Forecasting time variation
Post on: 13 Май, 2015 No Comment
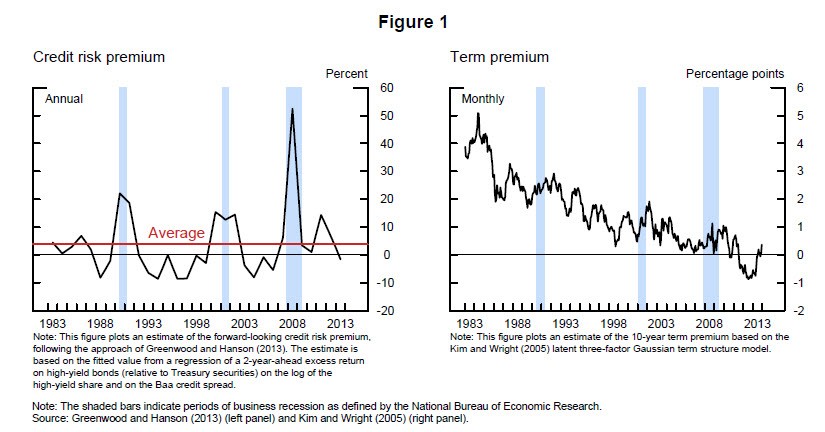
The finance essay below has been submitted to us by a student in order to help you with your studies.
Abstract
This paper seeks to evaluate the out of sample performance of contemporary beta forecasting models in predicting systematic risk of United Kingdom energy stocks. The forecasting models utilised are the Naive, Vasicek and the Blume rolling and constant methodologies. The relative out of sample performances of these models are tested on daily and weekly UK energy stock data across 7 different estimation periods. This research finally utilises symmetric and asymmetric error statistics to determine the most accurate forecasting methodology.
Introduction
Rational investors in every asset class are concerned with the risk and return profile which their investments yield, whereby higher investment risk should equivalently yield higher returns. This risk- return framework has been empirically identified as the appropriate benchmark to examine the performance of each investment. The Capital Asset Pricing Model (CAPM), first developed by Sharpe (1964) and Lintner (1965), offers a quantitative means of formulating the required returns any investment must generate relative to its systematic risk, as represented by the Beta coefficient within the model.
The beta coefficient, developed from the CAPM model, is mathematically defined as the covariance between an individual security and the market index, divided by the market index variance. It is also known as the slope coefficient and can be obtained by ordinary least squares (OLS) regression between the individual security rate of return and the market index rate of return. The OLS procedure minimises the squared errors between the observed market index and individual security rate of returns and the linear fitted regression line. As a result, the beta coefficient represents only the undiversifiable risk of an individual security in a stock market.
However, as the recent financial crisis which began in 2007 has further revealed, the systematic risk for most asset classes may evolve over time and this may result in mispricing of various assets and subsequent losses, if the time evolution of beta is not taken into account. As a result, the utilisation of historical betas may not be appropriate in forecasting the systematic risk for various assets. Jaganathan and Wang (1993) empirically showed, utilising 28 year data, that when betas are allowed to be time varying and human capital is included in wealth, the CAPM adequately measures the systematic variation in stock average returns. Fernandez (2004) also empirically showed that historical betas demonstrate instability across time in his research on 3,813 companies from the New York Stock Exchange and NASDAQ.
Thus, in order to develop robust Beta estimates for CAPM models, researchers have developed alternative forecasting methodologies which account for the time variation. Blume (1971) observed that historical betas for individual securities are unable to accurately forecast future betas, given a 36% correlation coefficient between the historical betas and the realised betas. Blume, contended that a general autoregressive model of the historical betas would provide a better estimation of future betas than the naive utility of historical betas.
Vasicek (1973) proposed an alternative forecasting methodology built on the Bayesian decision theorem. Vasicek methodology is built on the empirical basis that stock beta coefficients are influenced by the central limit theorem whereby stocks converge to the unity over time. Blume (1975) empirically demonstrated this beta mean regression tendency with four portfolios consisting of 100 stocks each which were obtained from the New York Stock Exchange (NYSE), whereby each portfolio regressed towards unity across each time period. Blume further contended that the observed regression tendency of beta to unity is caused by companies exhibiting less risky profiles across time. This is because companies either adopt less risky projects across time or the existing extreme risk of projects declines over time.
The Vasicek method implies that, in consideration of the cross sectional prior distribution of stock betas, the appropriate beta estimate is a weighted average between the stocks historical beta and the stock market mean beta in the previous period. The higher the variance of errors of the individual security, the higher the weighting assigned to the stock market mean beta. Also, vice versa, the lower the variance of errors of the individual security, the higher the weighting assigned to the individual security beta. Vasicek considered his Bayesian estimation technique to be superior to contemporary classical sampling methods as the method minimises misestimation losses. This is because the Bayesian theory utilises all available information on the parameter distribution. Also, according to Vasicek, another advantage of his Bayesian estimation technique is that it weights expected losses by a prior distribution of the parameters, which is especially relevant if the available information on prior distribution is large and comprises of a large number of stocks.
The Vasicek and Blume forecasting methodologies are the contemporary beta forecasting techniques risk measurement techniques utilised by finance professionals. This has resulted in a wide range of studies examining the relative efficiency of each method in accurately forecasting systematic risk of securities in different countries. Cloete, Jongh and De Wet (2002) utilised Vasicek estimators, combined with L-estimators, to forecast betas in the Johannesburg Stock Exchange (JSE) and observed that methodology produced significantly higher quality betas than naive betas, as measured by forecast error statistics. Gray et al (2009) observed that Vasicek estimators surpassed unadjusted betas as well as R-squared and t-stat filtered betas, in terms of beta forecasts for the stocks from the Australian Securities Exchange.
Cuoto and Duoque (2000) conducted their study on the thinly traded Portuguese stock market and observed that Vasicek and Blume betas outperformed unadjusted naive betas. However, they observed that neither the Blume nor Vasicek method outperformed each other in forecasting stock market beta estimates. Elton, Gruber and Urich (1978) observed in their study for 100 firms that both Vasicek and Blume forecast betas outperformed the naive betas. The study also noted that the Blume method provided better forecasts, across the 10 year sample period, when the average forecast for each technique was not adjusted to possess the same value. In contrast, when the average forecast for each technique was adjusted to possess the same value, Elton, Gruber and Urich noted that the Vasicek method provided better beta forecast values than the Blume method.
Klemkosky and Martin (1975) observed that both Vasicek and Blume betas outperformed unadjusted betas across the time periods considered in the study. However, the Blume beta forecast provided superior beta forecasts across three time period while the Vasicek method performed better forecast across two time periods. Lawrence and Kamin (1985) observed in their study on the NYSE stock market for a 558 month period that Blume forecast methodology generally outperformed the Vasicek method across the evaluation period. Eubank and Zumwalt (1979) utilised returns data from the CRSP to conclude that adjusted betas surpassed unadjusted betas in terms of forecasting betas. The use of adjusted betas was more useful for single securities, as against portfolios, as well as short beta estimation periods. In addition, Eubank and Zumwalt observed that the Blume method significantly outperformed the Vasicek method in short estimation periods, although there was no difference between both methods for longer estimation periods.
The results of the above empirical studies revealed that adjusted betas are useful means of forecasting systematic risk, especially as most of the adjusted stock betas outperformed unadjusted stock betas in the stock markets examined. Thus, finance professionals stand to gain from utilising adjusted betas to forecast systematic risk for their portfolios, given the global credit crisis which destabilised most financial markets and has presented finance professionals with the problem of forecasting the appropriate beta for the stocks.
This paper attempts to determine the most appropriate beta forecasting methodology for oil and gas stocks in the London Stock Exchang (LSE). The forecasting methodologies analysed are the naïve method, the Blume rolling and constant method and the Vasicek method. The second section of the paper presents the data and methodology. Third section summarizes the empirical findings and fourth section presents the conclusion
Data and Methodology
Data
The data utilised in this paper comprised of 19 oil and gas stocks traded on the London Stock Exchange Main market. These oil and gas stocks comprise solely of energy conglomerates which actively participate in both upstream and downstream activities. The observation period of the data collected is between September 19, 2001 and May 22, 2009. 15 of the stocks were not continuously traded during the observation period and were omitted from the study. These comprised of stocks which started trading within the period as well as thinly traded stocks. The market index utilised in the study is the FTSE All- Share index from the London Stock Exchange.
The study further utilises daily and weekly returns, within the observation period, to calculate the beta coefficients. The continuously compounded daily and weekly returns of each stock, as well as the stock index, are calculated as follows:
The daily and weekly beta coefficients for each stock are estimated through the standard
time series regressions:
where Rm,t denotes the market return at time t. Systematic risk for each stock is measured by bi..
The beta estimates are computed across 7 different estimation periods. The daily betas are estimated using bi-annual, annual and semi-annually windows while weekly betas are estimated across monthly, semi-annually, annual and bi-annual estimation windows. The non-overlapping periods are used to calculate the return and beta series.
Forecasting Techniques
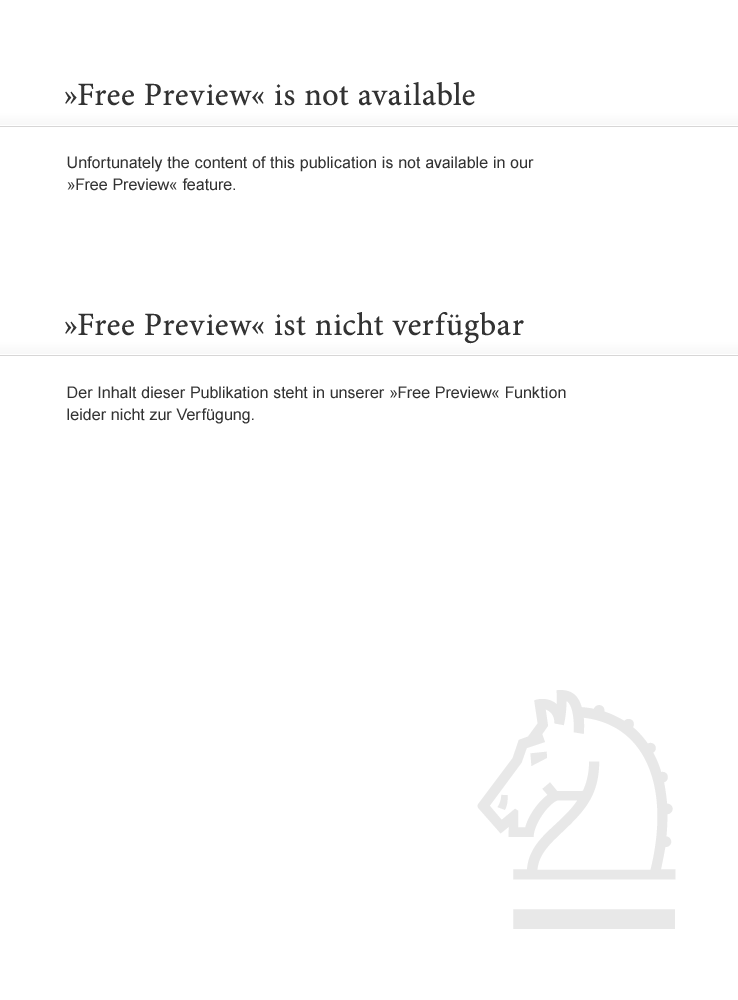
As highlighted in the first section, the study utilises three techniques to derive the beta forecasts namely: the historical mean (HM) method, the Blume method and the Vasicek method. The HM approach is a naïve technique. It forecasts the beta by simply using historical data without any adjustment. It may thus be considered as an average of previous historical betas. Mathematically;
where bi,t is the forecast beta for stock i for period t and is calculated as the average of all historical betas available up to period t-1.
The second alternative forecasting methodology utilised in this study is the Blume Method which is calculated by the following regression equation:
The Blume method is a first-order autoregression where the beta coefficients of the last period are regressed on one period-lagged estimated betas (Eq. 4). the estimated parameters a^? and ?^? are used for forecasting next period’s beta (Eq. 5). As mentioned in the first section, this study utilises the Blume constant and rolling methodologies. The Blume constant method utilises constant regression parameters, estimated from the first and second periods, to forecast future beta. Thus, the parameter estimates of a^? and ?^? are fixed. However, the second method utilises evolving regression parameters to forecast future beta and is called a rolling regression. This Blume forecast methodology permits the parameters a^? and ?^? to change across time. The Blume method theoretically states that future beta estimates will approach unity over time.
The Vasicek method is a Bayesian estimation technique which utilises the cross sectional prior information on the beta distribution to forecast betas. The Vasicek method is benchmarked on the empirical findings of Blume (1971), who observed that stock betas tend to evolve towards unity over time. In the this approach, the forecast beta is the weighted average of historical beta observed in the time period t-1 and the average beta which is around unity. Let ß_(u,t-1) represent average beta coefficient for k stocks in period t-1, s_(? ß?_(u,t-1))^2 be the variance of beta coefficients for k stocks in period t-1, s_(? ß?_(i,t-1))^2be the standard error of beta coefficient for stock i in period t-1, e_( i,t)^2 and be the estimation error.
Under above definitions, Vasicek’s (1973) beta forecast is given by
From the above equation (9), the weight given to average beta increases if the standard error of the estimated increases, which means that the weight of average beta is increased when the quality of estimated beta decreases.
Forecast Evaluation
We first employ four commonly used symmetric error statistics: the mean error (ME), the mean absolute error (MAE), the mean squared error (MSE), and the mean absolute percentage error (MAPE). Forecast error for period m is the difference between forecast beta (bf,m) and actual beta (ba,m) for each model. These conventional error statistics for each individual stock, i are computed as follows:
The symmetric error statistics give an equal weight to under-and-over-predictions of beta of similar magnitude. However, under/over-prediction of systematic risk is important for traders who may possess long and short margin positions in securities. We employ two asymmetric performance criteria, namely the mean mixed error (MME) statistics. The MME(U) and MME(O) identify and penalize underpredictions and overpredictions in betas. These non-conventional error statistics for each individual stock, i, are computed as follows:
Also, Km, Lm and Mm are dummy variables such that Km = 1 if bf,m > ba,m and 0 otherwise; Lm = 1 if bf,m 1, and 0 otherwise.
Similarly, Nm = 1 if bf,m > ba,m and |bf,m — ba,m| ba,m and |bf,m — ba,m| > 1, and 0 otherwise; and Pm = 1 if bf,m