Hypothesis Testing in Finance Concept & Examples
Post on: 12 Июнь, 2015 No Comment
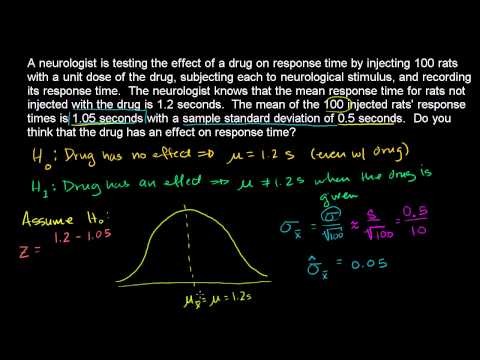
Your investment advisor proposes you a monthly income investment scheme which promises a variable return each month. You will invest in it only if you are assured of an average of a $180 monthly income. Your advisor also tells you that for the past 300 months, the scheme had returns with an average value of $190 and standard deviation of $75. Should you invest in this scheme?
Hypothesis testing comes to the aid for such decision making.
This article assumes readers’ familiarity with concepts of a normal distribution table, formula, p-value and related basics of statistics.
For more on practical applications of data to determine risk, see 5 Ways to Measure Mutual Fund Risk .
Hypothesis Testing (or significance testing) is a mathematical model for testing a claim, idea or hypothesis about a parameter of interest in a given population set, using data measured in a sample set. Calculations are performed on selected samples to gather more decisive information about characteristics of the entire population, which enables a systematic way to test claims or ideas about the entire dataset.
Here is a simple example: (A) A school principal reports that students in her school score an average of 7 out of 10 in exams. To test this “hypothesis”, we record marks of say 30 students (sample) from the entire student population of the school (say 300) and calculate the mean of that sample. We can then compare the (calculated) sample mean to the (reported) population mean and attempt to confirm the hypothesis.
Another example: (B) The annual return of a particular mutual fund is 8%. Assume that mutual fund has been in existence for 20 years. We take a random sample of annual returns of the mutual fund for, say, five years (sample) and calculate its mean. We then compare the (calculated) sample mean to the (claimed) population mean to verify the hypothesis.
Different methodologies exist for hypothesis testing. The following four basic steps are involved:
Step 1: Define the hypothesis:
Usually the reported value (or the claim statistics) is stated as the hypothesis and presumed to be true. For the above examples, hypothesis will be:
- Example A: Students in the school score an average of 7 out 10 in exams
- Example B: Annual return of the mutual fund is 8% per annum
This stated description constitutes the “Null Hypothesis (H0 ) ” and is assumed to be true. Like a jury trial starts by assuming innocence of the suspect followed by determination whether the assumption is false. Similarly, hypothesis testing starts by stating and assuming the “Null Hypothesis ”, and then the process determines whether the assumption is likely to be true or false.
The important point to note is that we are testing the null hypothesis because there is an element of doubt about its validity. Whatever information that is against the stated null hypothesis is captured in the Alternative Hypothesis (H1 ). For the above examples, alternative hypothesis will be:
- Students score an average which is not equal to 7
- Annual return of the mutual fund is not equal to 8% per annum
In summary, Alternative hypothesis is a direct contradiction of the null hypothesis.
As in a trial, jury assumes suspect’s innocence (null hypothesis). The prosecutor has to prove otherwise (alternative). Similarly, the researcher has to prove that the null hypothesis is either true or false. If the prosecutor fails to prove the alternative hypothesis, the jury has to let go the suspect(basing the decision on null hypothesis). Similarly, if researcher fails to prove alternative hypothesis (or simply does nothing), then null hypothesis is assumed to be true.
Step 2: Set the decision criteria
The decision-making criteria have to be based on certain parameters of datasets and this is where the connection to normal distribution comes into the picture.
As per the standard statistics postulate about sampling distribution, “For any sample size n, the sampling distribution of X̅ is normal if the population X from which the sample is drawn is normally distributed.” Hence, the probabilities of all other possible sample means one could select are normally distributed.
For e.g. determine if the average daily return, of any stock listed on XYZ stock market, around New Year’s time is greater than 2%.
H0. Null Hypothesis: mean = 2%
H1. Alternative Hypothesis: mean > 2% (This is what we want to prove)
Take the sample (say of 50 stocks out of total 500) and compute the mean of sample.
For a normal distribution, 95% of the values lie within 2 standard deviations of the population mean. Hence, this normal distribution and central limit assumption for the sample dataset allows us to establish 5% as a significance level. It makes sense as under this assumption, there is less than a 5% probability (100-95) of getting outliers that are beyond 2 standard deviations from the population mean. Depending upon the nature of datasets, other significance levels can be taken at 1%, 5% or 10%. For financial calculations (including behavioral finance), 5% is the generally accepted limit. If we find any calculations that go beyond the usual 2 standard deviations, then we have a strong case of outliers to reject the null hypothesis. Standard deviations are extremely important to understanding statistical data. Learn more about them by watching Investopedia’s video on Standard deviations .
Graphically, it is represented as follows:
In the above example, if the mean of the sample is much larger than 2% (say 3.5%), then we reject the null hypothesis. The alternative hypothesis (mean >2%) is accepted, which confirms that the average daily return of the stocks are indeed above 2%.
However, if the mean of sample is not likely to be significantly greater than 2% (and remain at say around 2.2%), then we CANNOT reject the null hypothesis. The challenge comes on how to decide on such close range cases. To make a conclusion from selected samples and results, a level of significance is to be determined, which enables a conclusion to be made about the null hypothesis. The alternative hypothesis enables establishing the level of significance or the critical value” concept for deciding on such close range cases. As per the standard definition. “A critical value is a cutoff value that defines the boundaries beyond which less than 5% of sample means can be obtained if the null hypothesis is true. Sample means obtained beyond a critical value will result in a decision to reject the null hypothesis”. In the above example, if we have defined the critical value as 2.1%, and the calculated mean comes to 2.2%, then we reject the null hypothesis. A critical value establishes a clear demarcation about acceptance or rejection.
More examples to follow – First, though, let’s look at some more key steps and concepts.
Step 3: Calculate the test statistic:
This step involves calculating the required figure(s), known as test statistics (like mean. z-score. p-value. etc.), for the selected sample. The various values to be calculated are covered in a later section with examples.
Step 4: Make conclusions about the hypothesis
With the computed value(s), decide on the null hypothesis. If the probability of getting a sample mean is less than 5%, then the conclusion is to reject the null hypothesis. Otherwise, accept and retain the null hypothesis.
Types of Errors in decision making:
There can be four possible outcomes in sample-based decision making, with regards to the correct applicability to entire population: