Application of Capital Asset Pricing (CAPM) and Arbitrage Pricing
Post on: 21 Сентябрь, 2015 No Comment
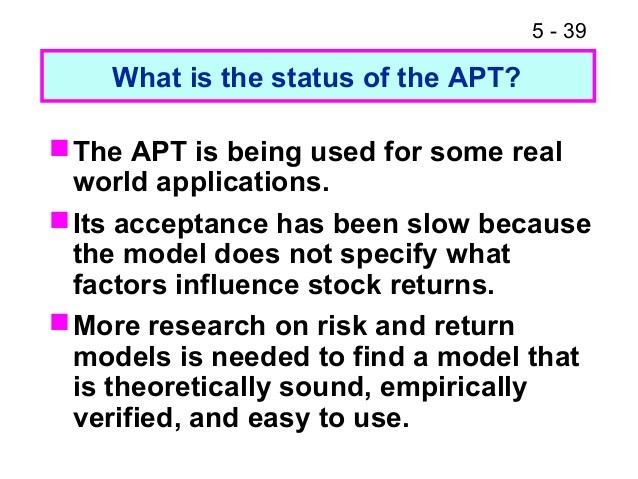
CONTENTS
Appendix 4
Appendix 5
Abstract
This paper examines the estimating and forecasting performance of the different and various Generalized Autoregressive Conditional Heteroscedasticity-GARCH’s models in relation to Capital Asste Pricing Model (CAPM) model. We apply the CAPM model with ordinary least squares (OLS) method to investigate if an ARCH (Autoregressive Conditional Heteroscedasticity) is presented and we are trying to decide and to analyze which GARCH model is the most appropriate and the best fitted for the financial time series that we have chosen. We apply CAPM model in the financial time series of the share prices of Technology-Software Sector in Athens Exchange stock market for the period January 1st of 2002 to October 30th of 2007 for the enterprises “Unibrain” “MLS Informatics” and “Dionic” respectively. from April 2nd of 2002 to 30th October of 2007 for the enterprise “Compucon”, from August 2nd of 2002 to 30th October of 2007 for the enterprise “Centric”, and finally from February 2nd of 2004 to 30th October of 2007 for the enterprise “Ilyda”. Additionally, we apply roiling regressions, where the full programming routines in EVIEWS and MATLAB are described detailed. We conclude that the slopecoefficient of CAPM model is not constant through the time period of rolling regressions we apply. In the final part we examine a simple Arbitrage Pricing Theory (APT) model.
Introduction
The aim of this paper is to present an estimation of the CAPM (Capital Asset Pricing Model), which was introduced by Sharpe (1964) and Linter (1965) where the mean and variance is supposed to be known. The difficulty of this model is that it can’t be estimated by the usual OLS method, because as is been referred the estimation of financial time series is nonlinear.
In section 1 we discuss the basic theoretical form of the CAPM model, we present the theoretical form of three major GARCH models which are the EGARCH, GJR and GARCH-M, and the basic characteristics of them as will examine the ARCH Component model.
In section 2 we present a brief statistic summary for the specific time series and to show if they are been characterized by leptokurtosis and to see the general situation and behavior of them and also will test for the stationarity of the specific time-series. Also we will test if there are ARCH effects in the specific time-series.
In section 3 we will follow the ARCH methodology for the CAPM model, for each price index separately, and to investigate if there are ARCH effects, which means if the CAPM models we analyze suffer from heteroscedasticity. If they are not then we estimate the specific model. Also we will test for the autocorrelation of the disturbance term. Finally we apply rolling-regressions to examine if the coefficientremains constant.
In section 4 followed by section 3 we apply the estimations of the GARCH models we mentioned before. So, if in section 3 we find that a CAPM model presents ARCH effects and as in the case of the CAPM-OLS estimation we apply rolling-regressions in the GARCH models.
In section 5 we discuss and present a simple APT model with cross-sectional regression as we will estimate with cross-sectional regression a CAPM model and we will make a comparison between them.
Section 1
As it was mentioned above the CAPM model was introduced in 1964 and four decades later is still widely used in applications, as the evaluation of managed portfolios performance (Fama and French, 2004). Sharpe pointed out that the only covariance that matters is that between the security’s return and that on the market portfolio. So the risk is known as the Beta coefficient which can be easily estimated with the simple linear regression (OLS) of the security’s return against that on the market portfolio (Booth, 1999).
The equation of the straight line has the theoretical form
illustration not visible in this excerpt
1 (1)
, where β is the Beta coefficient. Ifis the free-risk interest rate andis been substituted with the relation Abbildung in dieser Leseprobe nicht enthaltenthen the CAPM model has the theoretical form
illustration not visible in this excerpt
[1] (2)
And is constituted as [2]
illustration not visible in this excerpt
, where
illustration not visible in this excerpt
The higher the Beta is the higher must be the return of the share price (Elton, Gruber, Brown, Goetzmann, 2007, pp. 298-299). The CAPM model is nothing else than an application to the market portfolio of the relation between expected return and portfolio Beta that holds in any mean and variance efficient portfolio. But the problem is that is based on many unrealistic assumptions as one of them is the complete agreement (Fama and French, 2004 ). Also it would be better to write the CAPM model as
illustration not visible in this excerpt
(3)
, whereAbbildung in dieser Leseprobe nicht enthaltenis the disturbance term, because the estimation is been made econometrical and it would be a mistake to not mention the Abbildung in dieser Leseprobe nicht enthalten, because this is the “heart” of the econometrics.
First of all we will estimate this model with OLS to examine the relation of parameter Abbildung in dieser Leseprobe nicht enthaltenor with other words parameter Abbildung in dieser Leseprobe nicht enthaltengive us the constant proportion of the relation between the excess return of a risky asset and the excess return on the market portfolio (Cuthbertson, 1996, p.p. 24). So we will run the regression for each of the six stocks separately and one for the volume-weighted index of the specific sector. But the question is that Abbildung in dieser Leseprobe nicht enthaltenis it always constant? And should we consider the role of the disturbance termAbbildung in dieser Leseprobe nicht enthalten?
This will be examined with ARCH methodology and to test if there are ARCH effects. If there are then we will propose some GARCH models or else if there aren’t ARCH effects then we can say that we prefer the linear CAPM model.
The ARCH model as was originally proposed by Engle (1982) is
Abbildung in dieser Leseprobe nicht enthalten, where Abbildung in dieser Leseprobe nicht enthaltenis the variance Abbildung in dieser Leseprobe nicht enthaltenand Abbildung in dieser Leseprobe nicht enthaltenare square residuals Abbildung in dieser Leseprobe nicht enthalten, so the model can be rewritten as
illustration not visible in this excerpt
(4)
In the estimation of CAPM model there is the assumption that the variance is constant and so with other words the model does not present heteroskedasticity. With relation (3) we will test if there are ARCH effects and so on if the variance is constant or not. testing the hypothesis
illustration not visible in this excerpt
, where γ1. γ2, γ3…..and γk are the parameters of the regression
(5)
illustration not visible in this excerpt
When we conclude that there are ARCH effects then we will examine the CAPM with some GARCH models as the GARCH (1, 1). which process is much resemblance to the general ARMA process but it permits a more parsimonious description in many situations (Bollerslev, 1986). The general GARCH (p,q) model is
illustration not visible in this excerpt
(6)
The second GARCH model that we will estimate is the EGARCH model which was proposed by Nelson (1991). The EGARCH model is:
illustration not visible in this excerpt
(7)
Another GARCH model that we will examine is the GJR -GARCH which was proposed by Glosten, Jaganathan and Runkle (1993).
illustration not visible in this excerpt
(8)
illustration not visible in this excerpt
The fourth GARCH model that we will present is the GARCH-in mean which was proposed by Engle, Lilien and Robins (1987). The model is
illustration not visible in this excerpt
(9)
illustration not visible in this excerpt
Finally the last model we will estimate is the component ARCH model. First of all we can write the GARCH (1,1) as
illustration not visible in this excerpt
is the unconditional variance of the series. Thus the usual GARCH has a mean reversion
tendency towards Abbildung in dieser Leseprobe nicht enthalten. A further extension is to allow this unconditional or long term volatility to vary over time. This leads to the so called component ARCH that allows mean reversion to a varying level qt instead of Abbildung in dieser Leseprobe nicht enthalten. The model is
ht — qt = α(u2t-1 − qt-1)+δ(ht-1 − qt-1)
illustration not visible in this excerpt
Section 2
In this section we present a brief statistic and graphically summary of the stocks, the general index of Athens stock market and the special index of technology-software sector. We will present the computer results, which have been obtained by Eviews software, of leptokurtosis, of the stationarity and the autocorrelation of the financial time-series. In Appendix 1 figure 1.α. we present the line graph for the stock prices of the enterprise “DIONIC” and in figure 1.b. we present the histogram for “DIONIC” in figure 1.c. we present the line graph of the stock market returns for the same enterprise and in figure 1.d. we present the histogram of the stock market returns. We see that the skewness is positive and is specifically is 0.27 and kurtosis equal with 5.13. Is already known that if there is a normal probability distribution, then skewness and kurtosis will be 0 and 3 respectively. So we conclude that there is the leptokurtosis phenomenon. From the other side, as it was expected, the stock price series is not stationary, after we applied the ADF test (Gujarati, 2002)-see Table 1.α. Appendix 1 . From the other side the stock returns are stationary as we can conclude that from the table 1.b.
As in enterprise “DIONIC” the following figures and tables in Appendix 1 present the line graphs and the histograms for the stock prices, the general index of Athens stock market and the special index of technology-software sector and the line graphs and the histograms for the returns of all above, as the tables of unit root tests.
www.marketvolume.com/glossary/l0079.asp ) as it can be shown in figures 1-8 in Appendix 1.
Also, with the matter of stationarity we conclude that all the stock series are not stationary (with not differences), but they are stationary in their first differences, while the returns of the stocks and the indices-the general index of Athens Stock Market and the special index of the sector – are stationary, as we can see that from the tables 1-8 in Appendix 1. For example Table 1.α. shows the unit root test for stock price series without first differences. Table 1.b. shows the unit root test for stock price series with first differences and Table 1.c. shows the unit root test returns of the stocks without differences and it follows with the same order.
[1] Elton E.J. Gruber, M.J. Brown, S.J. Goetzmann, (2007) Modern Portfolio Theory and Investment Analysis, 7th edition, Wiley, pp. 298-299
[2] Galagedera U.A. Don, A Review of Capital Asset Pricing Models. Monash University. Department of Econometrics and Business Statistics
Excerpt out of 91 pages — scroll top