Quantitative selection of hedge funds using data envelopment analysis
Post on: 17 Июль, 2015 No Comment
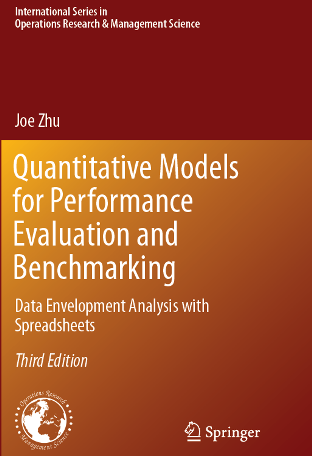
ABSTRACT Previous studies have documented that Data Envelopment Analysis
(DEA) could be a good tool to evaluate fund performance,
especially the performance of hedge funds as it can incorporate
multiple risk-return attributes characterizing hedge fund’s non
normal return distribution in an unique performance score. The
Abstract
Previous studies have documented that Data Envelopment Analysis (DEA) could
be a good tool to evaluate fund performance, especially the performance of hedge funds
as it can incorporate multiple risk-return attributes characterizing hedge fund’s non
normal return distribution in an unique performance score. The main purpose of this
paper is to generalize this framework and to extend the use of DEA to the context
of hedge fund selection when investors must face multi-dimensional constraints, each
one associated to a relative importance level. Unlike previous studies which used DEA
in an empirical framework, this research puts emphasis on methodological issues. I
showed that DEA can be a good tailor-made decision-making tool to assist investors in
selecting funds that correspond the most to their financial, risk-aversion, diversification
and investment horizon constraints.
JEL CLASSIFICATION: G2, G11, G15
KEYWORDS: hedge funds, data envelopment analysis, performance measures, Sharpe
ratio, fund selection.
∗Ph.D candidate at Laboratoire d’Economie d’Orl´ eans (LEO), Universit´ e d’Orl´ eans, Rue de Blois, B.P.
6739, 45 067 Orl´ eans Cedex 2. E-mail: Huyen.Nguyen Thi Thanh@univ-orleans.fr.
†Previous drafts of this paper were presented in the 2006 International Conference of the French Finance
Association on june 26th 2006 and the Research Seminar of LEO on November 25th 2005. I am grateful
to Georges Gallais-Hamonno (LEO), Michel Picot (LEO), Christophe Hurlin (LEO), Philippe Gillet (IAE
Poitiers) and Jean Moussavou (ESC Bretagne Brest) for their helpful comments and suggestions. I thank
the company Standard & Poor’s, especially Ms Marina Ivanoff and Mr Zahed Omar, for providing hedge
fund data used in this study.
halshs-00067742, version 2 — 14 Dec 2006
Author manuscript, published in (2006)
Page 2
1Introduction
The highly successful performance of the so-called hedge funds over the past two decades,
notably during the long bull equity market of the 1990s, has made them quickly well-known
to financial communities as well as to the public. After several years of outstanding growth
(60% in 2000, 40% in 2001), the net inflow of money into this industry is still recorded at 15
% in 2004 to end at $1 trillion, and a growth rate of 10-15% is estimated for 20051. While
hedge funds still manage only a fraction of the $8 trillion invested by mutual funds, their
assets have ballooned from only about $150 billion a decade ago. The main reason behind
this magic success is that hedge funds are believed to be able to generate superior returns
regardless of market environment, and thus offer investors a means to enhance returns,
reduce risk exposure and diversify portfolios invested in traditional assets. This argument
seems to be particularly attractive in the difficult and highly volatile environment that has
prevailed in equity and monetary markets since the early 2000s.
This increasing multiplication of hedge fund industry makes the investment choice quite
challenging for investors. With over 8,000 hedge funds now available, investors need efficient
tools to select the best funds in which to invest. In general, the selection of funds is assumed
to be essentially based on funds’ past performance, that is the ratio of returns adjusted to
risks. According to traditional financial theories, investors make their investment choices
by considering simultaneously the returns approximated by the mathematical mean return
and the risks measured either by the return dispersion (represented by variance or standard
deviation) or by the correlation with the market (defined by beta). Many of them though
validated in mutual fund and pension fund contexts might not be really adequate for hedge
funds.In fact, hedge fund returns are much different from those of mutual fund and
pension fund ”buy-and-hold” portfolios in several ways2. On the one hand, they are usually
asymmetric and kurtotic, a characteristic largely imputed to the intensive use of short sales,
leverage, derivative instruments and to the free call-option like incentive fee structure, all
specific to only the hedge fund industry. On the other hand, their short-term movements
across diverse asset categories and the market neutral absolute investment objective of
hedge fund managers make it really delicate to identify market factors necessary to the
use of multi-factorial models3. Recent techniques enlarge the evaluation dimension to the
1According to a Morgan & Stanley’s report.
2Unlike other kinds of investment funds, hedge funds are loosely regulated, and in many cases, are largely
exempted from legal obligations as the case of offshore hedge funds. Hedge fund managers thus have a broad
flexibility in determining the proportion of securities they hold, the type of positions (long or short) they
take and the leverage level they make. As a consequence, they are free to make very short-term movements
across diverse asset categories involving frequent use of short sales, leverage and derivatives to attempt to
time the market.
3Betas and R2obtained from market models are usually very low or insignificant.
halshs-00067742, version 2 — 14 Dec 2006
Page 3
skewness or/and the kurtosis, and even other higher moments in order to take into account
the non normality of return distributions (see, for example, Sortino & Price (1994); Leland
(1999); Stutzer (2000); Keating & Shadwick (2002); Gregoriou & Gueyie (2003); etc.).
Yet, there are suggestions that actual selection criteria, in fact, may be more compli-
cated and differ significantly from theoretical formulations since there are more attributes
to consider, each one being associated with a priority level. Apart from risk-return charac-
teristics, investors are also concerned about funds’ performance over various time-horizon,
about lock-up period, incentive fees, manager’s reputation, manager’s seniority and perhaps
other qualitative criteria. In addition, although investors share the same selection criteria,
importance level that each investor attach to each criterion is not necessarily the same be-
cause each investor has his own budget, diversification constraints, investment horizon and
consequently different priorities. Even when fund selection is made solely on the basis of
their risk and return, given the wide variety of risk and return measures without no measure
absolutely dominant, an investor may want to consider several of them at the same time,
with or without particular interest a priori to one (some) among them.
The need to simultaneously consider several criteria while incorporating selector own
preferences is particularly important for institutional investors such as pension funds, mu-
tual funds or endowment funds whose clients do not usually share the same financial objec-
tive, risk aversion, investment horizon, etc. From such a multi-objective decision-making
perspective, the Data Envelopment Analysis approach (hereafter, DEA) seems particularly
appealing as it provides the possibility of incorporating many criteria at the same time,
together with the control over the importance level paid to each criterion by means of a
tailor-made optimizing system. Unlike other performance measures, DEA does not provide
a complete ranking of funds. Instead, it only shares them out between efficient (dominant)
and inefficient (dominated) sets. The efficiency is simply relative to other funds in the
same category and thus can be changed once the considered sample is modified. Never-
theless, relative evaluation is a well-established concept in economic literature (Holmstrom
1982). Moreover, the relative property of fund evaluation is quite valuable because in the
Page 4
establishments, etc.), commerce (to evaluate supermarkets) and finance (to evaluate bank
branches, institutions of micro-finance, stocks, etc.). Recently, it has been used to assess
empirically the relative performance of mutual funds and hedge funds4.
The main purpose of this research is to study how the DEA method can be adapted
to the context of hedge fund selection. Unlike previous works that used DEA to evaluate
empirically the performance of the hedge fund industry, I rather put emphasis on method-
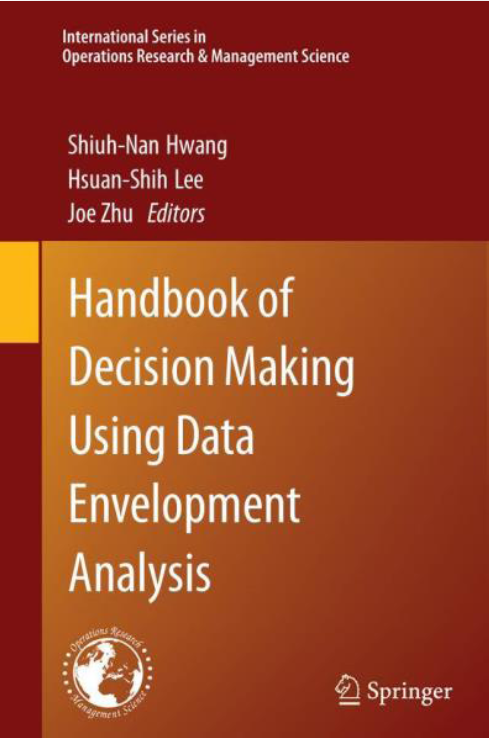
ological aspects of fund selection when investors must face multiple constraints, each one
associated to a different important level. I showed that DEA can be a good tailor-made
decision-making tool to assist investors in selecting funds that correspond the most to their
risk-aversion, financial, diversification and investment horizon constraints. To the best of
my knowledge, this study is the first to deal with this question.
The remainder of the paper is organized as follows. After a succinct review of studies
related to this research in section 2, section 3 introduces basic concepts of the DEA method.
This research emanated from two main streams of literature. The first one concerns the
application of DEA into making a selection when decision-makers have multiple objectives.
The second is about the use of DEA to evaluate empirically the performance of investment
funds.
With respect to the first literature, I refer especially to two important projects on siting
Page 5
safety indexes regarding earthquakes and volcanoes, access to an international airport, ease
of land acquisition, landscape, water supply, matters with historical associations, etc.5. A
common interesting point of these two siting analyses is that the evaluators, basing on prior
expert knowledge about the relative importance of chosen criteria, fixed lower and upper
bounds to the weights associated to each criterion in the mathematical optimization6.
The second literature which motivated my work is related to studies using DEA to
evaluate empirically the performance of mutual funds, ethical funds and more recently
hedge funds (see Table 1 for a summary). Studies on mutual funds include Murthi et al.
(1997), McMullen & Strong (1998), Choi & Murthi (2001)7, Basso & Funari (2001), Tarim
& Karan (2001) and Sengupta (2003). The common point of these work lies in supposing
that fund performance is a combination of multiple fund attributes such as mean returns
(outputs), risk (total or systematic) and expenses8, and sometimes even fund size, turnover
speed and minimum initial investment (inputs). Employing essentially basic DEA models
like CCR (Charnes et al. 1978) or BCC (Banker et al. 1984), they sought to compare the
efficiency of funds within a category or between several different categories of funds.
In the same vein, Basso & Funari (2003) found that DEA is particularly adapted to
assess the performance of ethical mutual funds. They suggested including in the outputs
an indicator measuring funds’ ethical level fulfillments. As argued by the authors, ”the
solidarity and social responsibility features that characterize the ethical mutual funds satisfy
the fulfillment of humanitarian aims, but may lower the investment profitability”. Hence,
we should not disregard the ethical component when evaluating ethical mutual funds.
The application of DEA in evaluating hedge funds emerged from the work of Gregoriou
(2003) and has been supported by Gregoriou et al. (2005)9and Kooli et al. (2005). One
common point among these studies is considering only risk-return performance without
referring to fees. They approximated outputs (what investors seek to maximize) by right-
hand-side values of return distribution and inputs (what investors seek to minimize) by
left-hand-side values. Hence, the inputs include (1) lower mean monthly semi-skewness,
(2) lower mean monthly semi-variance, and (3) mean monthly lower return; the outputs
include (1) upper mean monthly semi-skewness, (2) upper mean monthly semi-variance,
5I didn’t have access to documents related to this project. All the information mentioned is extracted
from Cooper et al. (2000), p.169.
6A detailed example is presented in Appendix 1.
7Choi & Murthi (2001) is in fact the extended version of their seminal work (Murthi et al. 1997). In the
later version, the constant returns to scale assumption is replaced by the variable returns to scale.
8The concept of expenses differs from study to study. It might include transaction costs and administra-
tion fees (totaled in expense ratio) and loads (subscription or/and redemption costs).
9Gregoriou et al. (2005) is an extended version of Gregoriou (2003) and more complete while employing
the same DEA methodology with Gregoriou (2003). Therefore, I will refer only to Gregoriou et al. (2005).
halshs-00067742, version 2 — 14 Dec 2006
Page 6
Table 1: Studies on invesment fund performance within the DEA framework