New tools for spread trading
Post on: 10 Апрель, 2015 No Comment
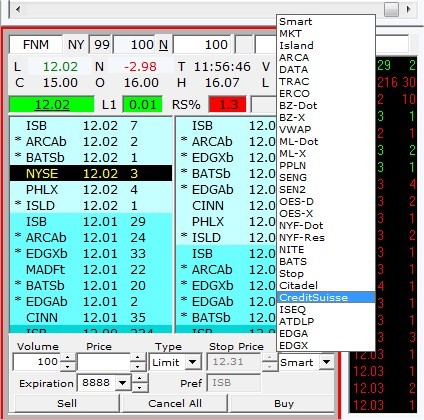
Copulas are tools for modeling the dependence between random variables. The word copula is derived from the Latin word copulare, meaning to connect or to join. Only recently has this theory found its way into statistics and even more recently into finance. Traditionally, this field has been dominated by Gaussian multivariate modeling techniques, which impose rigid assumptions upon the marginal distributions and their joint behaviors. It is now generally accepted that stock prices are rarely normally distributed.
Copulas model the dependence structure between two or more sets of observations without the rigid assumptions of Gaussian techniques. Copulas do this by separating the dependence structure from the marginal behaviors. As such, copulas overcome the problem of estimating a multivariate distribution function by splitting the process into two parts: Determine the margins F and G and estimate the respective parameters; and determine the dependence structure between the variables X and Y by specifying a meaningful copula.
Copulas link the returns of each asset probabilistically, instead of viewing the dependence between two or more risk factors as a single number. The two assets or risk factors are described by two cumulative distributions with range [0,1] and a joining surface, which is the copula.
The fundamentals of copulas, which assume some pre-existing statistical knowledge, are laid out in Copulas 101. For more background information, check out the texts An Introduction to Copulas by R.G. Nelsen and Copula Methods in Finance by U. Cherbuni, E. Luciano and W. Vecchiato.
PAIRS TRADING
Thanks to the much less rigid assumptions that have to be imposed on the data by copulas, regardless of the marginal distributions of stock returns, a suitable joint distribution can be found that provides much richer information than simply a correlation coefficient. It follows, then, that given the optimal copula between two stock returns, relative under- and over-valued positions can be identified. Those positions, in turn, can be exploited.
Thus, if the copula declares stock A to be overvalued relative to stock B, stock B must be undervalued relative to stock A. To apply this strategy you must know the marginal distributions, the relevant copula function and the conditional copula function.
We can demonstrate this procedure with daily closing stock prices of Fannie Mae (FNM) and Freddie Mac (FRE), from July 31, 2007, through Jan. 31, 2008. Data from February 2008 will be set aside for out-of sample validation. These out-of-sample data are not used in calibrating the marginal distributions and the corresponding copula.
To make the data set suitable for obtaining parametric marginal distributions, we need to transform it to log-returns. The marginal distributions fitted to FNM and FRE are known as Laplace and Cauchy distributions. These may be derived using a standard package of statistical analysis software. The procedures here are accomplished using Microsoft Excel, the distribution-fitting software Easyfit and Matlab, although Matlab could handle most tasks itself.
F(FNM) and G(FRE) are uniformly distributed on [0,1]. Equations 3 and 4 (below) are the respective distribution functions of FNM and FRE.
The correlation coefficient between these returns is 0.91, indicating that there is a strong linear relationship between FNM and FRE. More important, however, is what this dependence structure looks like. To gauge this, as well as the structure of the corresponding copula, observing two graphical representations is helpful (see Mae and Mac).
We can see from the scatter plots that there is some upper and lower tail dependence. The second scatter plot provides some clues regarding which copulas may be considered for this pair. Namely, wed look for a copula that can take tail dependence into account. There are two return outliers as well that will be accounted for by the correct copula function.
There is a vast array of copula families, each providing flexibility to capture the specific dependence structure between pairs. A class of copulas known as Archimedean copulas possesses some desirable mathematical properties for this strategy.
The next step is to calibrate the copula, or more plainly, to estimate the copula parameter. There are various ways of doing this and the details are abundant in literature. Well use Canoconical Maximum Likelihood estimation. The copula used can be found on page 95 of An Introduction to Copulas.
Once the relevant copula is specified and calibrated to the data, the next step is to obtain the indicator for relative over- or under-valuation. To accomplish that, well use the partial derivative of the copula with respect to u (FNM) and the partial derivative of the copula with respect to v (FRE).
A summary of the basic idea is as follows:
1. Obtain Pr and Pr
2. Set these conditional probabilities equal to 50% and solve for u and v, respectively.
3. Input these conditional u and v values into their respective quantile functions obtained from their marginal distributions to obtain conditional returns.
4. Obtaining the conditional price is trivial once the conditional returns are known.
5. If the market price of the stock is below the conditional price obtained from the copula, the stock is considered undervalued and a long position is warranted.
The conditional probabilities are set to 50% because the interest lies with the expected value of U given that V takes on a specific value of v; thus, the equation is solved for u, which will equate the conditional probability to 50%.
Another way of interpreting this would be that it is expected that U will be less or equal to u given that V is currently trading at v in the market and vice versa. These u and v values are conditional uniform variables. Because the marginal distributions of FNM and FRE already have been determined, it is easy to work backward to the returns corresponding to the different values of u and v and obtain conditional returns and, ultimately, conditional prices.
The heart of this theory relates to the hypothesis that the copula captures the co-movement between these stocks accurately enough to identify trading signals, which standard linear correlation analysis is not robust enough to accomplish. In its simplest form, if the copula indicates that FNM is overvalued relative to FRE, FRE is considered undervalued relative to FNM.
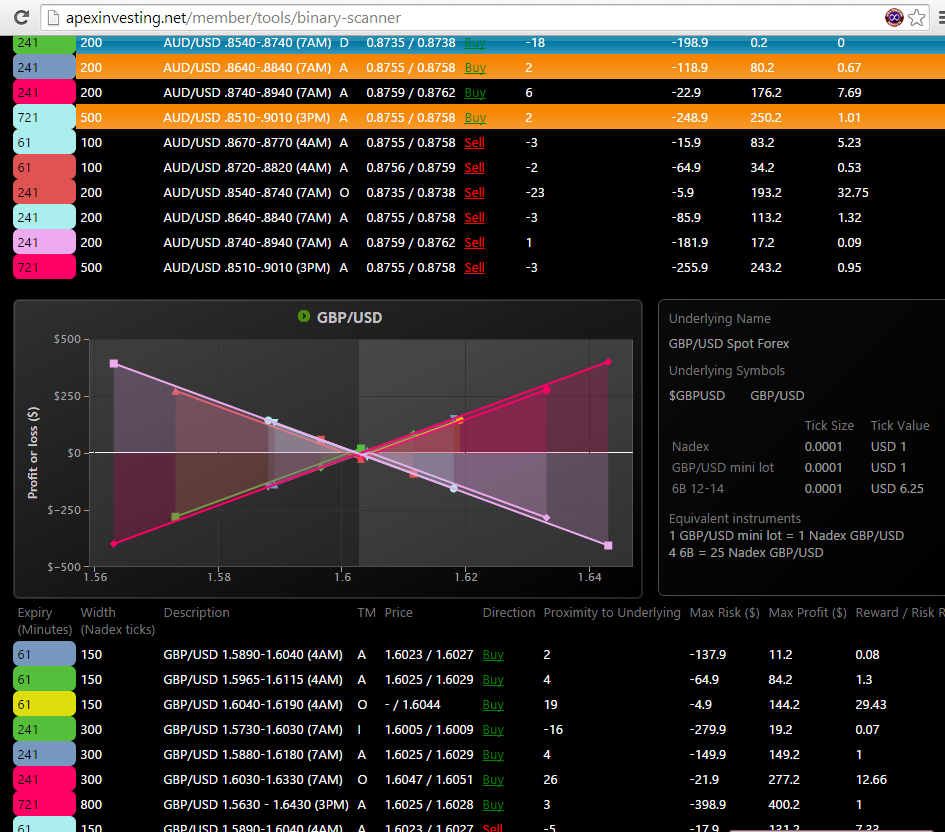
As a prerequisite for success, the pairs should be highly correlated. However, much potential information is lost when considering the correlation coefficient only. Employing a copula-based approach results in a far richer set of information, such as the shape and nature of the dependency between the stock pairs.
OUT-OF-SAMPLE VALIDATION
With the marginal distributions and the copula estimated for the in-sample date, we can backtest the strategy on the out-of-sample data. The results are shown in Profitable pair.
Profitable pair provides a wealth of information. The first two columns are the observed market prices of FNM and FRE. The third and fourth columns are the uniform variables obtained from the marginal distributions. The fifth column is the joint probability of the two stocks; thus, the fifth and sixth columns are the conditional probabilities described above. Comparing the observed market prices to the conditional or theoretical values supplied by the copula identifies an over- or under-valuation.
As a general guideline, stocks are relatively undervalued if the conditional probability is greater than 0.5 and relatively overvalued if the conditional probability is less than 0.5.
Following an example trade is helpful (see Trade example). On Feb. 1, it is observed that FNM is undervalued relative to FRE. The position taken is going long FNM and short FRE. The next day the position remains the same. The situation does not change until Feb. 12. FNM is overvalued relative to FRE, and FRE is undervalued relative to FNM. The position is closed out. The trade was not closed out on Feb. 8 to wait until the conditional probabilities in the seventh column, in this case, are high in the tail region of the conditional distribution; the higher the value, the more certain it may be that the stock is currently undervalued.
An important point to consider is the amount of stock to buy or sell when the position turns around. There are a number of ways to determine a suitable hedge ratio. Equation 5 (below) describes the example here.
Such that the total quantity of the exposure equals 2000 in this case.
Copulas provide flexibility and for such a sophisticated approach are relatively easy to implement. It is also a new approach in the trading arena, and is open to much discovery and further development.
While copulas are useful, no trading strategy is complete without a thorough analysis of the fundamentals behind a particular issue. This is one area ripe for investigation: the relationship between what the copula method indicates and what the fundamentals foretell. This approach will lead to superior understanding about the dependencies that exist between different stocks.
The author thanks Ronald McEwan for his support and guidance in this area of study.
Luan Ferreira is working as a quantitative manager for Credit Risk Analytics in Sydney. The author may be reached at ferreira2@webmail.co.za.