Risk Analysis What Is It and When to Use Deterministic & Stochastic Risk Analyses
Post on: 22 Июнь, 2015 No Comment
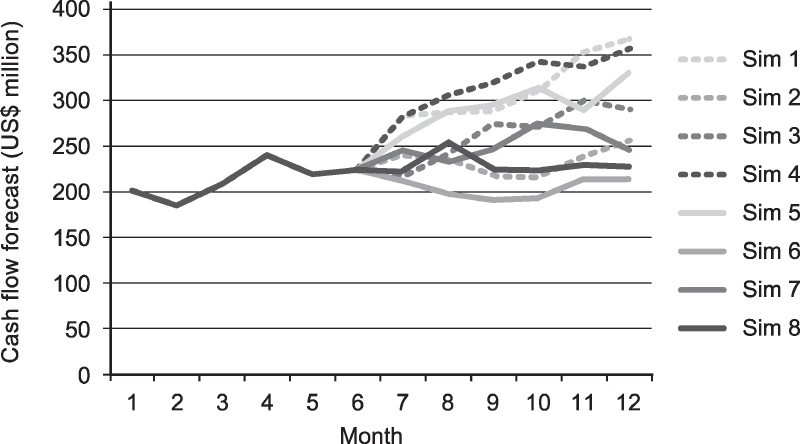
Wayne Winston
Winston discusses some of the unlimited applications of risk analysis.
Edward Biernat
Consulting with Impact, Ltd
Time. 01:03 min
The main thrust is to understand that there is variability
Risk analysis is systematic use of available information to determine how often specified events may occur and the magnitude of their consequences.
Risks are typically defined as negative events, such as losing money on a venture or a storm creating large insurance claims. However, the process of risk analysis can also uncover potential positive outcomes. By exploring the full space of possible outcomes for a given situation, a good risk analysis can both identify pitfalls and uncover new opportunities.
Risk analysis can be performed qualitatively or quantitatively. Qualitative risk analysis generally involves assessing a situation by instinct or gut feel, and is characterized by statements like, That seems too risky or Well probably get a good return on this. Quantitative risk analysis attempts to assign numeric values to risks, either by using empirical data or by quantifying qualitative assessments. We will focus on quantitative risk analysis.
Deterministic Risk Analysis Best Case, Worst Case, Most Likely
A quantitative risk analysis can be performed a couple of different ways. One way uses single-point estimates, or is deterministic in nature. Using this method, an analyst may assign values for discrete scenarios to see what the outcome might be in each. For example, in a financial model, an analyst commonly examines three different outcomes: worst case, best case, and most likely case, each defined as follows:
Worst case scenario All costs are the highest possible value, and sales revenues are the lowest of possible projections. The outcome is losing money.
Best case scenario All costs are the lowest possible value, and sales revenues are the highest of possible projections. The outcome is making a lot of money.
Most likely scenario Values are chosen in the middle for costs and revenue, and the outcome shows making a moderate amount of money.
There are several problems with this approach:
- It considers only a few discrete outcomes, ignoring hundreds or thousands of others.
- It gives equal weight to each outcome. That is, no attempt is made to assess the likelihood of each outcome.
- Interdependence between inputs, impact of different inputs relative to the outcome, and other nuances are ignored, oversimplifying the model and reducing its accuracy.
Yet despite its drawbacks and inaccuracies, many organizations operate using this type of analysis.
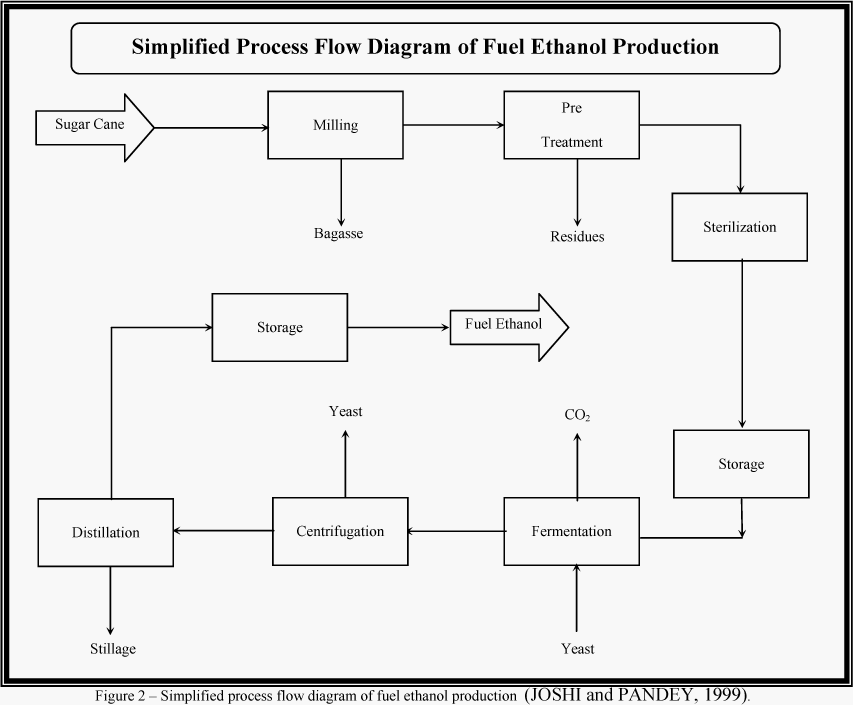
Stochastic Risk Analysis Monte Carlo Simulation
A better way to perform quantitative risk analysis is by using Monte Carlo simulation. In Monte Carlo simulation, uncertain inputs in a model are represented using ranges of possible values known as probability distributions. By using probability distributions, variables can have different probabilities of different outcomes occurring. Probability distributions are a much more realistic way of describing uncertainty in variables of a risk analysis. Common probability distributions include:
Normal Or bell curve. The user simply defines the mean or expected value and a standard deviation to describe the variation about the mean. Values in the middle near the mean are most likely to occur. It is symmetric and describes many natural phenomena such as peoples heights. Examples of variables described by normal distributions include inflation rates and energy prices.
Lognormal Values are positively skewed, not symmetric like a normal distribution. It is used to represent values that dont go below zero but have unlimited positive potential. Examples of variables described by lognormal distributions include real estate property values, stock prices, and oil reserves.
Uniform All values have an equal chance of occurring, and the user simply defines the minimum and maximum. Examples of variables that could be uniformly distributed include manufacturing costs or future sales revenues for a new product.
Triangular The user defines the minimum, most likely, and maximum values. Values around the most likely are more likely to occur. Variables that could be described by a triangular distribution include past sales history per unit of time and inventory levels.
PERT- The user defines the minimum, most likely, and maximum values, just like the triangular distribution. Values around the most likely are more likely to occur. It can generally be considered as superior to the Triangular distribution when the parameters result in a skewed distribution. An example of the use of a PERT distribution is to describe the duration of a task in a project management model.
Discrete The user defines specific values that may occur and the likelihood of each. An example might be the results of a lawsuit: 20% chance of positive verdict, 30% change of negative verdict, 40% chance of settlement, and 10% chance of mistrial.
During a Monte Carlo simulation, values are sampled at random from the input probability distributions. Each set of samples is called an iteration, and the resulting outcome from that sample is recorded. Monte Carlo simulation does this hundreds or thousands of times, and the result is a probability distribution of possible outcomes. In this way, Monte Carlo simulation provides a much more comprehensive view of what may happen. It tells you not only what could happen, but how likely it is to happen.
Monte Carlo simulation provides a number of advantages over deterministic analysis:
- Probabilistic Results. Results show not only what could happen, but how likely each outcome is.
- Graphical Results. Because of the data a Monte Carlo simulation generates, its easy to create graphs of different outcomes and their chances of occurrence. This is important for communicating findings to other stakeholders.
- Sensitivity Analysis. With just a few cases, deterministic analysis makes it difficult to see which variables impact the outcome the most. In Monte Carlo simulation, its easy to see which inputs had the biggest effect on bottom-line results.
- Scenario Analysis. In deterministic models, its very difficult to model different combinations of values for different inputs to see the effects of truly different scenarios. Using Monte Carlo simulation, analysts can see exactly which inputs had which values together when certain outcomes occurred. This is invaluable for pursuing further analysis.
- Correlation of Inputs. In Monte Carlo simulation, its possible to model interdependent relationships between input variables. Its important for accuracy to represent how, in reality, when some factors goes up, others go up or down accordingly.
Monte Carlo Simulation in Spreadsheets and Project Schedules
The most common platform for performing quantitative risk analysis is the spreadsheet model. Many people still unnecessarily use deterministic risk analysis in spreadsheet models when they could easily add Monte Carlo simulation using @RISK in Excel. @RISK adds new functions to Excel for defining probability distributions and analyzing output results. @RISK is also available for Microsoft Project, assessing risks in project schedules and budgets.
Watch how @RISK works