PreMBA Finance
Post on: 21 Апрель, 2015 No Comment
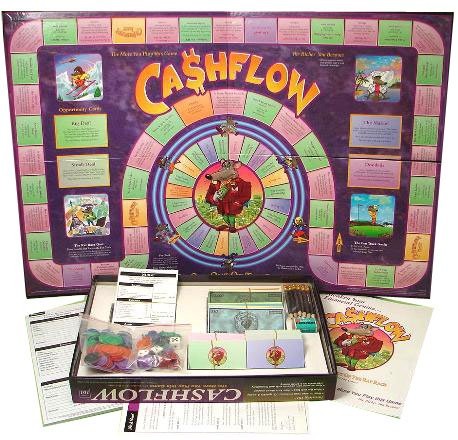
A simple portfolio
Suppose that you have $2 to invest. You can purchase shares in firm Alpha today at a cost of $1 each. There is a 50 percent probability that after one year the stock’s price will rise to $5 (yielding a 400 percent return). There is also a 50 percent probability that the stock price will still be $1 one year from now (yielding, of course, a 0 percent return).
Alternatively, you could purchase shares in firm Beta today at a price of $1 each. There is also a 50 percent probability that Beta’s stock price will rise to $5, and a 50 percent probability that Beta’s stock price will remain at $1.
Because the payoffs and the probabilities are identical, both investments have the same expected return (200% = 400%(.5) + 0%(.5)) and the same risk (the standard deviation is 200 percent in both cases). So you might be tempted to ask: Is there a benefit to purchasing one share of each stock (or diversifying) rather than purchasing two shares of one? The answer depends upon the correlation of the returns for the two stocks.
Scenario one Alpha’s and Beta’s stock prices are perfectly negatively correlated.
Consider these conditions. If average temperatures during the year are above normal, then Alpha’s stock will rise to $5, but Beta’s will remain at $1. If temperatures are below normal, Beta’s will rise to $5, but Alpha’s will remain at $1. Assume that the probability of each temperature outcome is 50 percent. Your total return from different investment options would look something like this:
Notice that the expected total value of your portfolio is $6 in all three cases ((.5 &multiplication;$2) + (.5 &multiplication; $10) = 1 + 5 = $6). But the returns in the third case have a standard deviation of zeroyou are guaranteed $6, no matter what. Even though the two individual investments are risky, there is absolutely no risk associated with the diversified portfolio. However, this is not the case if the two stock prices are related in a different manner.
Scenario two Alpha’s and Beta’s stock prices are perfectly positively correlated.
Suppose that Alpha and Beta’s stock prices always move together. That is, if temperatures are above normal, both stock prices rise to $5. If temperatures are below normal, both prices remain at $1.
Your total returns from the different investment options would look something like this:
Unlike scenario one, the diversified portfolio in this case is no less risky than either of the two individual investment possibilities. The problem is that the stock prices of the two companies are perfectly positively correlated. A perfect positive correlation means that the value of two assets moves in the same direction, by the same percentage, at the same time.
An important principle in finance is that risk reduction cannot be achieved through diversification if the returns on two or more assets are perfectly positively correlated. However, diversification provides benefit if the returns are not perfectly positively correlated. Furthermore, an investor can eliminate all non-systematic risk if the two assets are perfectly negatively correlated, as you saw in scenario one. Risk can seldom be eliminated (reduced to zero) through diversification, as in scenario 1, but it can be reduced.
Correlation Coefficient
To fully understand how the benefits of diversification are evaluated, start by considering the correlation coefficient.
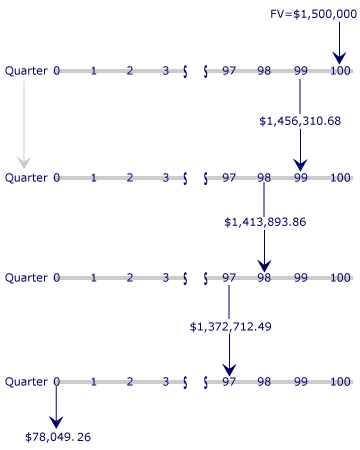
The correlation coefficient measures the degree to which two variables (such as two stock prices; indicated here by subscripts to rho. i and j ) move together. The correlation coefficient must always be between +1 and -1. (+1 indicates the extreme of perfect positive correlation, and -1 indicates the other extreme of perfect negative correlation.) Perfect positive correlation indicates that whenever stock A goes up, stock B goes up by the same percentage.
In the interactive graphic below, drag and drop the purple line onto the graph to match the type of correlation.
In the first graph, the returns of both stocks are perfectly positively correlated similar to what occurred in scenario two above. You can see that when the first stock increases in value, the second stock increases also, by the same amount. This means that no diversification is possible. Graph two shows two stocks that are perfectly negatively correlated as in scenario one above. When the first stock increases in value, the second decreases in value by the same magnitude, meaning that you can eliminate all risk. The final graph shows stocks that have absolutely no correlation. You can see that there is no pattern at all, and that when the first stock increases in value, the second stock sometimes goes up but is just as likely to go down. Typically, the correlation between stocks will track more closely to this pattern than to either of the others.
The formula for calculating the correlation coefficient for two returns, i and j. is
You should already be familiar with the terms in the denominator, which are the standard deviations of the individual returns. As you recall, standard deviation measures the dispersion of observed returns from the mean. The term in the numerator is called the covariance of the two returns and measures how the observed returns move together, or covary.
Covariance and correlation describe the degree to which two variables move together. You can use correlation and covariance to describe whether two stock prices move in the same direction, whether they move in opposite directions, or whether they move completely independently of one another. Covariance and correlation are also used to ascertain whether a stock price moves in tandem with a given economic variable, such as the stock market.
Covariance indicates the degree to which two variables move in unison. A positive covariance means that the variables tend to move together, and a negative covariance means that they tend to vary inversely. Correlation is a normalized measure that describes both the direction and the degree to which two variables tend to move together. To measure the covariance between historical data points, you would examine deviations from the mean for each variable.
Covariance between X and Y = average of (X — X * ) &multiplication; (Y — Y * ) where: