Monte Carlo Simulations iFinancialData Financial and Energy markets news and education
Post on: 3 Июль, 2015 No Comment
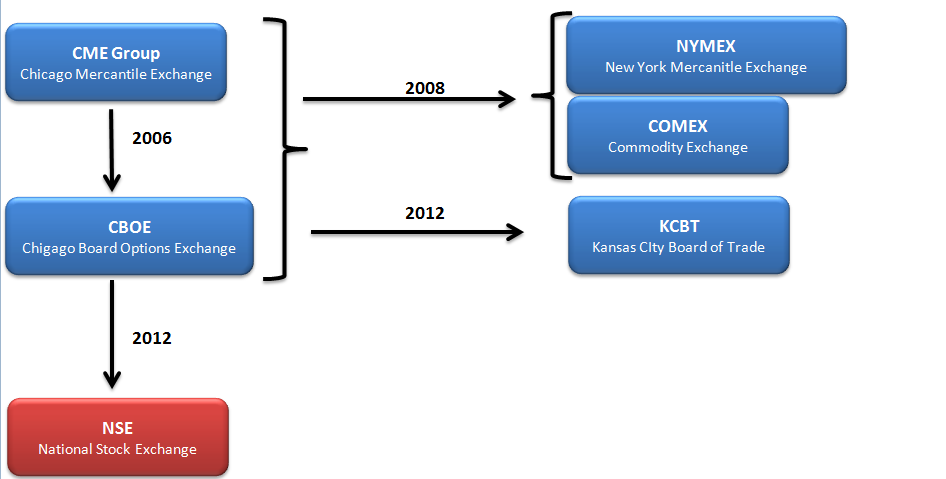
Monte Carlo Simulations
Monte Carlo Simulations — Geometric Brownian Motion
Monte Carlo Simulations is a useful method used in quantitative finance and mathematical finance to gauge and analyze financial instruments, asset portfolios and investments. The method is based on simulating the various uncertainty sources that can affect the financial value in a certain time horizon.
The final result is obtained by repeating the simulations N times and then determining either their average value or other statistics on the wide range of resultant outcomes. The simulations are done by the introduction of random variables into the process, in finance the most used process is given by Geometric Brownian Motions where the random variable follows a Wiener process, obtained as specific case of Markovian stochastic process.
In finance, Monte Carlo Simulations are a useful tool used to solve many financial aspects, such as for instance:
- Corporate Finance: in Corporate Finance, in case of financial projections, Monte Carlo Methods are used by financial analysts who wish to construct stochastic or probabilistic financial models as opposed to the traditional static and deterministic models. Within the simulations the future cash flow components are impacted by a random uncertainty and incorporating any correlation between these, mathematically reflecting their random characteristics. Usually the results are combined in a histogram of NPV (Net Present Value) given by discounting the future cash flow to time 0. Thus it is possible to gauge and analyze the average NPV of the potential investment as well as its volatility and other statistics sensitivities.
- Option Pricing: in valuing a stock option (or a basket option), the simulation made from the process generates several thousand possible random price paths for the underlying share in a certain time horizon. At the end of each simulated path we value the option’s pay off given the exercise value, then all these gauged payoffs are averaged and then discounted to today, the discounted result is the value of the option today (i.e. fair price).
- Fix Income: to value fixed income instruments and interest rate derivatives the underlying source of uncertainty which is simulated is the short rate, in this case the stochastic processes on short rates was created by Vasicek and CIR (Cox, Ingersoll and Ross). As we know, the bond prices is heavily dependent from the rates, so the best way to value the future bond prices is to model the future interest rates.
- Risk Management: in recent years, the seemingly large daily price movements in high- tech stocks have generated discussions on market risk and margin setting for financial institutions. To manage this risk the regulatory agencies introduced the value at risk (VaR). The VaR has become one of the most important risk margins for all the financial institutions. Monte Carlo Simulations is a very useful tool to value and manage the potential loss given a portfolio of assets in a certain time horizon.
Stochastic Processes
Each variable that change its value randomly during a certain time follows a stochastic process. The Markovian process is a specific stochastic process where just the current value of the variable is useful to predict the future. In this specific process what happened to the variable in the past is irrelevant.
So, if for instance a share price follows a Markov process, its future value does not depend by the past price values, that is why its future price (path) is completely independent by its past prices (past path).
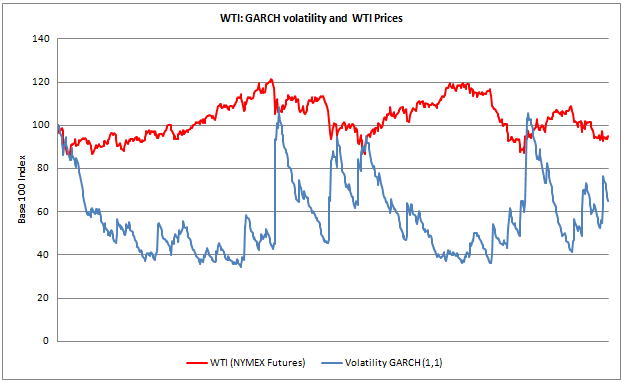
These processes can be used to value the future path of a share into a Geometric Brownian Motion. The underlying hypothesis is the future path of the share is given by a deterministic value (the average share returns) that is the expected annual return plus a random variable that gives the so called random walk to the share price, the random variable gives the volatility (uncertainty) to the process.
Using the Geometric Brownian Model and the Monte Carlo simulations is possible to value the forecast assets distribution in a future time T. It is possible to use the Wiener process to simulate a stochastic process in a discrete time for the share prices.
Where we value the variables as:
The process is driven by the hypothesis of normal distribution of returns (log- normal distribution of prices). Thus the random variable is obtained from an inverse probabilistic process of a standardized normal distribution with mean 0 and variance 1.