On Uncertainty Market Timing and the Predictability of Tick by Tick Exchange Rates
Post on: 30 Июнь, 2015 No Comment
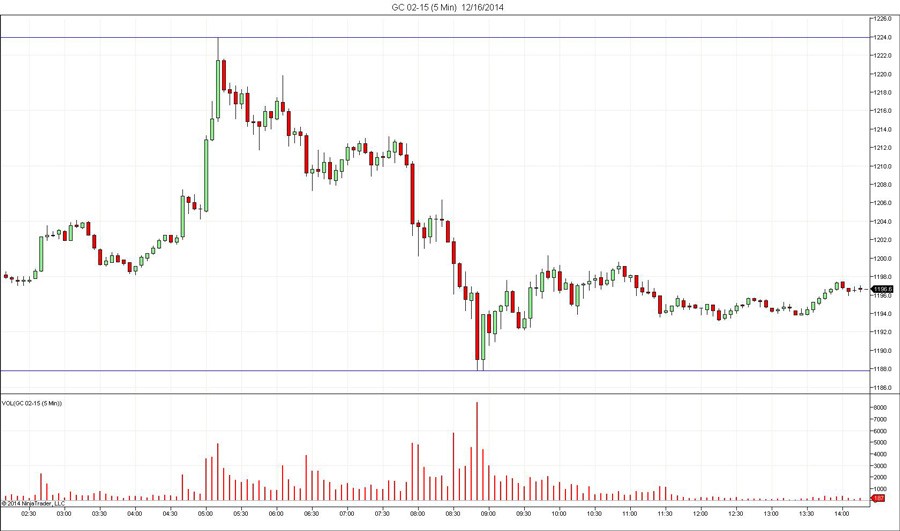
Page 1
WORKING PAPERS SERIES
WP08-06
On Uncertainty, Market Timing and the
Predictability of Tick by Tick Exchange Rates
Roman Kozhan and Mark Salmon
Page 2
On Uncertainty, Market Timing and the
Predictability of Tick by Tick Exchange Rates
Abstract
This paper examines the predictability of exchange rates on a transaction
level basis using both past transaction prices and the structure of the order
book. In contrast to the existing literature we also recognise that the trader
may be subject to (Knightian) uncertainty as opposed to risk regarding the
structure by which exchange rates are determined and hence regarding both the
model he employs to make predictions and the reliability of any conditioning
information. The trader is faced with a two stage decision problem due to this
uncertainty; first he needs to resolve a question of market timing as to when to
enter the market and then secondly how to trade. We provide a formalisation
for this two stage decision problem. Statistical tests indicate the significance of
out of sample ability to predict directional changes and the economic value of
predictability using one week of tick-by-tick data on the USD-DM exchange rate
drawn from Reuters DM2002 electronic trading system. These conclusions rest
critically on the frequency of trading which is controlled by an inertia parameter
reflecting the degree of uncertainty; trading too frequently significantly reduces
profitability taking account of transaction costs.
Financial Econometrics Research Centre,
Warwick Business School
Key words: Knightian uncertainty, market timing, predictability, high-frequency data.
JEL Classification: D81, F31, C53
∗Address for correspondence: Warwick Business School, The University of Warwick, Scarman
Road, Coventry, CV4 7AL, UK; tel: +44 24 7652 2853; e-mail: Roman.Kozhan@wbs.ac.uk and
Mark.Salmon@wbs.ac.uk. We would like to thank Martin Evans, Rich Lyons, Chris Neely, Carol
Osler, Dagfinn Rime, Paul Weller, Lucio Sarno and participants at the ESF Exploratory workshop
on the High Frequency Analysis of Foreign Exchange Markets held at Warwick in June 2006 for their
comments on an earlier version of this paper.
1Introduction
Considerable research has been devoted over a number of years to examining the pre-
dictability of foreign exchange rates following the classic paper by Meese and Rogoff
(1983). One reasonable interpretation of this work is that it has been found to be dif-
ficult to convincingly overturn their result regarding the inability of standard “macro”
based exchange rate models to beat a random walk in out of sample forecasting exer-
cises. Clearly this broad statement could be qualified as research has appeared which
claims to provide evidence of predictability in particular exchange rates and over par-
ticular sample periods (see for instance Abhyankar, Sarno and Valente (2005)) but the
general impression remains that standard fundamentals based models do not consis-
tently dominate a random walk.
At the same time there has been a major development in what has become known
as the New Micro Approach to Exchange Rates (see for instance Lyons (2001)) in
which attention is focussed more on the characteristics and micro structure of the FX
market itself rather than the macro fundamentals that drive the traditional theories of
exchange rate determination. In the light of Messe and Rogoff’s results this distinction
is critical since any statement regarding predictability is necessarily conditional on the
information set employed. A variable may appear to be completely unpredictable if ir-
relevant data is used as the conditioning information and yet may be highly predictable
if the correct conditioning data is used. While both approaches, micro and macro, seek
to explain the same exchange rate which is determined on a tick by tick basis in the
spot market, they obviously differ critically in the information sets they use to explain
exchange rate movements and hence forecast.
In this paper we present what we believe to be the first rigorous tests of pre-
dictability of an exchange rate using irregularly spaced tick by tick FX data where the
information set involves both the past price history and information on the structure
of the order book. Secondly we attempt to formally recognise the uncertainty that
a foreign exchange trader faces given that any model he uses to generate forecasts
and trading decisions will be incorrect in ways that he cannot capture in a unique
probability distribution. We therefore allow our trader’s decisions to reflect Knightian
what surprising result is that the we find little or no advantage in allowing the predictor
to exploit information in the order book. This result is exactly the opposite of what
we had expected before carrying out the empirical work but would be consistent with
the market price acting as a sufficient statistic and hence that no further information
is useful.
Our objective is not to re-examine the question of the predictability of technical
rules but to mirror the way in which technical rules appear to be used in practice
by currency traders when making their trading decisions. In the first place, there is
considerable evidence (see for instance Taylor and Allen (1992), Lui and Mole (1996))
that traders largely use technical rules only for short run decision making, which jus-
tifies our use of tick by tick data. Traders also do not follow a single rule but form
an impression as to where the market is moving on the basis of a number of technical
indicators, dropping those that appear not to have worked well.1Secondly we formalise
the sequential decision making seen in financial markets where an initial decision must
be taken as to when to enter the market – the question of market timing – alongside
the decision of how to trade. The market timing decision is intimately related to the
degree of uncertainty the trader faces; when he is confident in the direction the market
is moving he will act quickly and when he is uncertain he will show inertia and be
reluctant to trade.
Our results would seem to justify the investment that has been made by a number
of financial institutions to develop and apply automatic trading systems2. This does
suggest the correct interpretation of the Messe and Rogoff conclusion lies in the fact that
the correct conditioning information set had not been used rather than that exchange
rates are in fact unpredictable. In addition we show that even when you have the correct
information it is critically how and when it is used that determines if profitability will
appear since unless the frequency of trading is controlled, profitability disappears. The
frequency of trading is controlled by means of an “inertia” parameter reflecting the
degree of uncertainty and ensures the system can only trade when the predicted price
change is beyond some threshold level (taking transactions costs into account). Human
traders clearly do not trade in real time as frequently as our unconstrained trading rules
would suggest and so restricting the frequency of trades simple reflects reality.
In the next sections we briefly review the existing literature in this area and then
discuss the derivation and application of the Genetic Algorithm trading rules, the
testing procedures we have employed before turning to report our results.
1We have benefited from detailed discussions with the chief currency trader at the Bank of England
and the Royal Bank of Scotland regarding their use of technical analysis.
2We know, for instance, of one major bank that has an automatic trading system in place that
inspects 1200 currency pairs in real time and we are told delivers profits of the order of 500 million
Euro annually.
Page 5
2Predictability and Market Timing
Most existing statistical analysis suggests at best weak predictability in the FX mar-
ket. At the same time, we can clearly observe trends, ex post, in exchange rates over
particular periods of time which might imply ex ante short term predictability if the
correct conditioning information can be found and potentially short term arbitrage
opportunities. To state the obvious; the issue of market timing is intimately connected
to the degree of predictability in a market. Even if there is no predictability over a
long period or on average, it may be possible to forecast at some point of time over a
In the paper we use a two stage combined predictor in which the first step provides a
market timing indicator and the second gives a forecast of the future exchange rate and
a related trade. The market timing indicator effectively serves as a filter, extracting
irrelevant or uncertain information and noise from the time series data and then the
trader uses this filtered information set to take decisions in the market.
The more formal description of the individual’s preferences is given in the following
section.
2.1 Uncertainty Aversion and Inertia
An investor in real life is invariably faced with different types of uncertainty while mak-
ing trading decisions in a market. This uncertainty might be due to changing market
structure, estimation error or model uncertainty, lack of information, imprecision of
information of imperfect trading signals. Risk is defined by the probability of events
described by an assumed model structure and a unique probability distribution. We are
more concerned with capturing the uncertainty in decision making, beyond risk, which
results if there is a lack of knowledge about which model and probability distribution
to use. Risk calculus, as employed for instance in VaR or expected utility calculations,
is relatively straightforward and provides a confidence interval as the basis for action.
However, when we recognise that incorrect decisions can follow from inaccuracy in the
Page 6
pected utility fails to be an appropriate model by which to predict or make trading
decisions. Technical indicators may in fact reflect the Knightian concept of uncertainty
more accurately than expected utility based predictors since they are often viewed as
distribution free methods of predicting future prices. However, even technical rules are
recognised as being imprecise and may deliver incorrect forecasts which is why tech-
nical analysts tend to use several technical indicators simultaneously or interval based
rules like Bollinger Bands or stop-loss strategies.
The use of these strategies brings some inertia to the trading process which makes
trading less frequent. This notion of trading inertia is entirely consistent with the
question of market timing since it implies that the investor does not trade at every
feasible point of time but does so by trying to choose the best moment when to enter
the market.
There are several ways how the theory of decision-making under uncertainty can
be used to model the observed inertia in the market. One of the classic examples
is the no-trade condition introduced by Dow and Werlang (1992). Once the trader
observes this condition in the market, he becomes “uncertain” about a particular asset
and excludes it from his portfolio and doesn’t trade the asset again until the condition
disappears. This phenomenon describes some kind of extreme uncertainty aversion
in the agent who will have different degrees of uncertainty regarding each asset in the
market reflecting the different degree of confidence he has in his information set. It may
also be that the investor is uncertain about the market as a whole in that he does not
see value in any alternative to his current position. This implies that his position will
change only if there is another portfolio which clearly dominates his existing portfolio.
2.1.1The Market entry/ exit Decision
Theoretical support for preferences with trading inertia has been introduced by Bewley
(1986) (see also Bewley (2002), Ghirardato, Maccheroni and Marinacci (2004)) and we
now provide a short description of the simplified version of the Bewley’s approach that
we will employ.
Uncertainty Averse Preferences. Let S be a set of states (of the market) and
A2. Transitivity If f ?uag and g ?uah then f ?uah.
A3. Openness For all f ∈ L,
A4. Independence For all f,g,h ∈ L and for all α ∈ (0,1), g. h if and only if
αf + (1 − α)g ?uaαf + (1 − α)h.
Theorem 2.1. (Bewley, 2002) If ?uasatisfies Axioms A1-A4, then there is a closed
convex set P of probability measures on S such that
(i) for all f and g and B ∈ B we have f ?uag if and only if EP(f) > EP(g) for
all P ∈ P;
(ii) for all P ∈ P, P(B) > 0 for each non-empty B ∈ B.
The set of probability measures P reflects the trader’s uncertainty. The key point
in the preceding theorem is that the preference relation ?uais not complete leading to
a set of probability measures P rather than a unique measure as in the expected utility
theory. This means that there may exist two acts which are “incomparable” and the
decision-maker can not distinguish which is better nor in fact whether he is indifferent
as he does not have sufficient information to evaluate the different options. The notion
of incomparability is critically distinct from indifference. The latter means that if the
decision-maker is indifferent between f and g he will definitely prefer f +ε rather than
g if ε > 0. In the case of incomparability the investor needs more information beyond
f in order to compare two alternatives. This may imply that the decision-maker is not
fully rational as discussed in Bewley (2002). If the decision-maker can unambiguously
distinguish between two acts then we say that acts f and g are “comparable” and
denote this by f ≶uag.
There are different solutions as to how to act when there are incomparable portfolios
in the market. One is to randomly choose one of them, another is to assume that the
investor has a subjective distribution over the set of priors (a probability measure
over probability measures) and carries out some form of averaging with respect to his
beliefs. This approach leads to Bayesian Model Averaging and its generalization as
discussed in Klibanoff, Marinacci and Mukerji (2005). Instead we adopt the inertia
approach proposed in Bewley (2002). This implies that the decision-maker will change
his portfolio only if a new portfolio strictly dominates it. In order to formalize this we
Page 8
better alternative to his current portfolio he will not trade until the situation changes.
If a new alternative arises which is preferable according to every measure in the prior
set, the trader will change his current position. In a real market this inertia is also
justified by the presence of transaction costs since an investor would have to pay to
switch to an incomparable portfolio which would not be rational.
Although this representation of Bewley’s preferences allows us to model trading
inertia, it does not provide guidance as to which alternative to choose when faced
with several “incomparable” positions each of which is strictly better than the current
position. For example, Bewley’s preferences do not provide an answer as to what the
decision-maker holding the portfolio f should do if there exist g and h such that g ?uaf
and h ?uaf. It may happen that g and h are not comparable. If this is the case, the
decision-maker will get a signal that he needs to change his current portfolio, given
there are better portfolios than f in the market (both g and h in our case) but he does
not know which alternative, g or h to choose.
In order to fill this gap we propose two different preference relations in the space of
risky payoffs and construct a composite indicator which the decision-maker uses. The
first indicator is based on Bewley’s preferences ?uaas described above which represents
the trader’s perception of uncertainty and serves as a market timing indicator informing
the trader that he should alter his current position. The second indicator, td, (stands
for ”trading”) changes the trading direction and determines the quantity traded. We
denote the corresponding preference relation by ?td. We do not provide any specific
axiomatization for the ?tdpreference relation here as it may be any subjective predictor
based on a complete preference relation that the trader is willing to use: either based
on the expected utility model or a technical indicator or simply the trader’s intuition.
The composite preference relation. is then defined as follows:
g. f ⇔ (g ≶uaf) ∧ (g ?tdf).
Effectively decision-making based on the preference relation. is split into two stages:
market timing and trading. The uncertainty aversion preference relation ?uadoes not
indicate whether one asset is better than another; rather it provides the degree of
confidence for the trader that the td indicator has predictive power at the particular
Page 9
simple example. Assume that there are two assets in the market – one risky and one
risk-free. The agent has only three possibilities: invest his wealth in the risk-free asset,
invest everything in risky asset or take a short position in the risky asset which is equal
to his current wealth. The current wealth level is denoted by W0. The current price
of the risky asset is p0and its future price is a positive random variable p. The gross
return of the risk-free asset is 1+r. In order to define the set of priors for the model we
fix a measure P0on the probability space (S,B). We assume that all measures in the set
P are absolutely continuous with respect to the measure P0. By the Radon-Nikodym
theorem for every measure Q ∈ P there exists a non-negative random variable ηQwith
EP0(ηQ) = 1, such that dQ = ηQdP0. Therefore, we can identify the set of priors P with
the set of their Radon-Nikodym derivatives with respect to the probability measure P0.
The price expectation under the measure Q can be expressed as EQ(p) = ˜ p + kQ,
where ˜ p = EP0(p) and kQ= covP0(p,ηQ). Indeed, EQ(p) = EP0(ηQp) = covP0(p,ηQ) +
EP0(p)EP0(ηQ) = kQ+ ˜ p.
can be calculated by W1= 2W0(1 + r) −W0p
Page 10
Werlang (1992). Under Dow and Werlang’s condition the investor exits the market
and does not re-enter until the condition vanishes. In our case the trader does not exit
the market but waits and holds his current portfolio until a better alternative arises.
We consider the simplest case with two assets in the market – long and short
positions in the foreign currency. If denote by f the position held at time t which was
bought at time t0 for price pt0then we say that there exists a position g such that
f ≶uag ⇔ |pt0− pt| > k for some pre-defined positive k, where ptis the current price
of the asset g. This can be interpreted as implying there is no asset structure in the
market which dominates the one held until the transaction price change is predicted
to be large enough. The constant k reflects trader’s subjective attitude to uncertainty
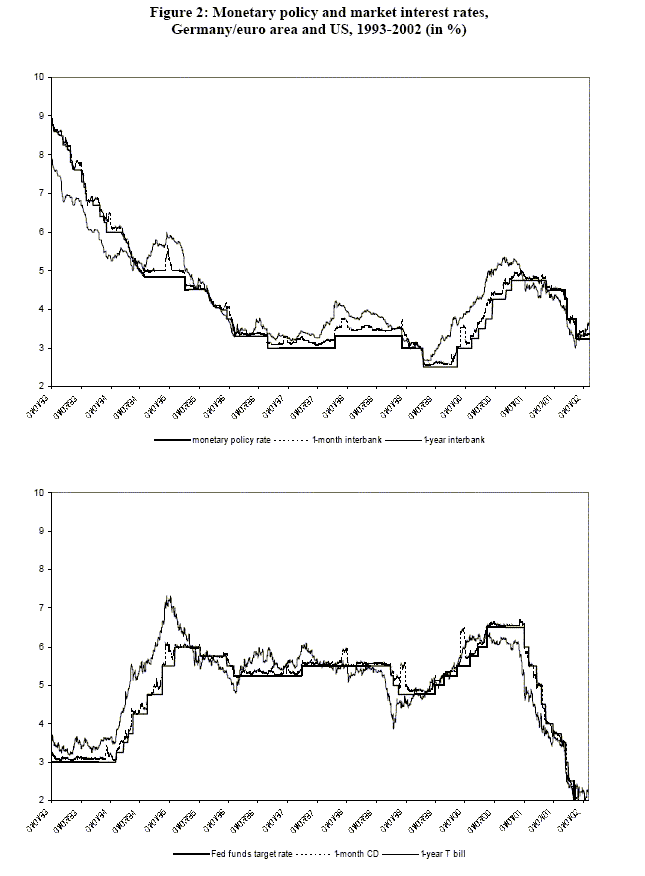
in the market. The larger k, the wider the band of inertia which leads to less frequent
trading. For the sake of simplicity below we use a symmetric interval for the market
timing indicator. Once the price change happens to be big enough the trader uses the
td indicator to determine his action in the market. In our case the td rule is provided
by a genetic algorithm trading rule which is discussed in the following section.
3Technical Analysis and Predictability
The debate as to whether using Technical Analysis results in significant profitability
has probably been running ever since the Dow Theory came into existence between
1900-1902 when Charles Dow suggested that the direction of prices in “The Industrial
Average”, made up of 12 blue chip stocks and “The Rail Average”, made up of 20
railroad companies appeared to be based on a set of rules.
In an early study Fama and Blume (1966), investigated the importance of Technical
Rules while analyzing foreign exchange markets and Dooley and Shafer (1976) obtained
results in favor of the profitability of similar filter rules. Sweeney (1986) confirmed these
positive results and proved their statistical significance. Carol Osler has demonstrated
in several papers (Osler (2003), Osler (2000), Chang and Osler (1999), Chang and
Osler (1995) and Savin, Weller and Zvingelis (2007))the potential for profitability of
more sophisticated technical rules in FX markets such as head and shoulder patterns.
Whereas most technical indicators are based simply on historical prices or returns, other
Page 11
Neely, Weller and Dittmar (1997)) report the profitability from using technical rules
but use daily or weekly data which is clearly unrealistic and misrepresents the informa-
tion set available to the trader in practice when making a trade using technical analysis.
The literature in which high-frequency trading rules are investigated is limited. Curcio,
Goodhart, Guillaume and Payne (1997) consider intra-day FX data aggregated to one
hour and find little or no evidence of profitability outside of periods when the exchange
rate is trending. Neely and Weller (2003) using half hourly quote data find considerable
out of sample predictability which does not translate into profitability once transaction
costs are taken into account which they claim supports the efficiency of the FX market.
The research most closely related to our own is that of Michael Dempster (Dempster
and Jones (2001)) who applied GP generated rules to FX transaction data with one
minute aggregation for trading but with indicators evaluated at fifteen minutes. To
quote from their conclusions — they find “the return from the 20 strategy portfolio
system trading at fifteen minute entry intervals is small and statistically insignificant
and is in fact less than the interest differential between pounds and dollars over the
same sample period. better excess returns would have be available from a static
buy and hold strategy. When only the best strategy is employed it is modestly and
statistically significantly profitable (returning 7%)”.
We seek to extend this literature by focussing on transaction level data — in other
words irregularly spaced transacted prices as opposed to quotes or temporally aggre-
gated data and by including the structure of the order book in the information set
available to the trader or automatic trading system. Secondly as discussed above we
introduce uncertainty into the trading process. We also focus on rigorous testing of
both directional change and economic value and use White’s reality check to immunise
our results from any Data Snooping bias.
3.1The Genetic Trading Rule
We want to consider evaluating predictability as generally as possible and so it is
important not to restrict ourselves to examining the performance of a fixed set of pre-
diction and trading rules. The Genetic algorithm (GA) provides an effective method
forced to impose on the search process. Never the less the rules selected below will have
evolved from searching over millions of competing rules and their performance is there-
fore known to be at least “good” if not achieving the global optimum. This is obviously
sufficient for our purposes since if predictability is found with these “good” rules then
we know we must at least have understated the potential degree of predictability that
might exist. Moreover our results should have much greater applicability than those
who have considered a fixed set of technical rules – however large4.
3.2Genetic Algorithm
Genetic algorithms have been successfully applied in a number of financial applications,
most notably for our purposes, Dempster and Jones (2001), Dworman, Kimbrough and
Laing (1996), Chen and Yeh (1997b), Chen and Yeh (1997a), Neely et al. (1997), Allen
and Karjalainen (1999), Neely and Weller (2001), Chen, Duffy and Yeh (1999), Arifovic
(1994), Arifovic (1995), Arifovic (1996), Arifovic (1997).
Starting from an initial set of rules a genetic algorithm evaluates the fitness of
various candidate solutions (trading rules) using the given objective function of the
optimization problem and provides as an output solutions that have higher fitness val-
ues. Two operations of crossover and mutation are applied to create a new generation
of decision rules based on the genetic information of the fittest candidate solutions
Crossover operation: for the crossover operation one randomly selects two parents
from the population based on their fitness, then take a node within each parent as a
crossover point selected randomly and the subtrees at the selected nodes are exchanged
to generate two children. One of the offspring then replaces the less fit parent in the
population. In our implementation we use a crossover rate of 0.4 for all individuals in
the population. This operation combines the features of two parent chromosomes to
form two similar offspring by swapping corresponding segments of the parents. in our
case these segments are represented by sub-nodes of binary tree. The intuition behind
the crossover operator is information exchange between different potential solutions.
Mutation operation:to mutate a rule one selects one of its subtrees at random
and replaces it with the new randomly generated tree. This operation guarantees the
refreshment of the genetic code within the population. The best 25% of rules are not
mutated at all and the remaining are mutated with probability 0.1. The intuition
behind the mutation operator is the introduction of some extra variability into the
population.
The elements of every trading rule are terminals and operations and the rule pro-
4We are also interested in the simplicity of the rules selected by the genetic program and how
these may correspond to robust decision rules that may justified formally in the face of uncertainty
as opposed to risk in financial markets.
vides a boolean value as the output. If the value of the rule is “true”, it gives the signal
to “buy”USD, or equivalently, it indicates the USD-DM exchange rate is increasing.
If the rule is “false”– the trader “sells”USD. The rules are represented in the form of
randomly created binary trees with terminals and functions in their nodes. We employ
the following choices of operations and terminals;
Operations: the function set used to define the technical rules consists of the binary
algebraic operations <+,−,∗,/,max,min>, binary order relations <<,>,≤,≥,=>, log-
ical operations and unary functions of absolute value and change of
sign.
Terminals: the terminal set contains the variables
lagret2, maxpr5, maxpr10, maxpr20, minpr5, minpr10, minpr20, avgpr5, avgpr10,
avgpr20, avgret5, avgret10, avgret20>, where the first 6 variables indicate currently
observed price and returns of USD in terms of DM and their lag values, maxpr and
minpr as well as maxret and minret denote maximum and minimum price over the
indicated period of the exchange rate and its return respectively, avgpr and avgret
are average value prices and returns over the period. We should make clear that by
“period”we mean the irregular instants of time when the transaction is realized.
For the cases when the algorithm searches over order book information we also use
the following additional variables: bestbid, bestoffer, bestbidq, bestofferq, quantity,
liqbid, liqoff, dpthbid, dpthoffer, time, which represent best bid, best offer, best bid
2). Set i := i + 1.
3). Evaluate fitness of each tree in the population using the fitness function.
4). Generate a new population (i.e. the set of all genetic trees) using the genetic
operations (crossover and mutation)
5). Repeat 2)–5) while i < N.
In the program we use the population size of 100 individuals and provide 50 itera-
tions of the algorithm (that is, N = 50).
The complexity of trading rules are controlled in a probabilistic manner. In fact,
the probability for a binary node to appear in the tree is smaller than the probability
of a unary one which prevents the tree to become very large.
3.3 Fitness functions
A fitness value also needs to be assigned to each of the generated trees or trading rules in
order to solve the optimization problem. We use three different fitness functions. First
we consider the percentage of correctly predicted change of directions in the exchange
rate by the composite technical rule. Although the trader as described in the previous
section, does not make a transaction until the exchange rate changes sufficiently, the
information received during the period when the trader remains passive is used to
compute the current values. For example, if the exchange rate et−1 = et = e, the
values of variables price and lagpr1 are both set to e. We denote the percentage of
correct directional change predictions by DC.