Big Data Gets Bigger Now Google Trends Can Predict The Market
Post on: 15 Июль, 2015 No Comment
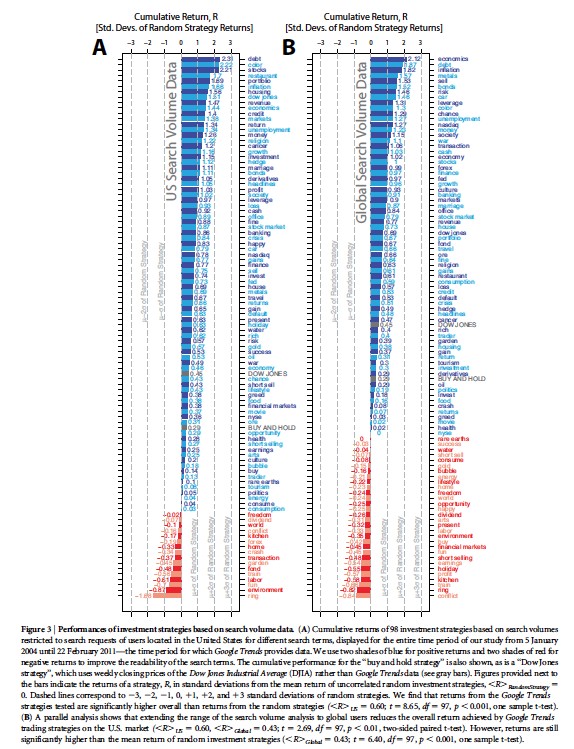
Yesterday three economists, (Tobias Preis of Warwick Business School in the U.K. Helen Susannah Moat of University College London, and H. Eugene Stanley of Boston University) published an eye-opening paper that said Google Trends data was useful in predicting daily price moves in the Dow Jones industrial average, which consists of 30 stocks. Their research result.
An uptick in Google searches on finance terms reliably predicted a fall in stock prices.
“Debt” was the most reliable term for predicting market ups and downs, the researchers found. By going long when “debt” searches dropped and shorting the market when “debt” searches rose, the researchers were able to increase their hypothetical portfolio by 326 percent. (In comparison, a constant buy-and-hold strategy yielded just a 16 percent return.)
This was a 180-degree turnaround from earlier research, by Prof. Preiss. published back in 2010.
Back in 2010, he used Google Trends data and found the opposite conclusion:
“The Google data could not predict the weekly fluctuations in stock prices. However, the team found a strong correlation between Internet searches for a company’s name and its trade volume, the total number of times the stock changed hands over a given week. So, for example, if lots of people were searching for computer manufacturer IBM IBM one week, there would be a lot of trading of IBM stock the following week. But the Google data couldn’t predict its price, which is determined by the ratio of shares that are bought and sold.”
What happened? Are people revealing more of their investment intentions in their searches?
The clue may be in looking at changes in the nature of what’s reported on Google Trends. In a nutshell, the data is getting bigger, by getting finer, and faster. We can see this by looking at two other papers, co-authored by Google’s chief economist, Hal Varian, author of the “if you only read one book on internet economics, read this one” Information Rules.
In the opening paragraph of an April 2009 paper, “Predicting the Present with Google Trends,” Hyunyoung Choi and Hal Varian describe Google Trends data:
“Economists, investors, and journalists avidly follow monthly government data releases on economic conditions. However, these reports are only available with a lag: the data for a given month is generally released about halfway through through the next month, and are typically revised several months later. Google Trends provides daily and weekly reports on the volume of queries related to various industries.
We hypothesize that this query data may be correlated with the current level of economic activity in given industries and thus may be helpful in predicting the subsequent data releases.”
All of the examples in the 2009 paper have either months or years on the time axis. They compared sales forecasts from standard seasonally adjusted and “momentum” models with similar models that also used the monthly Google Trends data. Here’s an example where they looked at sales of Ford cars. There were modest improvements in accuracy, a few percent, and it was about sales, not stock prices.
Interesting, but nothing to trade on.
Flash forward to 2011, in a newer version of the paper by Varian and Choi, with the same title, “Predicting the Present,” the same authors describe Google Trends differently
In this paper we examine Google Trends, which is a a real-time daily and weekly index of the volume of queries that users enter into Google. We have found that these query indices are often correlated with various economic indicators and may be helpful for short-term economic prediction.
The new paper is similar to the first version on the time scales they used for their econometric examples, but the desrcription of the data may contain the secret: Faster data is better and bigger data (real-time is both faster and better than lagged, and a spreadsheet with daily trends data is going to be about 30 times larger than one with monthly data)
Sorry guys, but when it comes to data for market predictions, size matters.