ShortSelling Uptick Rule and Market Quality Evidence from HighFrequency Data on Hong Kong Stock
Post on: 16 Март, 2015 No Comment
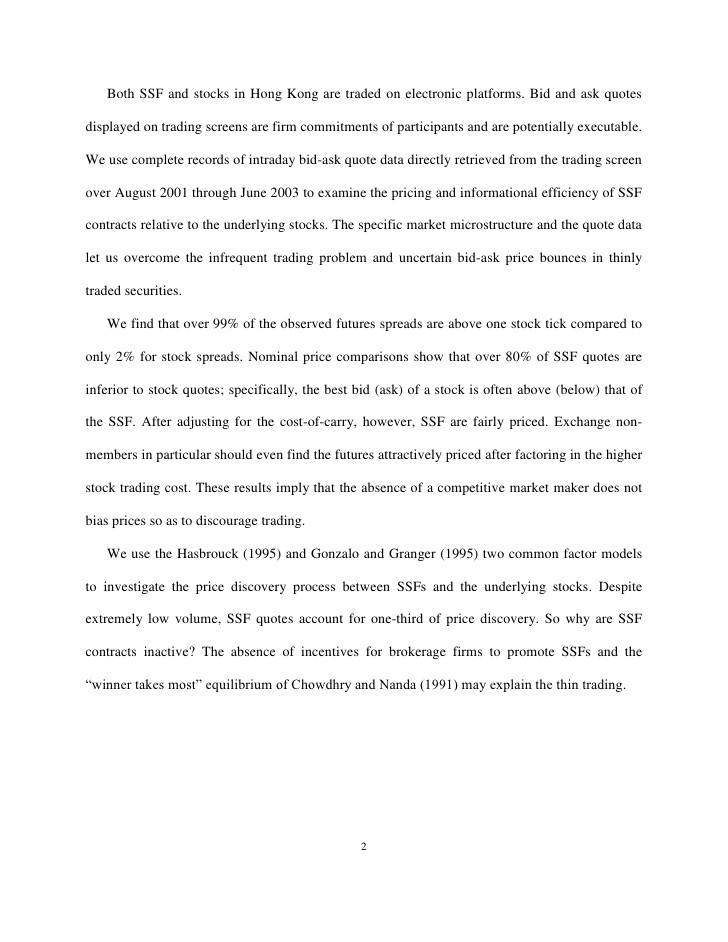
Page 1
ssrn.com/abstract=1710924
Short-Selling, Uptick Rule, and Market Quality: Evidence from
High-Frequency Data on Hong Kong Stock Exchange∗
Pengjie GaoJia HaoIvalina Kalcheva Tongshu Ma†
This draft: March 1, 2011
First draft: November 1, 2006
Comments welcome
Abstract
Much empirical research has been conducted concerning the effect of short-selling on market
quality and volatility. However, the evidence is inconclusive and still a matter of debate. Using
intraday data in a pure order-driven market we show that allowing for short-selling decreases
the adverse selection costs for less-visible firms, firms with less analyst coverage, larger adverse-
selection cost component of the bid-ask spread, low price per share, and high relative tick size
(given the same market capitalization). Allowing for short-selling also decreases (increases)
intraday volatility for less- (more-) visible stocks. In addition we document that with the uptick
rule in place (not in place) there is not statistically significant difference in liquidity (intraday
volatility) between stocks that are allowed for short-selling and those that are not.
Keywords: short-selling, market quality, liquidity, volatility, uptick rule
JEL classification codes: G1, G2
∗We are grateful to staff members of Research Department, and in particular Alexandra Young, at the Hong
Kong Security and Futures Commission (SFC) and Karen Lam of the Hong Kong Stock Exchange and Clearing
House Incorporated (HKSE) for numerous discussions about short-sale regulations in Hong Kong. We thank
George Gao, Karen Lam, David Xue, Alexandra Young, and Xueping Wu for helping us with some of the data used
in this study. Seminar participants from Northwestern University provided useful feedback. We benefited from
discussions with Torben Andersen, Hank Bessembinder, Kent Daniel, Ravi Jagannathan, Mitchell Peterson, Todd
Pulvino, Ernst Schaumburg, Annette Vissing-Jorgensen, Wallace Mok, Xueping Wu and Sudip Datta. Morgan
Stanley Market Microstructure Fund provides partial funding for this research project. Jon Ross from the David
Eccles Business School and Patricia Li´ ebana from the Kellogg School of Management provided indispensable
computational assistance in this project. The views herein are solely those of the authors, and not those of
SFC, HKSE, Morgan Stanley and Company, or any other person or entity. We are responsible for any remaining
omissions and errors. Earlier versions of this manuscript were titled “Does Removing the Short-sale Constraint
Improve Quality? Evidence from Hong Kong”
†The authors are from University of Notre Dame, Wayne State University, University of Arizona and
Binghamton University, respectively.The emails for the authors are pgao@nd.edu, jia.hao@wayne.edu,
kalcheva@arizona.edu, and tma@binghamton.edu, respectively. Corresponding author: Ivalina Kalcheva, Address:
Eller College of Management, McClelland Hall 315L, 1130 E. Helen St. P.O. Box 210108, Tucson, Arizona 85721-
0108, Tel: + 1-520-621-0747, Fax: + 1-520-621-1261, E-mail: kalcheva@arizona.edu.
Page 2
ssrn.com/abstract=1710924
Short-Selling, Uptick Rule, and Market Quality: Evidence from
High-Frequency Data on Hong Kong Stock Exchange
Abstract
Much empirical research has been conducted concerning the effect of short-selling on market
quality and volatility. However, the evidence is inconclusive and still a matter of debate. Using
intraday data in a pure order-driven market we show that allowing for short-selling decreases
the adverse selection costs for less-visible firms, firms with less analyst coverage, larger adverse-
selection cost component of the bid-ask spread, low price per share, and high relative tick size
(given the same market capitalization). Allowing for short-selling also decreases (increases)
intraday volatility for less- (more-) visible stocks. In addition we document that with the uptick
rule in place (not in place) there is not statistically significant difference in liquidity (intraday
volatility) between stocks that are allowed for short-selling and those that are not.
Page 3
I. Introduction
How do short-sale constraints matter for the functioning of the financial markets? What is the
role of the “uptick rule,” which generally prevents securities from being sold short at a declining
price? This has been a topic of debate among market practitioners, regulators, and financial
economists at least since the 1930s (Jones, 2008). Recently, the interest in short-selling and price
tests has increased dramatically in light of the 2007–2009 financial crisis, which caused regulators
across the globe to implement some sort of short-sale constraint (Beber and Pagano, 2010). Our
paper revisits this debate by using high-frequency data in a pure order-diven market and taking
advantage of the fact that on the Hong Kong Stock Exchange (HKSE hereinafter) short-selling
is allowed1by regulators for stocks that meet certain requirements, and more importantly, for
the stocks that are allowed to be sold short there was a period when the uptick rule was in
place and when it was not, creating a suitable natural experimental environment. The HKSE is
one of the largest and most liquid equity markets in the world where short-selling is practiced
(Bris, Goetzmann, and Zhu, 2007). The HKSE achieves a high level of market transparency
and operates as a pure order-driven market similar to the prototype public limit order book
envisioned in Glosten (1994). More details on the trading mechanism can be found in Appendix
On the HKSE before January 1994, short-selling was prohibited. After January 1994, only
some stocks were allowed for short-selling, while others were not. The list of designated securities
for short-selling was revised on a quarterly basis after 1996 — stocks which met the criteria of
“eligible stocks” were added into the short-sale list, while those no longer eligible were removed
from the list. In this case, we have a complete history of whether each individual stock is
allowed for short-selling on each day. The fact that stocks become eligible for short-selling on
different dates is an advantage as it implies a reduced likelihood that comparisons will be affected
by contemporaneous changes in market-wide factors affecting the inferences of the relationship
between the variables we study (Bessembinder, 2000). The details of the regulation on the
1According to the regulations of the SFC of Hong Kong, breach of short-sale constraint is a criminal offense
punishable by fines and imprisonment according to the security laws in Hong Kong. The level of fines and
imprisonment were revised (from a maximum of $10,000 and 6 months) to HK$100,000 and 2 years imprisonment
with the introduction of the Securities (Amendment) Ordinance 2000 on July 3, 2000.
Page 4
selection of stocks eligible for short-selling is provided in Appendix A. Generally, stocks eligible
for short-selling on the HKSE are to a great extent large and liquid stocks.
In the pilot program, the HKSE also instituted the uptick rule, which mandated that a
short-sale could not be made below the best current ask price. The uptick rule was abolished in
March 1996 and reinstated on September 7, 1998, due to changes in market conditions during
the East Asian financial crisis. Hence, from March 1996 to April 1998, short-sales were operated
without the uptick rule. At other times in our sample, short-sales were operated with the uptick
rule.
Our study employs high-frequency transaction data from a pure order-driven market to
examine the relationship between short-selling, the uptick rule, and market quality by looking
at an array of measures at the stock level: spreads, order imbalance, relative depth of the
limit order book, and intraday return volatility. Using intraday data allows us to decompose
the total spread into its order-processing and adverse-selection components which provides an
opportunity for a direct test of the Diamond and Verrecchia (1987) model as explained in the
next section. We adopt the quasi-experiment framework (using the terminology of Meyer, 1995)
and apply difference-in-difference (DID hereinafter) estimation as our main empirical tool. As
the regulation changes are not random and the choice of stocks to be allowed for short-selling is
not entirely exogenous, using control sample and control covariates in the empirical methodology
is critical. Such a methodology allows us to make more reliable inferences.
Using the DID approach, our empirical results suggest that short-sale constraints affect
market quality. The results suggest that the impact of short-sale constraints on market quality
measures is not homogeneous across stocks and depends on firm’s visibility. Less-visible firms are
defined as those with less analyst coverage, larger adverse-selection cost component of the bid-ask
spread, low price per share, and high-relative tick size (given the same market capitalization).
When the uptick rule is not in place, allowing for short-selling generally reduces transaction
costs. More importantly, most of the reduction is associated with the reduction of the adverse
selection component of the bid-ask spread and concentrates in less-visible stocks. In fact, with
the uptick rule in place, the difference in transaction costs between stocks that are allowed to
be sold short and those that are not, is statistically and economically insignificant. With the
Page 5
uptick rule in place, however, allowing for short-selling decreases intraday volatility for less-
visible stocks but increases the intraday volatility for more-visible firms. With the uptick rule
not in place, we do not find statistically significant difference in intraday volatility between
stocks that are allowed for short-selling and those that are not.
We take advantage of two distinctive institutional differences between HKSE and the U.S.
exchanges to gauge the visibility level of a firm. First, unlike U.S. stock exchanges, which have a
uniform tick size across most of the stocks, the tick size schedule on the HKSE is a step function
of the underlying security’s price. For example, for stocks traded between HK$0.01 and HK$0.25,
the tick size is HK$0.001; while for the stocks traded above HK$200.00 and below HK$1000.00,
the tick size is HK$1. The complete schedule of tick sizes is illustrated in Appendix C. The
schedule is valid from October 1996 to October 2005.2Second, the majority of the securities
on the HKSE are low-priced stocks, but it should be noted that they are not “penny stocks”
given the U.S. standards. For example, Angel (1997) notes that the median U.S. stock sells for
about $40, while a typical Hong Kong share is about $2. More importantly, on the HKSE the
low-priced stocks are spread rather evenly across large and small firms. According to the model
presented in Angel (1997), a highly visible firm will have a lower optimal relative tick size than
a less visible firm. Given the step tick function on the HKSE, lower-priced securities according
to Angel (1997) will be less-visible firms and higher-priced securities will be more visible.
Therefore, in our analysis we look at three different price groups — “low,” “medium,” and
“high” — as a proxy for a firm’s visibility in order to investigate whether the relationship
between short-selling, the uptick rule, and market quality is not homogeneous across stocks.
The reader should keep in mind that the “Low-Price” Group does not include “penny stocks”
given the U.S. standards. By sorting on price per share, our Low-Price Group includes firms
that are better characterized as firms with less analyst coverage (public information is more
2Effective from November 1, 2005, the HKSE implemented Phase 1 of the proposal to reduce minimum trading
spreads for shares with a price above HK$30, so the minimum tick size schedule is no longer valid. Specifically,
for stocks priced above $30 and below $50, the minimum tick size is $0.05; above $50 and below $100.00, the
minimum tick size is $0.100; above $100 and below $200, the minimum tick size is $0.100; above $200 and below
$500, the minimum tick size is $0.200; above $500 and below $1,000, the minimum tick size is $0.500; above
$1,000 and below $2,000, the minimum tick size is $1.000; above $2,000 and below $5,000, the minimum tick
size is $2.000; and above $5,000 and below $9,995, the minimum tick size is $5.000 (all units are in HK dollars).
www.hkex.com.hk/rule/exrule/sch-
2 eng.doc. During our sample period, there is no reduction of minimum tick size for the sample of traded stocks
we consider.
Page 6
limited), larger adverse-selection cost component of the bid-ask spread, low price per share, and
high-relative tick size (given the same market capitalization). Our evidence suggests that the
direction and the strength of the effect of short-sale constraints on market quality measures is
dependent upon a firm’s visibility.
We provide evidence on the effect of short-selling constraints in an order-driven market.
This is important because today more than half of the world’s stock exchanges are order-driven
(Ro¸ su (2010)), with no designated market makers (e.g. Euronext, Helsinki, Hong Kong, Tokyo,
Toronto), while in many hybrid markets designated market makers have to compete with a
limit order book (NYSE, Nasdaq, London). One could imagine that the effects of short-selling
constraints will be different across different market structures — e.g. order-driven vs. quote-
driven. One reason, for example, could be that in a quote-driven market a market maker could
still be allowed to short-sell under a short-sale ban environment (as in the recent U.S. shorting
ban) while this is not the case in a pure order-driven market. Despite the growth of order-driven
markets around the world, we are not aware of a theoretical study that compares and contrasts
the effects of short-selling constraints on stock-level liquidity, volatility, and price informational
efficiency across the two market structures, order-driven and quote-driven. This is even more
important in light of the recent spree of short-selling bans around the world in response to the
2007-2009 global financial crisis (Beber and Pagano, 2010).
The rest of the paper is organized as follows.The next section reviews the literature
and develops our hypotheses. Section III explains our empirical methodology in more detail,
including the data used and the sample selection process, and it introduces the proxies for market
quality. Section IV presents the results, and Section V concludes.
II. Literature Review and Hypothesis Development
The short-selling restrictions that regulators have imposed, in and outside U.S. for the most
part comprise but a single event, and even more importantly, most shorting bans are triggered
as a response to deteriorating market conditions. For example, Jones (2008) studies the short-
sale regulation changes in the 1930s that were a response by regulators to worsened market
conditions. Boehmer, Jones, and Zhang (2009) study the SEC emergency order as of September
Page 7
2008 to temporarily ban most short-sales in approximately 1,000 financial stocks for the short
period of time from 9/19/2008 to 10/8/2008, and Boehmer, Jones, and Zhang (2008) study the
repeal of the uptick rule by the SEC on July 6, 2007. Diether, Lee, and Werner (2009) and
Alexander and Peterson (2008) invoke regulation SHO3to also study short-sale price tests. In
contrast to these studies, the current study does not fall into the group of “before-vs.-after”
studies (to use the terminology in Bessembinder, 2000). One important feature of our sample
is that, at each point in time, there are stocks that are allowed for short-selling as well as those
that are not, and the list of stocks eligible for short-selling is updated quarterly. Hence, we can
also control for market-wide changes that are caused by events unrelated to short-sale regulation
changes. Further, for stocks included in the short-selling list there is a period of time when the
uptick rule is in place and a period when it is not in place. We perform firm-level cross-sectional
analysis by comparing stocks affected by the constraints4and stocks that are not at the same
time given different levels of firm visibility using high-frequency data in a pure order-driven
market.
Boehmer, Jones, and Zhang (2009) show that the financial stocks subject to the short-sale
ban suffered a severe degradation in market quality, as measured by spreads, price impacts, and
intraday volatility. Outside the U.S. the cross-country study by Charoenrook and Daouk (2008)
focuses on the market-wide aggregate impact of relaxing the short-sale constraints rather than
the market quality at the individual stock level.5They find that when short-selling is possible,
there is greater aggregate market liquidity. Beber and Pagano (2010) study short-selling bans
around the world in response to the 2007–2009 crisis, using daily data. Chang, Cheng, and
Yu (2007) use the HKSE’s short-sale regulation changes, as in the current study. Our study,
however, differs from theirs in terms of the research question, the empirical design, and the
3It is worth noting that Regulation SHO also contains additional regulations on requiring locating stocks and
more frequent disclosure of short interests. Therefore, strictly speaking, the empirical tests of Regulation SHO
are a joint-test of all of these regulation changes.
4We note that, on September 8, 1995, the SEHK launched its Traded Stock Options Market. In our sample,
we cannot identify which firms have traded options. However, the fact that the sample contains firms with traded
options strengthens our results. Figlewski and Webb (1993) and Danielsen and Sorescu (2001) have argued that
the introduction of traded options represents an economically important relaxation of short-sale constraint. Even
if the whole sample consists of firms with traded options, given that to a large extent our sample comprises of
large and liquid stocks, this will strengthen our result.
5Bris, Goetzmann, and Zhu (2007) is also a cross-country study that focuses on market-wide aggregate impact
of relaxation of short-sale constraints, but they study primarily the effects on price informational efficiency.
Page 8
frequency of data. They focus on short-sale constraints’ effect on stock valuation and volatility
using daily prices and returns data. In contrast, we use high-frequency transaction data. The
high-frequency data allow us to measure intraday spreads, order imbalance, relative depth of the
limit order book, and intraday return volatility. Moreover, we can decompose the intraday total
spread into its order-processing and adverse-selection components to directly test Diamond and
Verrecchia (1987) theoretical model as explained later in this section. Chen and Rhee (2010)
also use the HKSE data, but their focus is on price adjustment to new information.
Boehmer, Jones, and Zhang (2008) show that the repeal of the uptick rule causes market
liquidity to worsen slightly, although they find no evidence that repeal of the uptick rule
contributed to the bout of volatility experienced by U.S. stocks in late July and early August
2007. Alexander and Peterson (1999) examine the impact of the uptick rule on short-sell orders
sent to the NYSE. They find that the execution quality of short-sell orders is adversely affected
by the uptick rule, even when stocks are trading in advancing markets. Alexander and Peterson
(2002) consider how smaller tick size is related to short-sale order executions by looking at the
1997 reduction in tick size from 1/8th to 1/16th. They argue that the move to decimalization
in conjunction with the uptick rule lessens the impact of the uptick rule for short market-
orders. However, smaller tick size hurts the short at-the-quote limit orders when the spread is
larger than the minimum tick size. They also suggest that short-sellers may place market-orders
more frequently than at-the-quote limit orders, but also cancel their orders more frequently and
quickly. Jones (2008) studied the regulation changes in the 1930s U.S. equity market using daily
data. He found that requiring brokers to secure written authorization before lending shares to
a customer decreased liquidity, consistent with Diamond and Verrecchia (1987). However, the
uptick rule and “strict uptick rule” seemed to increase rather than decrease market liquidity
because these rules essentially force short-sellers to use limit orders rather than market orders,
thus providing liquidity. Diether, Lee, and Werner (2009) use the recent Regulation SHO to
evaluate how market liquidity is affected by the suspension of the uptick rule and the conversion
to price-test on the NYSE, as well as the suspension of the bid-price test and the conversion to
price-test on NASDAQ. They found that Regulation SHO reduces the depth at the offer side
relative to the bid side, reduces buy-order imbalance, and increases quoted spreads for the NYSE
Page 9
sample but not much for the NASDAQ sample. Alexander and Peterson (2008) also examine
the recent Regulation SHO on market liquidity and their results are consistent with those in
Diether, Lee, and Werner (2009). Diether, Lee, and Werner (2009) and Alexander and Peterson
(2008) find that the pilot program suspending the uptick rule widens spreads slightly.
On the theoretical front, to our knowledge, Diamond and Verrecchia (1987) is the only study
that explicitly models the effect of short-sale constraints on stock-level liquidity. The basic
structure of the Diamond and Verrecchia (1987) model is based on Glosten and Milgrom (1985):
market makers are risk-neutral, earn zero expected profits from each trade, and face no inventory
costs or constraints. The latter assumption means that they are willing to keep selling or buying
the asset at fair prices. Short-prohibition in their model excludes both informed traders and
uninformed traders from short-selling, and hence it leaves the probability that a sale comes from
an informed trader the same whether short-selling is prohibited or not. Thus, short-prohibition
does not make sell orders more informative. Short-prohibition does not change anything on the
buy side in their model as well.
Generally, Diamond and Verrecchia’s (1987) common-priors rational-expectations model
predicts that short-sale constraints impede the flow of private information. Short-sale prohibition
increases the average bid-ask spread, compared with the case in which short-selling is costless,
because the speed of adjustment of prices to values that imply a small bid-ask spread is reduced.
In other words, the short-sale prohibition reduces the speed at which the price converges to the
true liquidation value, and therefore it reduces the speed at which the bid-ask spread narrows
over time.
The size of the spread and the price of the stock are determined by supply and demand. The
increase in the bid-ask spread, when short-selling is prohibited, is due to a lower supply of stocks
for sale because of some investors wanting to sell but not owning the stock. This fact manifests
itself as a no-trade period in the model, when no transaction takes place during a given trading
interval. Short-selling constraints lead to an increased incidence of no-trade outcomes, which is
one of the main points of Diamond and Verrecchia (1987). The no-trade outcome is important
in that the frequency of no-trades is informative to the market maker in the sense that probably
informed traders have not entered the market yet. A no-trade period is a sign of the release of
Page 10
private information.
The Diamond and Verrecchia (1987) model is a rational-expectations model that predicts
the effects of short-selling restriction on liquidity in a quote-driven market. Interestingly, most
empirical studies that focus on price effects of short-selling constraints provide evidence mostly
consistent with differences-in-beliefs6models, as in Miller (1977).We are not aware of a
theoretical study that predicts the effects of liquidity in a differences-in-beliefs setting in an
order-driven market per se.
Given our analysis above and the order-driven environment in the HKSE, the effect of short-
sale constraints on liquidity and volatility is ultimately an empirical question. For example,
Diamond and Verrechia (1987) ignore the depth dimension of liquidity as they assume one unit
size for all trades; however, one could imagine that trade size (or depth of the limit order) would
matter. In any case, using the intuition presented in Diamond and Verrecchia (1987), our three
main hypotheses are as listed below.
H1:When a stock is initially allowed for short-sale, on average, the bid-ask spreads, and
especially the adverse selection component of the bid-ask spreads, will drop.
Note that in the world of Diamond and Verrecchia, the market makers incur no inventory
cost. Therefore, the bid-ask spread is really the asymmetric information component rather than
the overall bid-ask spread. In the empirical analysis, we will decompose the total spread into
adverse-selection and order-processing components.
H2: When there is no uptick rule, allowing a stock for short-sale will reduce bid-ask spreads,
and particularly the adverse-selection components of the bid-ask spreads, more than when the
uptick rule is present.
We speculate that firms with increased incidence of no-trade outcomes when short-selling is
prohibited will be the less-visible firms as measured by: firms with less analyst coverage, larger
adverse-selection cost component of the bid-ask spread, low price per share, and high-relative
tick size (given the same market capitalization). Even more importantly, these are stocks that
ultimately have the most to gain from short-selling in terms of liquidity.
H3: Among the stocks initially allowed for short-sale, on average the bid-ask spreads will
6For example, see Figlewski (1981); Boehme, Danielsen, and Sorescu (2006); Danielsen and Sorescu (2001);
and Jones and Lamont (2002), among others.
Page 11
drop more significantly for stocks with less public information (or more private information) —
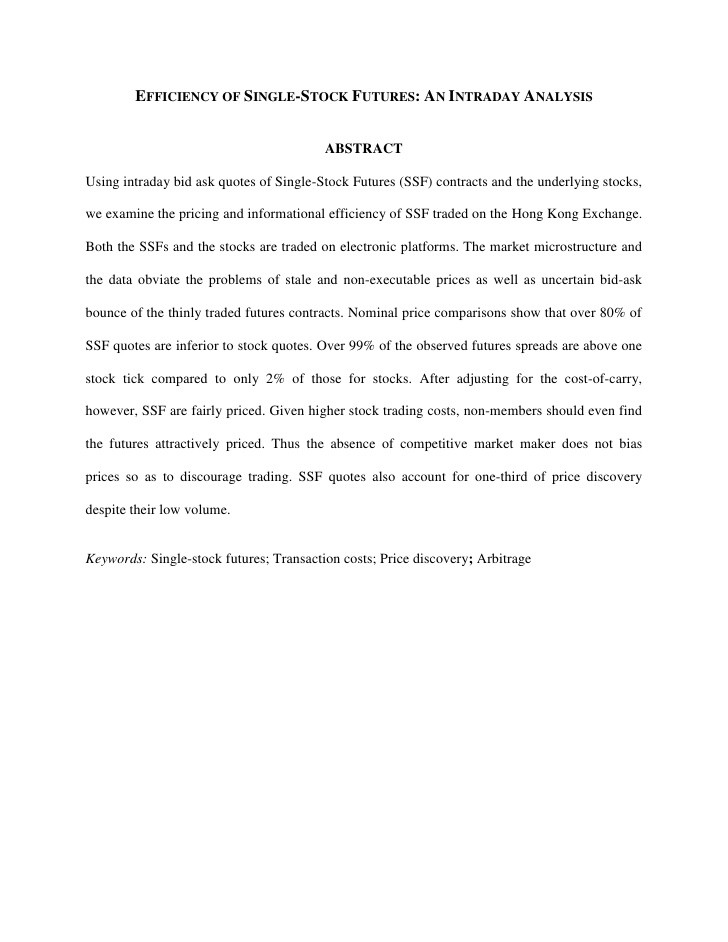
i.e. less visible firms.
The last hypothesis is also related to the results reported by Cohen, Diether, and Malloy
(2007) and Beber and Pagano (2010). Cohen, Diether, and Malloy (2007) find that an increase
in shorting demand leads to negative abnormal returns of 2.98% in the following month,
and more significantly, their results are stronger in environments with less public information
flow, suggesting that the shorting market is an important mechanism for private information
revelation. Beber and Pagano (2010) find that short-selling bans are associated with decrease in
liquidity as measured by daily bid-ask spreads and that this effect is more pronounced for small-
cap and more volatile stocks. However their study falls into before-vs.-after studies in which the
short-selling bans are triggered by recent market changes. Our paper uses high-frequency data,
which give us the possibility to look at intraday spreads and more importantly to decompose
it into order-processing and adverse-selection components, which is the core issue behind the
Diamond and Verrecchia (1987) model. The high-frequency data also allow us to study order
imbalances, relative depths of the limit order book, and intraday volatility in an order-driven
market.
Another important issue is whether short-sales are connected to market crashes. Bernardo
and Welch (2004) suggest that fear of financial crises, rather than fear of a real liquidity shock, is
the true cause of financial crises. One implication of their model is that implementing constraints
that hinder some market participants from front-running other investors can effectively prevent
financial crises from occurring. This implication is consistent with the finding of Allen and Gale
(1991) that short-sales can potentially destabilize an economy. On the other hand, Hong and
Stein (2003) argue that if some investors are constrained from selling short, their accumulated
unrevealed negative information will not be manifested until the market begins to drop, which
further aggravates market declines and leads to a crash. The Bai, Chang, and Wang (2006)
rational-expectations equilibrium model suggests that in the absence of information asymmetry,
short-sale constraints reduce stock price volatility because they limit the range of fluctuations in
aggregate stock demand. But in the presence of information asymmetry, short-sale constraints
can cause the price volatility to increase as less-informed investors perceive higher risks and
Page 12
demand larger price adjustments in accommodating trades. Therefore, we expect that for less-
opaque, more-visible firms, once the short-sale prohibition is lifted the volatility will rise and
that for more-opaque, less-visible firms, the volatility will drop. Kraus and Rubin (2003) model
also predicts that volatility could either increase or decrease depending on the parameter values
of the model.
Thus, we also investigate the individual stock’s volatilities using the realized intraday
volatility measure developed in Andersen, Bollerslev, Diebold, and Labys (2003).Previous
studies on the effect of short-sale constraints have relied on daily data to estimate stock volatility
and skewness. We use a realized volatility measure calculated using transaction data, and the
realized volatility measure has been shown to be more precise than those calculated using daily
data (e.g. Andersen, Bollerslev, Diebold, and Labys, 2003).
In his study of the 1930s U.S. short-sale regulation changes, Jones (2008) finds that among
the three events for which short-sales were more tightly regulated, volatility did not change
significantly in two of the events, while in the third event there was weak evidence that volatility
actually became higher afterwards. Bris, Goetzmann, and Zhu (2007) test whether short-sale
constraints stabilize or destabilize financial markets by examining the skewness of market and
individual stock returns in 46 countries. They find strong evidence that in markets where short-
selling is either prohibited or not practiced, market returns display significantly less negative
skewness. However, at the individual stock level, short-sales restrictions appear to make no
difference. Charoenrook and Daouk (2008), in their cross-country study of 111 countries, find
that when short-selling is possible, aggregate stock returns are less volatile.They find no
evidence that short-sale restrictions affect either the level of skewness of returns or the probability
of a market crash. Chang, Cheng, and Yu (2007), using daily returns from HKSE, find that
when short-sales are allowed, individual stock returns exhibit higher volatility and less positive
skewness. Diether, Lee, and Werner (2009) find that relaxing short-sale constraints increased
market volatility for their NYSE sample but not the NASDAQ sample. Alexander and Peterson
(2008) find no effects on volatility when short-sale constraints are suspended either in NYSE
or in NASDAQ. Ho (1996) finds an increase in stock return volatility when short sales were
restricted during the Pan Electric Crisis in the Singapore market in 1985–1986.
Page 13
III. Methodology and Data
In this section, we first describe the overall methodology for conducting our tests, then we
describe our sample selection and provide summary statistics, and finally we conclude with the
construction of our measures of market quality.
A. Methodology: Difference-in-Difference Estimation
We first consider a simple time-series comparison of means or medians of the values of
interest in determining the impact of short-sale regulation changes on the following proxies of
market quality: proportional quoted spread, proportional effective spread, order-processing and
adverse-selection costs, and intraday return volatility. The construction of these measures will
be discussed later in this section. It is possible, however, that the time-series comparison may
simply capture the secular trend for the market as a whole for the measures. This is particularly
plausible if the pre-event and post-event windows that we choose are too long. Therefore, in
most of our analyses, we intentionally choose a short event window around short-sale regulation
changes. To further minimize such a possibility, we also construct a market capitalization, price
and dollar volume matched sample, and we examine whether there is any secular trend in this
control sample.7To mitigate the impact of time-series trends when examining the short-sale
regulation changes’ effects, we use the difference-in-difference regression approach (Meyer, 1995;
Bertrand, Dufflo, and Mullainathan, 2004; Angrist and Krueger, 2001). Specifically, we estimate
the following difference-in-difference regression:
yi= α0+ β1Shortablei+ β2NoRulei+ β3Periodi+ β4Groupi+ γXi+ ?i,
(1)
where yiis the individual stock’s average market quality measure (e.g. spread, intraday volatility,
etc.) during either the pre- or post-event window. Shortableiequals one if the stock is eligible
for short sale, NoRuleiequals one if the stock eligible for short sale is during the period without
the uptick rule, Periodiequals one if the observation lies in the post-event period, and Groupi
7We choose these variables because they have been shown to be related to cross-sectional difference in spreads
in other studies. For a survey, see Appendix A of United State Security and Exchange Commission’s report on
the comparison of order executions across equity market structure (2001). The document can be obtained from
www.sec.gov/pdf/ordrxmkt.pdf.
Page 14
equals one if the observation belongs to the treatment group (stocks that ever become eligible
during our sample). Xiis a vector of stock-specific control covariates to mitigate the imperfect
matching of treatment and control groups. In the regressions, we use the logarithm of individual
stocks’ market capitalization, inverse of the price, and logarithm of dollar volume. For both the
treatment group and control group, the pre- (post-) window values of the control covariates are
measured at the beginning of the month prior (the end of the month after) a stock’s becoming
eligible for short sale. The testable hypotheses boil down to testing: (1) whether the event of
stock becoming eligible for short-selling impacts individual stock market quality, or β1= 0; (2)
whether the event of “no uptick rule” impacts individual stock market quality, or β2= 0 ; and
(3) whether the removal of the short-sale constraint during the no-uptick-rule period impacts
individual stock market quality, or β1+ β2= 0.
Note that we have average pre-event and post-event measures for both the treatment sample
and the control sample. So if there are N stocks that ever became shortable, then the number
of observations in regression (1) is 4N. As a convention, in the tables in which we report the
number of observations, we only report the number of stocks eligible for short-sale rather than
the total number of observations in the regression. Bertrand, Dufflo, and Mullainathan (2004)
point out several methodological issues in the application of difference-in-difference estimation.
Their simulations illustrate that the treatment effects could be significantly overestimated when
the pre- and post-event windows contain repeatedly sampled observations without appropriate
adjustments. Their evidence also suggests that the t-values we compute for the treatment effect
are rather conservative.
We further acknowledge that the selection of stocks to the short-list is not an exogenous
event. According to the HKSE, stocks are selected based on market capitalization and turnover
ratios, and both criteria are associated with the market microstructure attributes that we are
examining here. Therefore, our empirical analysis is not carried out in a “natural experiment”
but a “quasi-natural experiment” setting (using the terminology of Meyer, 1995). We take into
account such selection bias by using a difference-in-difference approach and by using individual
the dummy variables Groupi and Periodi are significantly different from zero, the control is
particularly problematic. Fortunately, in many of our difference-in-difference estimations, none
of the coefficient estimates from Groupior Periodiindicates any serious problem.
B. Data
We rely on five data sources. The data on the stock returns, number of shares outstanding,
and dollar trading volume are from the Daily Stock Price and Returns File — Hong Kong, the
Pacific-Basin Capital Markets Databases (PACAP). The PACAP data cover all stocks listed on
the HKSE for the period January 1995 to December 2002. Unfortunately, PACAP stops covering
the HKSE stocks after December 2002, so we supplement this information for 2003 and 2004
from our second database, the Standard and Poor’s Global Advantage Issues database. Since the
PACAP and Standard and Poor’s Global Issues databases do not share a unique common link,
we hand matched them based on ticker symbol, SEDOL/CUSIP code, and company name. Our
third data source is the intraday transaction data from the HKSE. Because the HKSE started
to release the bid-ask records only in March 1996, our sample of complete intraday transaction
data starts from March 1996 and ends in December 2003.8
These data (hereinafter “HKTAQ”) are similar to the Trade and Quote database (TAQ)
released by the NYSE. These data contain two files: Trade Records and Bid-Ask Records.9
However, these data differ from the TAQ data provided by the NYSE in two aspects. First,
the Bid-Ask Records file provided by HKSE records not only the best bid and ask prices and
8Therefore, our sample excludes the first batch of 17 Hong Seng Index constituent stocks. Additionally, even
though in most of our empirical exercise, we require the [-30, +30]-day event window, we relax this particular
requirement for the stock introduced to the short-sale list on March 25, 1996 (since for this batch, the trade and
quote data only have 25 calendar days before the event). If we exclude all of this batch of observations, we lose
a large number of stocks eligible for short sale.
9The trade and bid-ask files provided by the HKSE have two identifiers: STKCODE and STK ID. STKCODE
is not unique and can be reused once the company is delisted from the HKSE, but STKCODE is unique. In the
cleaned HKTAQ database on the server, we retain STK ID. The I/B/E/S International Summary File contains
both the STKCODE (albeit under the different name called EXCHANGE TICKER) and SEDOL Code. The
DataStream database contains the Local Code, where the first two digits are for country/region and the other
digits are for local exchange code. That is, we may link HKTAQ, I/B/E/S, and DataStream together via the
STKCODE. An alternative source of accounting information is Standard and Poor’s Global Advantage. However,
there is no common link identification from Global Advantage to the other databases; even though we can locate
the variant of SEDOL code, it does not seem to match the other three databases. So we hand matched Global
Advantage with the other databases. To check the consistency of our matching, we also referenced the Master
file of the HK Trade and Quotes database, DataStream, and I/B/E/S. As the ticker symbols may be reused by
different issues in the HKSE, the name match is of critical importance to ensure the integrity of the matching