Price trends and patterns in technical analysis A theoretical and empirical examination
Post on: 16 Июнь, 2015 No Comment
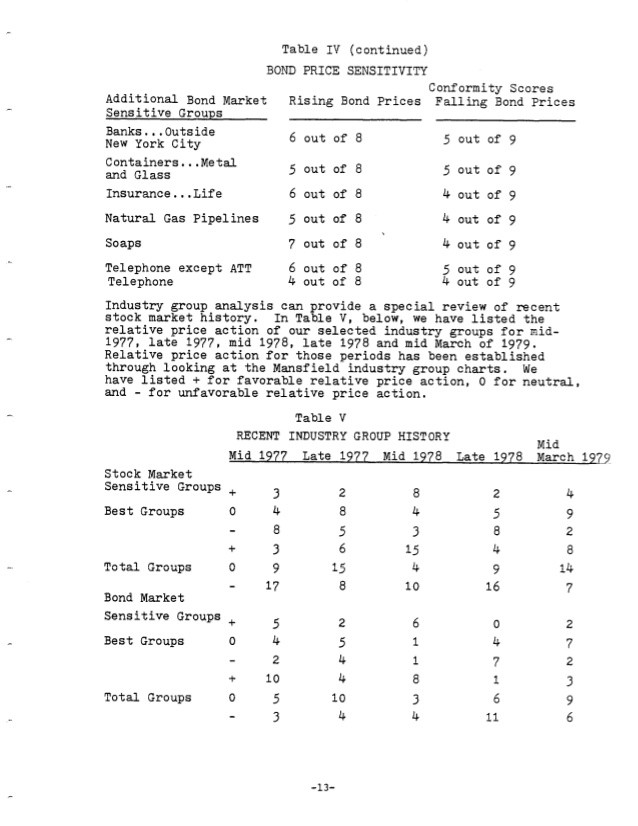
Page 1
Price Trends and Patterns in Technical Analysis:
University of Iowa
Geoff C. Friesenb
University of Nebraska-Lincoln
Lee M. Dunhamc
University of Nebraska-Lincoln
Draft Date: August 2007
JEL Classification: G14, G11, G12, C1
Keywords: Technical Analysis, Trading Rules, Equity Jumps, Momentum, Confirmation Bias
a Henry B. Tippie College of Business, Department of Finance, S252 Pappajohn Business Bldg. Iowa City, IA
52242-1994. Email: paul-weller@uiowa.edu. Tel: 319.335.1017. Fax: 319.335.3690.
b Corresponding author. Department of Finance, 237 CBA, Lincoln, NE 68588-0490. Email: gfriesen2@unl.edu.
Tel: 402.472.2334. Fax: 402.472.5140.
c Department of Finance, 233 CBA, Lincoln, NE 68588-0490. Email: ldunham2@unl.edu. Tel: 402.472.2325. Fax:
Page 2
Abstract
We develop a formal model of asset prices in which investors are subject to confirmation bias,
which describes the tendency of individuals to search for and interpret information selectively to
conform to a given set of beliefs. The model produces three notable results. First, the model
generates price patterns which validate certain well-documented trading strategies, in particular
the “head-and-shoulders” pattern. Second, asset prices exhibit negative autocorrelations over
very short horizons, positive autocorrelations over intermediate horizons, and negative
autocorrelations over long horizons, which matches the observed stylized properties of U.S.
equity prices. Third, the model predicts that sequential price jumps for a particular stock will be
positively autocorrelated. Several recent econometric papers have shown that one can identify
significant jumps by comparing realized volatility and bi-power return variation. Using this
methodology, together with tick-by-tick data on all stocks in the S&P 100 index from 1999-
2005, we identify and calculate significant jumps in stock prices. Consistent with the predictions
of the model, we find that jumps exhibit statistically and economically significant positive
autocorrelations.
Page 3
1. Introduction
Technical analysts use information about historical movements in price and trading
volume, summarized in the form of charts, to forecast future price trends in a variety of financial
markets. The claims of technical analysts were initially discounted by the academic community
on the grounds that they were inconsistent with market efficiency. But recent work has called
into question the extent to which markets are fully efficient. There is now convincing evidence
that stock prices display short-term momentum over periods of six months to a year and longer-
term mean reversion (De Bondt and Thaler, 1985; Chopra, Lakonishok and Ritter, 1992;
Jegadeesh and Titman, 1993). This provides support for a particular class of technical trading
rule that is designed to detect persistent trends. Such rules have been shown to perform profitably
in foreign exchange markets (Dooley and Shafer, 1983; Sweeney, 1986; Levich and Thomas,
1993; Neely, Weller and Dittmar, 1997). There is also evidence of economically significant price
reversals over short time horizons of a week to a month (Jegadeesh, 1990; Jegadeesh and
Titman, 1995; Lehmann, 1990; Gutierrez and Kelley, 2007).1
Various theoretical arguments have been advanced to explain the observed patterns of
momentum and reversal (see e.g. Barberis, Shleifer and Vishny, 1998; Daniel, Hirshleifer and
Subrahmanyam, 1998, Hong and Stein, 1999). These models introduce various departures from
fully rational behavior, and imply that investors using trading rules of the trend-following variety
may be able to profit from these departures from rationality.
The use of technical signals based on price patterns has received less academic attention,
despite the fact that these signals are widely used by practitioners. Chang and Osler (1999)
examine the profitability of using the “head-and-shoulders” pattern in the foreign exchange
1 Conrad, Kaul and Nimalendran (1991) demonstrate that bid-ask bounce explains some of this return reversal.
Cooper (1999) and Subrahmanyam (2005) find that microstructure issues cannot fully explain the documented
return reversal.
Page 4
market to predict changes of trend, and find evidence of excess returns for some currencies but
not others. Lo, Mamaysky and Wang (2000) develop a pattern detection algorithm based on
kernel regression. They apply this methodology to identify a variety of technical price patterns
including “head-and-shoulders” in the U.S. stock market over the period 1962 — 1996. They find
statistical evidence that there is potentially useful information contained in most of the patterns
they consider. Savin, Weller and Zvingelis (2007) show that a modified version of the algorithm
of Lo, Mamaysky and Wang applied to the “head-and-shoulders” pattern has substantial
predictive power for U.S. stock returns over periods of one to three months.
The aim of this paper is to develop a theoretical framework that can account for the
apparent success of both trend-following and pattern-based technical trading rules. We introduce
a single cognitive bias into the model, that of confirmation bias. The bias is a phenomenon that
has been extensively documented in experimental studies. It refers to the search for, or the
interpretation of evidence in ways that favor existing beliefs or expectations. It has been
described as “perhaps the best known and most widely accepted notion of inferential error to
come out of the literature on human reasoning.” (Evans, 1989, p.41 quoted in Nickerson, 1998).
A related phenomenon has been extensively investigated in the management literature
under the heading of “escalation of commitment.” This research seeks to provide explanations
for commitment within organizations to losing courses of action. Theoretical explanations often
focus on the theory of cognitive dissonance (Festinger, 1957). It is argued that people who are
responsible for poor decisions seek to rationalize them by biasing their interpretation of
information relevant for assessing the outcome of the decisions. A study of the banking industry
found that bank executive turnover predicted both provisions for loan losses and the write-off of
bad loans (Staw, Barsade and Koput, 1997). The implication of these findings is that those
Page 5
individuals responsible for making the original loan decisions exhibited systematic bias in their
interpretation of information about the status of the loans.
A specific example of how confirmation bias is recognized as a potential source of
inefficiency within the investment community is provided by Camerer and Loewenstein (2004,
p.17). They report how an investment banker had described the way in which his firm combated
the effects of traders’ “emotional attachment to their past trades” by periodically forcing traders
to switch positions with each other. In a study looking at dissonance effects in the context of
mutual fund investment, Goetzmann and Peles (1997) found that even well-informed investors
had a tendency to favorably distort their perceptions of the past performance of funds that they
held. This may explain the observed asymmetry between investment flows into winning funds
and out of losing funds (Ippolito, 1992).
Confirmation bias has also been shown to manifest itself in group decision making
(Schulz-Hardt, Frey, Lüthgens and Moscovici, 2000). Using a sample of middle managers from
banks and industrial companies, the experiment involved analysis of a case study in which a
company has to decide whether or not to proceed with a large investment. Subjects were required
to come to a preliminary conclusion individually before being combined into groups. At this
point they were given access to additional information. Groups that agreed in their preliminary
conclusions showed a strong preference for accessing supporting rather than conflicting
information. This finding is of particular interest in the present context, since many portfolio
investment decisions are the outcome of group deliberations.
In our model, information arrival is modeled with signals of various magnitudes, arriving
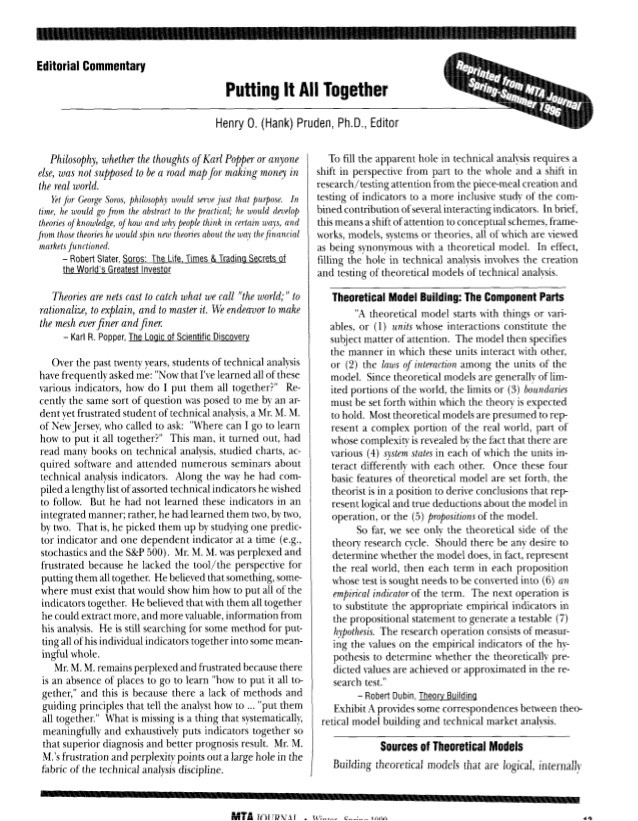
at differing frequencies. Large, infrequently observed signals are interpreted rationally by
investors. However, investors’ interpretation of less informative signals (which arrive more
Page 6
frequently) is biased by the recently observed large signals. The model generates price patterns,
most notably the “head-and-shoulders” pattern, which have the predictive power for future stock
returns claimed by technical analysts. The model thus provides a theoretical foundation for
several price patterns commonly used by technical analysts. The model also produces the well-
documented pattern of price momentum which can be exploited by trend-following technical
rules such as those based on the comparison of short- and long-run moving averages.
In addition, our model makes several predictions. First, return autocorrelations are
negative over very short horizons, positive over intermediate horizons, and become negative
again over long horizons. This feature of the model conforms to the empirical properties of U.S.
equity prices described above. Our model also produces a sharp prediction that the time series of
jumps in the price series should be positively autocorrelated. To our knowledge, this is a new
and untested empirical prediction.
We provide empirical evidence that confirms the prediction of our model that sequential
price jumps in equity prices are positively autocorrelated. Specifically, we utilize the statistical
bi-power variation estimation technique to identify all statistically significant jumps in the price
series of the individual component stocks of the S&P 100 Index over the sample period 1999-
2005. We find that sequential price jumps exhibit statistically and economically significant
positive autocorrelations, and that these autocorrelations decay at a rate that is also consistent
with the model.
Our model and empirical tests complement the recent empirical work of Gutierrez and
Kelley (2007). They document negative weekly autocorrelations immediately after extreme
information events, but find that momentum profits emerge several weeks after an extreme return
and persist over the remainder of the year. Moreover, this momentum easily offsets the brief and
Page 7
initial return reversal. Our model produces predictions consistent with this finding. They also
find that markets react similarly to explicit (public) and implicit (private) news, and note that
many behavioral models require investors to react differently to different types of news. In
contrast, our model makes no distinction between public and private news.
The rest of the paper is organized as follows: Sections 2 and 3 present the model.
Section 4 describes various trading rules and relates them to the model. In Section 5 we describe
our jump detection methodology, and in Section 6 present empirical results. Section 7
concludes.
2. The model with a single low-frequency signal
The process by which information is revealed and incorporated into prices is constructed
to capture the important features of a jump-diffusion process in a discrete-time framework. The
jump-diffusion model of stock returns has a long history (Merton, 1976) and recent work by
Barndorff-Neilsen and Shephard (2004) indicates that jumps in equity prices contribute a
significant proportion of total price volatility. Research on empirical option pricing has also
found that introducing jump components into the underlying price series alleviates some of the
pricing biases found in standard models (Bates, 2003). We suppose that there are low-frequency
signals that are more informative than high-frequency signals. One can think of the low-
frequency signals as generating the jumps in the price series, and the high-frequency signals as
generating the diffusion. There is a single representative investor who is assumed to be risk
neutral, and who observes a low-frequency signal (L-signal) at date 0 about the liquidation value
of a security. At subsequent dates, a sequence of high-frequency signals (H-signals) is observed.
At date T, all information about security value is revealed and the investor receives its liquidation
value.
2.1 Model Specification
The risky security has a liquidation value