Data Mining_1
Post on: 29 Апрель, 2015 No Comment
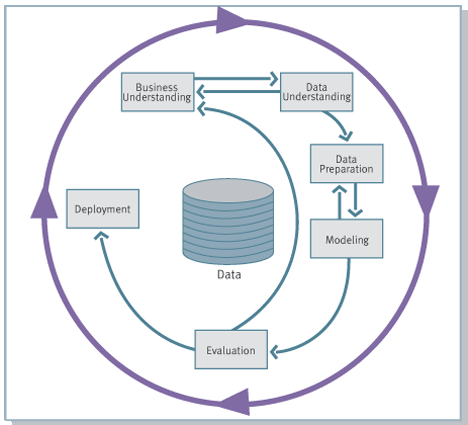
To Err Is Human. To Really Screw Up, You Need a Computer.
— Popular Campus T-shirt, circa 1980
Stupid Data Miner Tricks in Quantitative Finance
This chapter started out over 10 years ago as a set of joke slides showing silly, spurious correlations. Originally, my quantitative equity research group planned on deliberately abusing the genetic algorithm (see Chapter 8 on evolutionary computation) to find the wackiest relationships, but as it turned out, we didnt need to get that fancy. Just looking at enough data using plain vanilla regression would more than suffice.
We uncovered utterly meaningless but statistically appealing relationships between the stock market and third world dairy products and livestock populations which have been cited often in Business Week. The Wall Street Journal. the book A Mathematician Plays the Stock Marke t,(1) and many others. Students from Bill Sharpes classes at Stanford seem to be familiar with them. This was expanded, to have some actual content about data mining, and reissued as an academic working paper in 2001. Occasional requests for this arrive from distant corners of the world. An updated version appeared in the Journal of Investing in 2007.* [*This article originally appeared in the Spring 2007 issue of the Journal of Investing. as Stupid Data Miner Tricks: Overfitting the S&P 500 . It is reprinted in Nerds on Wall Street with permission.
Without taking too much of a hatchet to the original, the advice here is still valuable perhaps more so now that there is so much more data to mine. Monthly data arrives as one data point, once a month. Its hard to avoid data mining sins if you look twice. Ticks, quotes, and executions arrive in millions per minute, and many of the practices that fail the statistical sniff tests for low-frequency data can now be used responsibly. New frontiers in data mining have been opened up by the availability of vast amounts of textual information. Whatever raw material you choose, fooling yourself remains an occupational hazard in quantitative investing. The market has only one past, and constantly revisiting it until you find that magic formula for untold wealth will eventually produce something that looks great, in the past. A fine longer exposition of these ideas is found in Nassim Talebs book, Fooled by Randomness: The Hidden Role of Chance in Markets and Life (W.W. Norton, 2001).
Your Mama Is a Data Miner Getting a Bad Name in Computational Finance
It wasnt too long ago that calling someone a data miner was a very bad thing. You could start a fist fight at a convention of statisticians with this kind of talk. It meant that you were finding the analytical equivalent of the bunnies in the clouds, poring over data until you found something. Everyone knew that if you did enough poring, you were bound to find that bunny sooner or later, but it was no more real than the one that blows over the horizon.
Data mining is a small industry, with entire companies and academic conferences devoted to it. The phrase no longer elicits as many invitations to step into the parking lot as it used to. Whats going on? These new data mining people are not fools. Sometimes data mining makes sense, and sometimes it doesnt.
The new data miners pore over large, diffuse sets of raw data trying to discern patterns that would otherwise go undetected. This can be a good thing. Suppose a big copier company has thousands of service locations all over the world. It wouldnt be unusual for any one of them to see a particular broken component from any particular copier. These gadgets do fail. But if all of a sudden the same type of part starts showing up in the repair shops at 10 times its usual rate, that would be an indication of a manufacturing problem that could be corrected at the factory. This is a good (and real) example of how data mining can work well, when it is applied to extracting a simple pattern from a large data set. Thats the positive side of data mining. But theres an evil twin.
The dark side of investment data mining techniques
The dark side of data mining is to pick and choose from a large set of data to try to explain a small one. Evil data miners often specialized in explaining financial data, especially the U.S. stock market. Heres a nice example: We often hear that the results of the Super Bowl in January will predict whether the stock market will go up or down for that year. If the National Football Conference (NFC) wins, the market goes up; otherwise, it takes a dive. What has happened over the past 30 years? Most of the time, the NFC has won the Super Bowl and the market has gone up. Does it mean anything? Nope. We see similar claims for hemlines, and even the phases of the moon. (2)
When data mining techniques are used to scour a vast selection of data to explain a small piece of financial market history, the results are often ridiculous. These ridiculous results fall into two categories: those that are taken seriously, and those that are regarded as totally bogus. Human nature being what it is, people often differ on what falls into which category.
The example in this paper is intended as a blatant instance of totally bogus application of data mining in finance. My quantitative investment management equity research group first did this several years ago to make the point about the need to be aware of the risks of data mining in quantitative investing. In total disregard of common sense, we showed the strong statistical association between the annual changes in the S & P 500 index and butter production in Bangladesh, along with other farm products. Reporters picked up on it, and it has found its way into the curriculum at the Stanford Business School and elsewhere. We never published it, since it was supposed to be a joke. With all the requests for the nonexistent publication, and the graying out of many generations of copies of copies of the charts, it seemed to be time to write it up for real. So here it is. Mark Twain (or Disraeli, or both) spoke of lies, damn lies, and statistics. In this paper, we offer all three.
Strip Mining the S & P 500 with Statistical Finance Techniques
Regression is the main statistical technique used to quantify the relationship between two or more variables.(3) It was invented by Adrien-Marie Legendre in 1805. A regression analysis would show a positive relationship between height and weight, for example. If we threw in waistline along with height, we’d get an even better regression to predict weight.
>>>>>> READ MORE HERE < <<<<<<
1. John Allen Paulos, A Mathematician Plays the Stock Market (New York: Basic Books, 2003).
2. It gets much wackier than this. A man named Norman Bloom, no doubt a champion of all data miners, went beyond trying to predict the stock market. Instead, he used the stock market, along with baseball scores, particularly those involving the New York Yankees, to “read the mind of God.” I offer a small sample of Bloom, in the original punctuation and spelling, here: “The instrument God has shaped to brig proof he has the power to shape the physical actions of mankind — is organized athletics, and particularly baseball. the second instrument shaped by the one God, as the means to bring proof he is the one God concerned with the mental and business aspects of mankind and his civilization is the stock market — and particularly the greatest and most famous of all these — i.e. the New York Stock Exchange.” Mr. Bloom’s work was brought to my attention by Ron Kahn of Barclays Global Investing. Bloom himself did not publish in any of the usual channels, but seekers of secondary truth can consult “God and Norman Bloom” by Carl Sagan, in American Scholar (Autumn 1977), p. 462.
3. There are many good texts covering the subject. For a less technical explanation, see The Cartoon Guide to Statistics by Larry Gonnick and Woolcott Smith (New York: HarperCollins, 1993).
4. Stephen M. Stigler, The History of Statistics: The Measure of Uncertainty before 1900 (Cambridge, MA: Belknap Press, 1986). This invention is also often attributed to Francis Galton, never to Disraeli or Mark Twain.
5. See John Freeman, “Behind the Smoke and Mirrors: Gauging the Integrity of Investment Simulations,” Financial Analysts Journal 48, no. 6 (November – December 1992): 26 – 31.
6. This question is addressed in “A Reality Check for Data Snooping ” by Hal White, UCSD Econometrics working paper, University of California at San Diego, May 1997.
7. This was the actual ad copy for a neural net system, InvestN 32, from Race Com. which was promoted heavily in Technical Analysis of Stocks & Commodities magazine, often a hotbed of data mining.
8. There are several alternatives in forming random data to be used for forecasting. A shuffling in time of the real data preserves the distribution of the original data, but loses many time series properties. A series of good old machine generated random numbers, matched to the mean, standard deviation, and higher moments of the original data
do the same thing. A more elaborate random data generator is needed if you want preserve time series properties such as serial correlation and mean reversion.
papers.ssrn.com/sol3/papers.cfm?abstract_id=1045281 .