Contribution of CoSkewness and CoKurtosis of the Higher Moment CAPM for Finding the Technical
Post on: 4 Июнь, 2015 No Comment
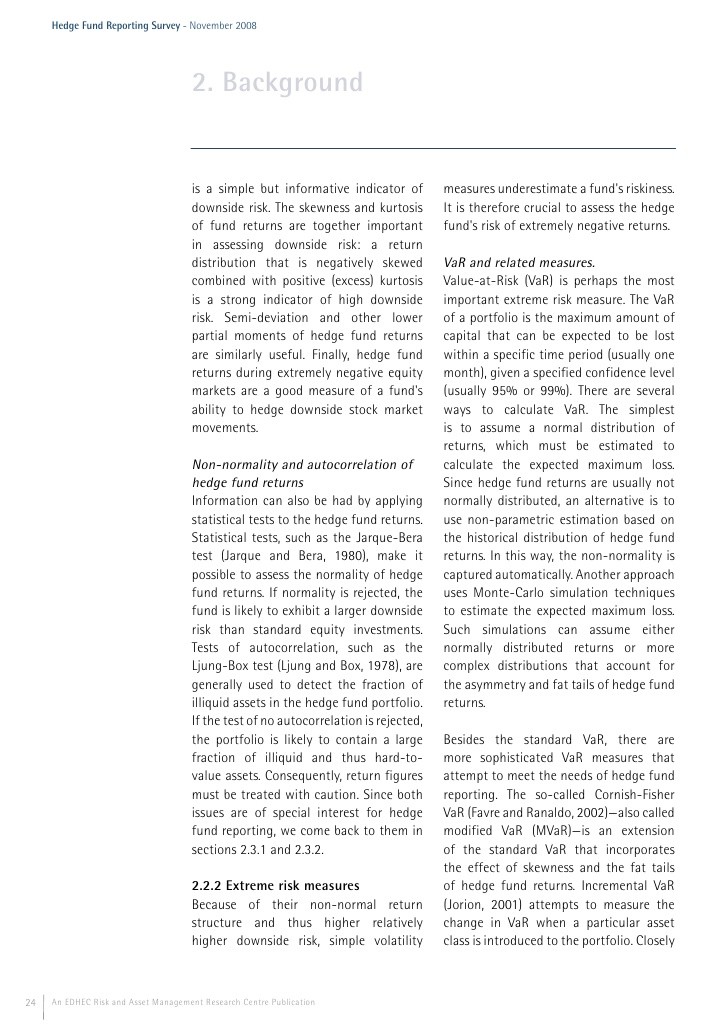
Contribution of Co-Skewness and Co-Kurtosis of the Higher Moment CAPM for Finding the Technical Efficiency
Mathematics Section, School of Distance Education, Universiti Sains Malaysia, Penang, Malaysia
Received 13 June 2013; Revised 22 November 2013; Accepted 9 December 2013; Published 16 January 2014
Academic Editor: Jean Paul Chavas
Abstract
The objective of this paper is to present the technical efficiency of individual companies and their respective groups of Bangladesh stock market (i.e. Dhaka Stock Exchange, DSE) by using two risk factors (co-skewness and co-kurtosis) as the additional input variables in the Stochastic Frontier Analysis (SFA). The co-skewness and co-kurtosis are derived from the Higher Moment Capital Asset Pricing Model (H-CAPM). To investigate the contribution of these two factors, two types of technical efficiency are derived: (1) technical efficiency with considering co-skewness and co-kurtosis (WSK) and (2) technical efficiency without considering co-skewness and co-kurtosis (WOSK). By comparing these two types of technical efficiency, it is noticed that the technical efficiency of WSK is higher than the technical efficiency of WOSK for the individual companies and their respective groups. As per available literature in the context Bangladesh stock market, no study has been conducted thus far to measure technical efficiency of companies and their respective groups by using the risk factors which are derived from the H-CAPM. In this research, the link between H-CAPM and SFA is established for measuring technical efficiency and it is believed that the findings of this study may be applied to other emerging stock markets.
1. Introduction
In the finance literature, CAPM is one of the most important developments which predicts that the expected return on an asset is linearly related to systemic risk. But, because of the large number of empirical evidence against the CAPM, the financial researchers started to search for a substitute model to describe the risk-return relationship of risky assets. This searching had led the researchers to the extension of the CAPM. The higher moment CAPM was initially proposed by Rubinstein [1 ] and sequentially developed by Kraus and Litzenberger [2 ], Fang and Lai [3 ], Hwang and Satchell [4 ], and Harvey and Siddique [5 ]. Rubinstein [1 ] noted that when the market returns are not normal (but skewed or leptokurtic), the standard CAPM is not enough to price equity returns. So, he recommended for the addition of higher moments. Kraus and Litzenberger [2 ] extended the Sharpe-Lintner CAPM model by introducing the third moment “skewness” and examined the effect of skewness in return distributions. They found that the systematic skewness (co-skewness) is capable of explaining the behavior of asset returns which was not fully explained by the traditional CAPM. Fang and Lai [3 ] showed that in the presence of skewness and kurtosis in asset return distribution, the expected excess rate of return is related not only to the systematic variance but also to the systematic skewness and systematic kurtosis in the U.S. stock market. Hwang and Satchell [4 ] investigated whether the emerging markets are better explained with the additional risk factors such as higher moments (skewness and kurtosis). They tested the higher moment CAPM by using the generalized method of moment (GMM) and found that the higher moment CAPM is better explained than the conventional mean-variance CAPM in emerging markets. Harvey and Siddique [5 ] tested the extended CAPM model which was proposed by Kraus and Litzenberger [2 ] and found that the model incorporating co-skewness is helpful in explaining some of the nonsystematic components in cross-section variation of equity returns. Brunnermeier et al. [6 ] concluded in his study that the desire for skewness can also impact the market return. Very recently, the study of Kostakis et al. [7 ] examined the preferences of the higher moments of returns’ in the asset pricing model of London Stock Exchange during the period of 1986–2008. The final results of their study fully confirmed that co-skewness and co-kurtosis premia are priced in the UK market. Young Chang et al. [8 ] investigated whether the market skewness and kurtosis risks affect the cross section of stock returns. The results of their study were contributed to the existing literature which highlights the importance of higher-moment risk in asset pricing. Carmichael and Coen [9 ] studied the effect of co-skewness on asset valuation and found the contribution of idiosyncratic co-skewness in asset pricing.
So, a number of studies have tested higher order CAPM model for developed stock markets, but there has been little work in the emerging stock markets. However, there are only a few studies in the Bangladesh context which are related to CAPM or higher moment CAPM. Alam et al. [10 ] investigated whether or not the CAPM model is working in the DSE market by using the daily data of market index and returns for the period of 1994 to 2005. They found that CAPM is not working in the market. Ali et al. [11 ] also tested the validity of the CAPM in the DSE market. They concluded that the invalidity of CAPM in the market is because of finding nonlinear relationship between risk and return and not finding beta as a complete measure of risk. Mollik and Bepari [12 ] examined the nature of instability of CAPM’s beta and found that beta instability increases with an increase in holding (sample) periods. To the best of our knowledge, no research has been done to measure the technical efficiency of companies listed in the Bangladesh stock market by using the risk factors which are derived from higher moment CAPM.
According to Berger and Humphrey [13 ], many of the researchers used either parametric approach: SFA or nonparametric approach: Data Envelopment Analysis (DEA) for investigating the technical efficiency of financial institutions, for example, banking industry [14 –18 ] and insurance industry [19. 20 ]. In this study, SFA was used instead of DEA for measuring the technical efficiency. The reason of using SFA was that it has the advantage of dealing with stochastic noise, allowing for statistical tests of hypotheses concerning production structure and degree of inefficiency. The reason of not using DEA was that DEA does not impose any assumptions about production functional form and also does not take into account random errors; hence, the efficiency estimates may be biased if the production process is largely characterized by stochastic elements [21 ].
SFA employs a composed error model in which inefficiencies are assumed to follow an asymmetric distribution, usually the half-normal or the truncated-normal, while random errors are assumed to follow a symmetric distribution, usually the standard normal [22 ]. Most past studies used the half-normal or the truncated-normal distribution as assumption about inefficiency effects model because of the ease of estimation and interpretation [23 ]. Application of different distributions, like gamma and exponential, can also be significant sometimes [24 –26 ].
2. Materials and Methods
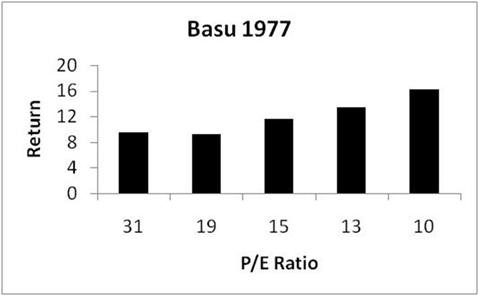
2.1. Data Sources
The paper focused on DSE market of Bangladesh, because it is not only the country’s oldest stock exchange but also one of the fast growing emerging stock markets in South Asia. In fact, when most of the world stock markets declined during the last global financial crisis in 2008, stock prices in DSE market experienced a continuous rise [27 ]. The reasons behind this were that DSE was isolated from the global financial markets and Bangladesh Bank (BB) took prompt actions to safeguard the banks and other financial institutions from the crisis. That is why DSE has significant implications for the performance of financial sector, and even the economy as a whole [28 ].
The data which was collected from DSE market belongs to 71 nonfinancial companies for the period of 2002–2011. Recently, the DSE market included 22 categories of companies, of which the following 10 categories were covered in this analysis: engineering, food and allied products, fuel and power, textiles, pharmaceuticals and chemicals, service and real estate, cement, tannery industries, ceramic industry, and miscellaneous.
2.2. Variables Construction
For this study, individual company’s return was taken as a dependent variable. Market return market capitalization, book to market ratio, and market value were taken as the independent variables. We also introduced the co-skewness and co-kurtosis terms as independent variables in the final analysis of SFA, as we know that one of the main objectives of this study was to check the contribution of co-skewness and co-kurtosis (which was derived from H-CAPM) for finding the technical efficiency of the studied companies and their respective groups in the DSE market.