A Semiparametric Gaussian Copula Regression Modelfor Predicting Financial Risks from Earnings Calls
Post on: 16 Март, 2015 No Comment

for Predicting Financial Risks from Earnings Calls
William Yang Wang
School of Computer Science
Carnegie Mellon University
Abstract
Earnings call summarizes the financial performance of a company, and it is an important indicator of the future financial risks of the company. We quantitatively study how earnings calls are correlated with the financial risks, with a special focus on the financial crisis of 2009. In particular, we perform a text regression task: given the transcript of an earnings call, we predict the volatility of stock prices from the week after the call is made. We propose the use of copula. a powerful statistical framework that separately models the uniform marginals and their complex multivariate stochastic dependencies, while not requiring any prior assumptions on the distributions of the covariate and the dependent variable. By performing probability integral transform. our approach moves beyond the standard count-based bag-of-words models in NLP, and improves previous work on text regression by incorporating the correlation among local features in the form of semiparametric Gaussian copula. In experiments, we show that our model significantly outperforms strong linear and non-linear discriminative baselines on three datasets under various settings.
1 Introduction
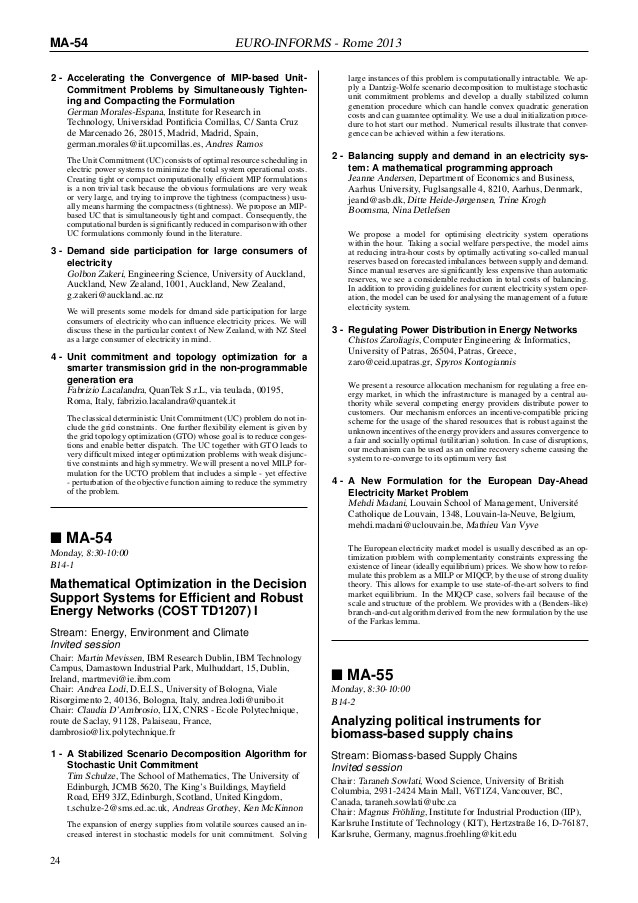
Predicting the risks of publicly listed companies is of great interests not only to the traders and analysts on the Wall Street, but also virtually anyone who has investments in the market [24 ]. Traditionally, analysts focus on quantitative modeling of historical trading data. Today, even though earnings calls transcripts are abundantly available, their distinctive communicative practices [4 ]. and correlations with the financial risks, in particular, future stock performances [35 ]. are not well studied in the past.
Earnings calls are conference calls where a listed company discusses the financial performance. Typically, a earnings call contains two parts: the senior executives first report the operational outcomes, as well as the current financial performance, and then discuss their perspectives on the future of the company. The second part of the teleconference includes a question answering session where the floor will be open to investors, analysts, and other parties for inquiries. The question we ask is that, even though each earnings call has distinct styles, as well as different speakers and mixed formats, can we use earnings calls to predict the financial risks of the company in the limited future?
Given a piece of earnings call transcript, we investigate a semiparametric approach for automatic prediction of future financial risk 1 1 In this work, the risk is defined as the measured volatility of stock prices from the week following the earnings call teleconference. See details in Section 5. To do this, we formulate the problem as a text regression task, and use a Gaussian copula with probability integral transform to model the uniform marginals and their dependencies. Copula models [37. 31 ] are often used by statisticians [16. 27. 30 ] and economists [7 ] to study the bivariate and multivariate stochastic dependency among random variables, but they are very new to the machine learning [17. 18. 44. 28 ] and related communities [12 ]. To the best of our knowledge, even though the term “copula” is named for the resemblance to grammatical copulas in linguistics, copula models have not been explored in the NLP community. To evaluate the performance of our approach, we compare with a standard squared loss linear regression baseline, as well as strong baselines such as linear and non-linear support vector machines (SVMs) that are widely used in text regression tasks. By varying different experimental settings on three datasets concerning different periods of the Great Recession from 2006-2013, we empirically show that our approach significantly outperforms the baselines by a wide margin. Our main contributions are:
We are among the first to formally study transcripts of earnings calls to predict financial risks.