include: (1) stock prices underreact to information, (2) there is a delayed overreaction to information, and (3) the profits are generated from cross-sectional differences in expected returns.
The first two explanations are consistent with some recent behavioral models. For example, the underreaction explanation is consistent with the Barberis, Shleifer, and Vishny (1998) model where a conservatism bias can lead investors to underreact or underweight new information. In the case with a pure conservatism bias, once the information is fully incorporated in prices, there is no predictability in stock returns. In this case, the expected post-holding period returns are zero.
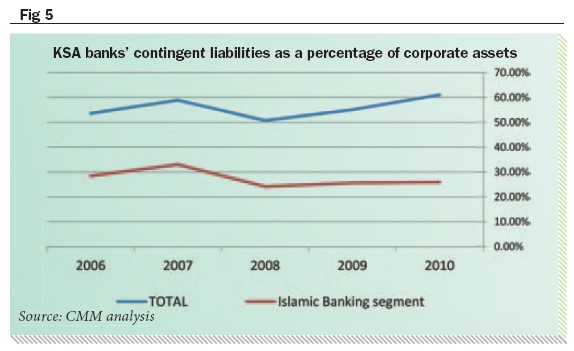
There are a number of behavioral models that are consistent with a delayed overreaction. Bar-beris et al. (1998) also discuss this possibility and describe what they call the representative heuristic, which suggests that investors may overly extrapolate a firms past extraordinary earning growths into the future, and hence overreact to positive (or negative) information that is preceded by positive (or negative) information. In addition, Daniel et al. (1998) argue that delayed overreaction can arise because of self-attribution (or cognitive) bias. That is, investors tend to become more overconfident when their stock picks become winners and take more aggressive positions that push up the prices of winners above their fundamental values. Finally, Hong and Stein (1999) propose a model with two groups of investors: informed investors and technical traders, who do not fully take into account the actions of each other. As a result, information is incorporated slowly into stock prices, providing a potential profit opportunity for technical traders. These traders, however, tend to push prices of past winners above their fundamental values. In each of these behavioral models, prices tend to eventually overreact to information and then reverse when prices eventually revert to their fundamentals. All these behavioral models predict the expected post-holding period returns to be negative.
The third explanation is consistent with an efficient market where stocks have different expected rates of return because of different risk exposures. In particular, Conrad and Kaul (1998) emphasize that there would be some evidence of momentum even if there were no time-series variation in expected returns since stocks with high-(low) expected returns would be expected to have the highest (lowest) returns in adjacent periods. This explanation suggests that the profits from a momentum strategy should be the same in any post-ranking period.
To test these competing hypotheses, we normally examine the post-holding period returns of momentum portfolios beyond the first year after formation, typically up to five years. The empirical evidence from the U.S. (Jegadeesh and Titman, 2001) and Asian markets (Chui et al. 2002) appears to support the delayed overreaction explanation. That is, the returns on the momentum portfolio eventually reverse to negative 2-5 years after formation. In addition, Fama and French (1996) find that the Fama-French (1993) three factors cannot explain the momentum profits in the United States.
Momentum Profits and Firm Characteristics
Firm characteristics such as topic-to-market ratios, market capitalization, and turnover have shown to have the ability to predict the cross section of expected stock returns in the United States. Behavioral models also predict that momentum profits are related to firm characteristics.
The overconfidence model by Daniel, Hirshleifer, and Subrahmanyam (1998) suggests that momentum profits arise because investors are overconfidence. Daniel and Titman (1999) argue that overconfidence is likely to influence the perception of investors relatively more, when they analyze fairly vague and subjective information, and use topic-to-market ratios as a proxy for information vagueness. Consistent with their hypothesis, they find that momentum profits are negatively related to the firms topic-to-market ratio in the U.S. market. Chui et al. (2002) also find similar results for Asian markets.
Trading volume or turnover could also proxy for information vagueness. As suggested by asym- metric information models (see for example, Blume et al. 1994), trading volume reflects investors disagreement on a stocks intrinsic value. The more vague the information used to value the firm, the more disagreement among the investors, and hence, the greater the trading volume. Therefore, the momentum effect should be stronger for firms with high trading volume or turnover. and Swaminathan (2000) find that momentum profits are indeed higher for firms with high turnover ratios in the U.S. market. Chui et al. (2002) also find similar results for Asian markets.
In contrast, Hong and Stein (1999) predict that stocks with slow information diffusion should exhibit stronger momentum. Hong et al. (2000) provide tests that support this prediction. In particular, except for the very smallest decile stocks, the profitability of momentum investment strategies declines sharply with firm size. Hong et al. (2000) also look at momentum profits and analyst coverage and find that holding size fixed-momentum strategies work better for stock with low analyst coverage. In addition, they find that the effect of analyst coverage is greater for stocks that are past losers than for stocks that are past winners. They conclude that their findings are consistent with the gradual information diffusion model of Hong and Stein (1999).