Introduction to Time Series Modeling
Post on: 16 Март, 2015 No Comment
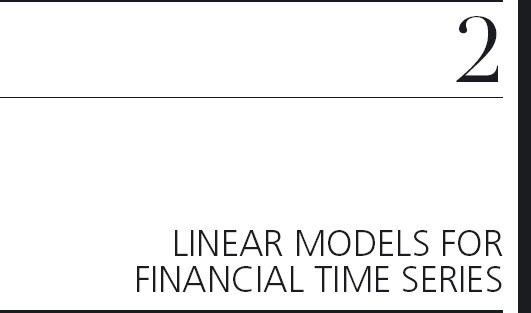
A time series is a sequence of data points. Typically the data points are arranged according to the time at which they are collected, though certain other kinds of sequential data can be amenable to time series analysis. Usually the data are collected at uniform time intervals. The characteristic property of time series is that the data are not generated independently, and the nature of the dependence is of great interest. Often the data embody trends and have cyclic components. These too are of significant interest. Time series arise in essentially any discipline in which sequences of numerical data are collected. Applications range from medical (electrocardiograms and electroencephalograms) to business and economics (stock prices and interest rates) to meteorology (daily maximum temperatures and precipitation).
Time series analysis consists of methods for exploring sequential data in order to extract meaningful statistics and identify significant characteristics. The goals of the analysis generally include at least one of the following: development of models to understand the stochastic processes underlying the data, prediction of future events based on past events, application of control to influence future values of the data, and identification of persistent signals present in the data.
The current book focuses on the modeling of time series. In roughly the first half of the text, the author introduces the basic ideas and primary tools of time series analysis. A significant part of this is the treatment of autoregressive models, such as ARMA (autoregressive moving average) that represent a time series value as a linear combination of past values and white noise. The challenge with these is first to estimate the order of the AR model (how many past values to use), then to estimate the unknown coefficients. The author describes several approaches, including the standard one that uses the Yule-Walker method and Levinsons algorithm.
Although both time-domain and frequency-domain approaches to time series modeling are commonly used, the author concentrates on the time domain. Just one chapter discusses spectral analysis and periodiograms. What distinguishes this book from comparable introductory texts is the use of state space modeling. Along with this come a number of valuable tools for recursive filtering and smoothing including the Kalman filter, as well as non-Gaussian and sequential Monte Carlo filters.
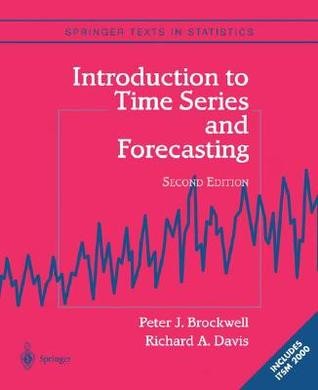
As an introduction to the subject, this book has an excessively theoretical emphasis. Although the author provides many graphs of interesting time series from a variety of sources, there are very few examples of computation using those data. Most students learn time series analysis best by applying the techniques theyre learning to real data sets, but they have very little opportunity to see that done here. By contrast, Time Series Analysis: Forecasting and Control by Box and Jenkins, a classic text in this field, uses a collection of standard time series data to great effect in order to illustrate individual analysis methods. Another important practical issue is that common errors in modeling and analyzing time series are not considered. Yet that is something that students need to see.
The current book is a useful reference for the application of state space modeling to time series. As an introductory textbook, however, it leaves much to be desired.
Bill Satzer (wjsatzer@mmm.com ) is a senior intellectual property scientist at 3M Company, having previously been a lab manager at 3M for composites and electromagnetic materials. His training is in dynamical systems and particularly celestial mechanics; his current interests are broadly in applied mathematics and the teaching of mathematics.