Financial Forecasting The Bayesian Method_2
Post on: 13 Май, 2015 No Comment
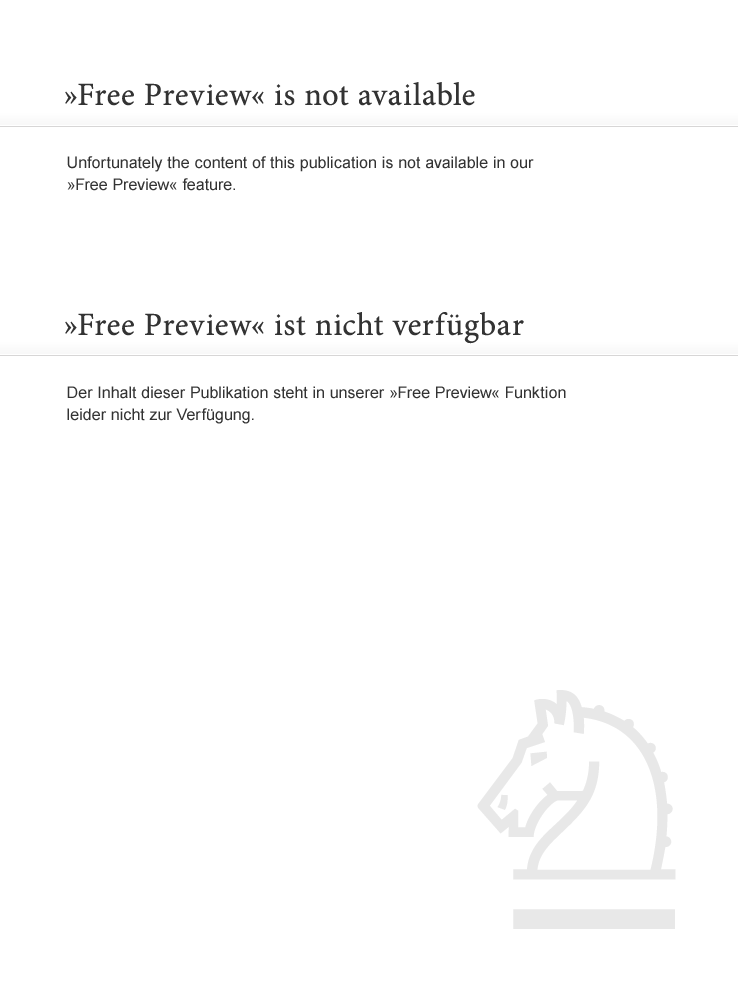
Despite many of the negatives that we hear about DCF-based stock valuation these days, it is still a mainstream method for stock valuation as part of fundamental equity research. In his 1992 Berkshire Hathaway (BRK.A) annual report concerning the DCF stock valuation method, Warren Buffett stated In the Theory of Investment Value, written over 50 years ago, John Burr Williams set forth the equation for value, which we condense here: The value of any stock, bond or business today is determined by the cash inflows and outflows discounted at an appropriate interest rate that can be expected to occur during the remaining life of the asset. Many of the popular stock market report bands equity analysis resources that retail value-investors rely on utilize this stock valuation method. This article will examine the strengths and weaknesses of DCF-based intrinsic value calculations and why it is importing for value investing.
Lets review the main weaknesses of DCF-based stock valuation.
The first is that it requires us to predict cash flows or earnings long into the future. Data shows that most equity analysts cannot predict next-years earnings accurately. On a macroeconomic level, the experts have a terrible track record in predicting jobless claims, the year-end S&P, or GDP. This is no different when it comes to projecting the future cash flow of a business when picking stocks. We have to admit to ourselves that we have tremendous limitations in the ability to forecast future cash flows based on past results and recognize that a small error in the forecast can result in a large difference in the stock valuation.
The second challenge is determining the appropriate discount rate. What is the discount rate? Should we dust off our college or graduate school notebook and look at the CAPM, which calculates the discount rate as the risk-free rate plus the risk premium?
Well, since this I learned this formula from the same guy (by business school finance professor) that convinced me as a 22-year old, wet-behind the ears student that markets are efficient, I am skeptical. The most famous value investor Warren Buffetts public comments about the issue have evolved as he has stated that he uses the long term US treasury rate since he tries to deal with things about which we are quite certain but reminded us in 1994 that In a world of 7% long-term bond rates, wed certainly want to think we were discounting the after-tax stream of cash at a rate of at least 10%. But that will depend on the certainty that we feel about the business. The more certain we feel about the business, the closer were willing to play. Im inclined to take these seemingly contradictory guidelines from Buffett and from there derive a reasonable estimate of the discount rate as part of my stock research. With the September 1, 2011 30-Yr treasury yield at 3.51%, we must think that our discount rate for large cap stocks is closer to 10% than to the risk-free rate.
Finally, the problem with determining a feasible growth rate is that a DCF will simulate the growth rate to be eternal, and we know that no business can sustain an above-average growth rate in perpetuity.
Data mining is the retrieving of hidden information from data using algorithms. Data mining helps to extract useful information from great masses of data, which can be used for making practical interpretations for business decision-making. It is basically a technical and mathematical process that involves the use of software and specially designed programs. Data mining is thus also known as Knowledge Discovery in Databases (KDD) since it involves searching for implicit information in large databases. The main kinds of data mining software are: clustering and segmentation software, statistical analysis software, text analysis, mining and information retrieval software and visualization software.
Data mining is gaining a lot of importance because of its vast applicability. It is being used increasingly in business applications for understanding and then predicting valuable information, like customer buying behavior and buying trends, profiles of customers, industry analysis, etc. It is basically an extension of some statistical methods like regression. However, the use of some advanced technologies makes it a decision making tool as well. Some advanced data mining tools can perform database integration, automated model scoring, exporting models to other applications, business templates, incorporating financial information, computing target columns, and more.
Some of the main applications of data mining are in direct marketing, e-commerce, customer relationship management, healthcare, the oil and gas industry, scientific tests, genetics, telecommunications, financial services and utilities. The different kinds of data are: text mining, web mining, social networks data mining, relational databases, pictorial data mining, audio data mining and video data mining.
Some of the most popular data mining tools are: decision trees, information gain, probability, probability density functions, Gaussians, maximum likelihood estimation, Gaussian Baves classification, cross-validation, neural networks, instance-based learning /case-based/ memory-based/non-parametric, regression algorithms, Bayesian networks, Gaussian mixture models, K-Means and hierarchical clustering, Markov models, support vector machines.
Some popular data mining software includes: Connexor Machines, Copernic Summarizer, Corpora, DocMINER, DolphinSearch, dtSearch, DS Dataset, Enkata, Entrieva, Files Search Assistant, FreeText Software Technologies, Intellexer, Insightful InFact, Inxight, ISYS:desktop, Klarity (part of Intology tools), Leximancer, Lextek Onix Toolkit, Lextek Profiling Engine.
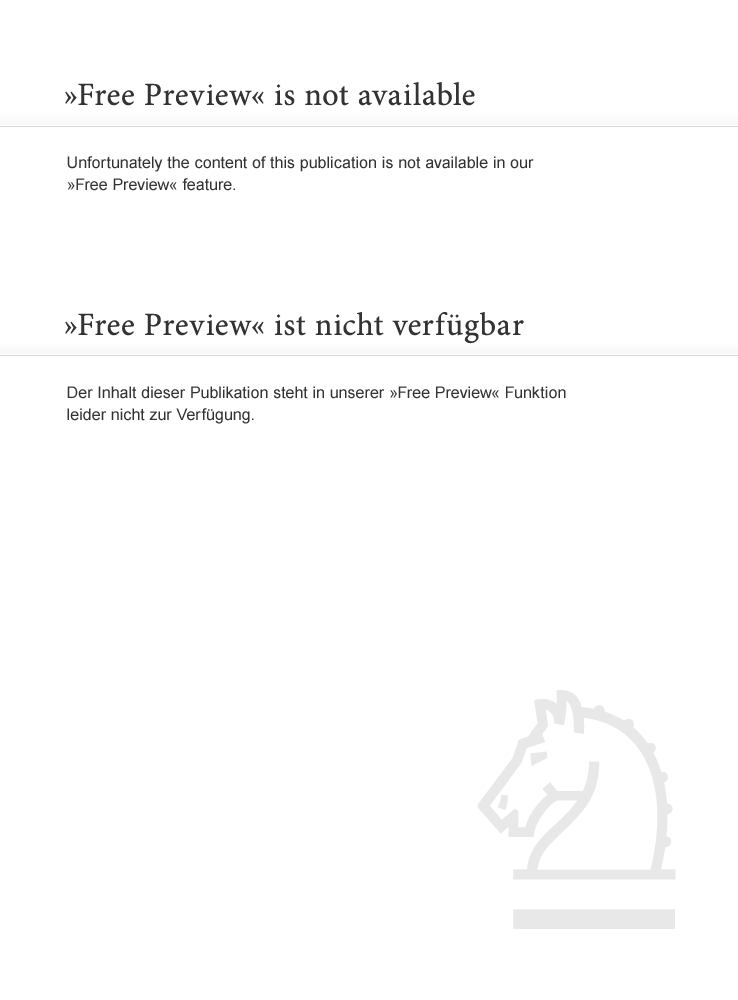
Many companies continue to struggle with poor forecast numbers even after implementing Demantra Demand Management (DM) or Advanced Forecasting (AFDM) modules. The promise of a more accurate forecast post- Demantra implementation remains unfulfilled as demand planners are forced to resort to manual overrides for large number of records leading to a lengthy forecast review process causing significant delays in the overall demand management process.
The most common reason for poor forecast numbers generated by Demantra is the engine not being tuned to take into account client specific data set requirements.
Ideally, during the implementation, a lot of time needs to be spent on analyzing the data set and configuring the engine parameters while keeping the client specific data model in mind. Unfortunately, it has been observed that Demantra engine tuning exercise is accorded least priority and often left for until after go-live period.
Many a times during the Demantra implementation, both the consultants as well as the business users are so focused on catering to requirements related to worksheets, series, workflows etc. that they tend to take better forecast accuracy out of Demantra for granted and ignore to do the due diligence to tune the Demantra engine.
Also, since Demantra engine tuning being a specialized skill; it requires indepth understanding of various factors that contribute towards better forecast accuracy and understanding of various engine parameters that need to be setup for better results.
Though this is a specialized area and should be performed by highly qualified and experienced consultants but, users of Demantra and demand planners should also be familiar with the different factors that can influence the forecast accuracy.