Valuation Of A Path Dependent Convertible Bond Finance Essay
Post on: 23 Апрель, 2015 No Comment
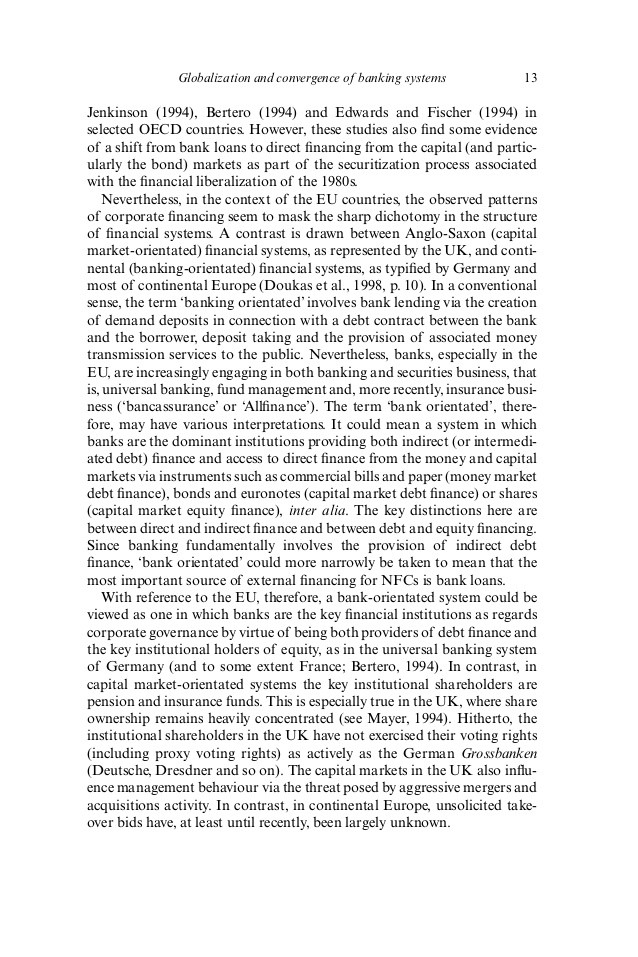
Introduction
A central problem in quantitative finance is the valuation of derivatives, including pricing and hedging. The famous theoretical analysis by Black and Scholes (1973) started the era of mathematical finance. Using this pricing theory, many derivative securities can be hedged and a market can develop quickly. A convertible bond (CB) is a corporate bond that can be converted into certain shares of the corporate stock at the bond holder’s choice. The purpose of this research is to introduce a particular path-dependent CB and present the work on the valuation of this particular CB.
High-frequency trading has developed quickly in recent years. Although it does not consider certain financial functions like financing or risk control, it is attractive because of one simple reason — it can generate profits. Limit order books were accessible only by market makers until recently. Today any participant in financial markets can place limit orders and therefore play the role of a market maker. An interesting question is how to determine the quotes bid and ask prices.
Convertible Bonds
Convertible bonds (CBs) are special types of bonds. They are attractive to both investors and issuers. Investors can benefit from an appreciation of the stock value but do not assume the risk of loss in equity value. Issuers pay a lower coupon rate than that of a straight bond. For further analysis of their advantages, see Nyborg (1996).
In addition to having the same features as straight bonds, which include issue date, maturity date, face value (FV) and coupon rate, convertible bonds also have a conversion ratio (CR), which is the pre-announced number of shares into which each convertible bond could be converted. While the conversion option is European-style, the payoff at the maturity date T is
Max = FV+CR (S(T) — )+
Therefore a convertible bond is separated into two parts, one pure debt and the other pure equity derivative (call options on stock). Consequently, the valuation is simple. Here we will consider only American-style options, which have much more complex structure.
Most CBs have a call feature as well, namely, the choice by the issuer to call a bond back at an earlier time than the maturity date, sometimes subject to certain constraints on corporate stock performance. This call feature is of two types. One is a hard-call, which provides the issuer the absolute right to recall the bond, even against the investor’s will. The other, a soft-call, adds some restrictions on the right to recall. For example, it might be required that the stock price exceeds some trigger before the call can be exercised. In this case, the issuer is still able to purchase large amounts of stock intentionally to move the stock price up, circumventing the soft restriction. An interesting variant is the 20-out-of-30-day soft-call, in which the restriction becomes that the (closing) stock price being above some trigger level for at least 20 out of the previous 30 trading days. This prevents the issuer from triggering at will. We will refer to the 20-out-of-30-day soft-call simply as soft-call.
Once the issuer exercises the call option, either simply within a hard-call window or under the circumstance that a soft-call is triggered, the holder must redeem the bond at the call price or convert the bond into stocks, of course, choosing the better value. The call option is American within the window, and the soft-call window is typically two years. The bond may also be repurchased, that is, the holder effectively has a put option to require the issuer to repurchase the bond at a pre-determined price on some specified dates.
Valuation
The valuation can be divided in two distinct frameworks — the structural approach and the reduced-form approach. The former category relates CB to the firm value and the asset volatility (Brennan and Schwartz, 1977; Brennan and Schwartz, 1980; Ingersoll, 1977). The latter category views CB as derivatives (see Goldman Sachs (1994)), therefore Monte-Carlo simulation and PDE are the two most important methods. Generally speaking, Monte-Carlo simulation can handle multi-dimensional and path-dependent problems, but it is difficult to deal with American-style options. The paper Longstaff & Schwartz (2001) describes a well known method for Monte Carlo use for American options, and the book Glasserman (2003) contains extensive discussion on this topic. It is even harder to compute the Greeks, which are crucial for hedging. See Avellaneda and Gamba (2001) for related work. For PDE methods, the major limitations are that the dimension cannot be too high (must be no more than 2 in practice), therefore many exotic derivatives like basket options are very difficult to value using PDE methods. Also, path-dependent problems need special techniques. The problem we will discuss involves heavy path dependence, in addition to dealing with American-style options.
Among PDE methods for CB valuation, a one-factor model uses the corporate stock price as the underlying variable. A two-factor model uses a stochastic volatility or a stochastic interest rate as the second underlying variable. A particular one-factor model is using local (deterministic) volatility along with local default intensity for the bonds, which can be calibrated by methods in Andersen and Buffum (2004). It has the obvious advantage that the associated PDE has only one spatial dimension.
Also, as is concluded in Grimwood and Hodges (2002), the stock price is of primary importance. Our work is based on the one-factor model. The valuation of a CB without the soft-call feature is as easy to solve numerically as an American option problem (see Wilmott et al (1995)). Many approximations to soft-callable CB can be solved with existing methods, but none of these properly consider the soft-call feature. A typical model in the CB industry presumes that the bond is callable as soon as the stock touches the trigger, known as a one-touch model. It is clearly not accurate. There are only a few publications considering this particular soft-call problem. In Navin (1999) an approximation is presented, making the bond “one-touch” callable on some days and not on others, where the “assumed” callable days are determined by the stock closing price history over the past 30 days. Another typical approximation is by the Parisian-style condition (callable if the stock closing price is above the trigger for the previous I consecutive days) for some I between 1 and 20, which is easy to solve.
Statement of Problem
For a non-path-dependent problem (like vanilla options), the value at time t is a function of the underlying stock price and can be computed from the values at time t + Δt, which is the following point on the discrete temporal grid. For a path dependent problem, the value at time t is a function of both the underlying stock price and the history path, which can be computed from the values at time t+Δt. However, in our problem, the path has too many possible values. This makes direct storage and computation infeasible. Unlike the traditional method, which stores one value for each stock price (on a discrete grid) and each history path, we store those history paths that give the same value for a fixed stock price. We develop a special storage system which is very efficient. We then follow the backward computation using the new storage method and finally compute the fair value. However, the computational complexity during the process depends on two critical quantities, which can be quite large. To handle this issue, we also develop techniques to control them. During the controlling procedures, a small amount of accuracy is sacrificed for compensation, which yields a biased-high price and a biased-low price. Finally, the implementation shows that the error is within a practically good tolerance range.
There are many interesting problems for future consideration. The most important one is to find a theoretical estimate of the error between the biased-high price and the biased-low price, so as to guarantee the accuracy of our method. Since we are using finite difference PDE, interesting future work also includes the convergence property when the time step and space step tend to zero. To change from the current explicit scheme to an implicit scheme would also be an important development. In addition to this particular valuation problem, we are also interested in extending the path set method to other path-dependent dynamic programming problems.
Purpose of the study
Economics tells us that the price will increase if demand exceeds supply and the price will decrease if supply exceeds demand. This simple fact is reflected by the mechanics of these two types of orders. When a large market buy order arrives, not only the limit sells order with the lowest price but also a few others are executed. Consequently, the actual average trading price is higher than the existing best ask price, with a temporary increasing price impact. Conversely, a large market sell order can generate a temporary decreasing price impact. The temporary price impact incurs a cost, which is assumed by the market order trader. The profits diminish but do not disappear for the limit order traders.
We are interested in answering the question of how to make the bid and ask so as to maximize profits, by formulation it as an optimal control problem. The pioneer work Avellaneda and Stoikov (2008) combines the utility framework in Ho and Stoll (1981) and other literature regarding the microstructure of limit order books. The underlying diffusion process is exactly one stock true value and the decision contains a bid price and an ask price. Decisions are made at every moment within a finite time horizon to maximize the expectation of a utility function at the terminal time. The utility function is an exponential function of wealth, which consists of the cash part and the equity part. Different decisions give different limit order execution intensities and therefore influence profits. In Avellaneda and Stoikov (2008) an approximate solution to the associated Hamilton-Jacobi-Bellman (HJB) equation is given, and it is concluded that the optimal quotes bracket the true value with a spread determined by the inventory position.
Our work is mainly an extension of Avellaneda and Stoikov (2008), which we call the full observation model. We modify the basic model to make the stock true value observable only at discrete trading times, and study the optimal submission strategies with such restricted information. We call it the partial observation model. The purpose is to have a more realistic model. It involves more state variables but the optimal decision can only depend on observable state variables. Therefore it is more complicated. We find two intrinsic invariance properties of the value function, and reduce the HJB equation by two dimensions to make a numerical solution possible.
The numerical solutions and simulations indicate the similarities and differences with the full observation model. We also propose several interesting future problems in this area, including alternative modeling of the stock true price process and allowing many limit orders with distinct prices.
Literature Review
Limit Order and Market Order
Limit orders and market orders are the two most common trading methods today. Empirical evidence is provided in Hollifield et al (2006) showing that the limit orders market is a good trading mechanism. The following definitions and analyses are obtained from SEC limit order (2001) and SEC market order (2001).
“A limit order is an order to buy or sell a security at a specific price. A buy limit order can only be executed at the limit price or lower, and a sell limit order can only be executed at the limit price or higher. A market order is an order to buy or sell a stock at the (best) current market price. When you place a market order, you can’t control the price at which your order will be filled.”
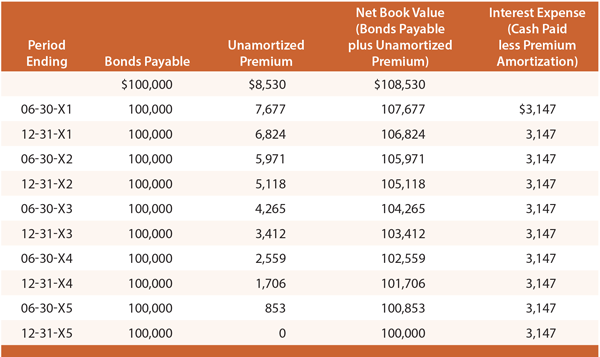
“For example, if you want to buy the stock of a ‘hot’ IPO (Initial Public Offering) that was initially offered at $9, but don’t want to end up paying more than $20 for the stock, you can place a limit order to buy the stock at any price up to $20. By entering a limit order rather than a market order, you will not be caught buying the stock at $90 and then suffering immediate losses if the stock drops later in the day or the weeks ahead.”
The advantage of a market order is you are almost always guaranteed your order will be executed (as long as there are willing buyers and sellers). The disadvantageis the price you pay when your order is executed may not always be the price you obtained from a real-time quote service or were quoted by your broker. This may be especially true in fast-moving markets where stock prices are more volatile. When you place an order ‘at the market’, particularly for a large number of shares, there is a greater chance you will receive different prices for parts of the order.
“Remember that your limit order may never be executed because the market price may quickly surpass your limit before your order can be filled. But by using a limit order you also protect yourself from buying the stock at too high a price.”
For detailed analysis on their advantages and disadvantages, see Lo and Sapp (2005) and Harris and Hasbrouck (1996). A comprehensive analysis of the rationale for limit order trading is given in Handa and Schwartz (1996), and one of its conclusions is that placing a combination of buy and sell limit orders as a pure strategy can be profitable. Much research has been done on optimal submission strategies. Equilibrium dynamics is used in Parlour (1998) to establish an optimal submission. In Hollifield et al (2004) the link between economic trade-offs and the order submissions is analyzed. In Wald and Horrigan (2005) the case of a possibly risk-averse investor placing a limit order is considered. In Lillo (2007) a utility maximization approach is used to determine the optimal submission. Strategies in these papers do not consider a high-frequency setting, that is, canceling the previous limit order and resubmitting a new one very frequently.
Although there is still debate on the usefulness of high-frequency trading (Polyn (2002)), it is being used in the current financial markets. High-frequency trading is essentially a method for portfolio management and does not really have any financial function, such as hedging. It has grown very fast in recent years, but its future is unclear. It may in fact be forbidden or restricted in some sense by regulators because of possible damage to the financial markets. However, it may actually develop further to be the prevailing trading mode and replace the current one, as traditional trading was replaced by modern online trading showing the need to this topic to be studied.
Profits can be generated via high-frequency trading in a limit order book. The full observation model has given the optimal bid and ask strategies based on the stock true value and the current stock inventory position. We built the partial observation model, which introduces a more realistic scenario. This model has many similar characteristics as the previous one, and it also creates more interesting trading behavior. Our numerical result shows that the partial observation trading is slightly less profitable than the full observation trading, which is consistent with the theoretical result. The strategy is still very profitable despite the risk from the unobserved information.
Research method
In this paper we will employ the model to examine the question of whether initial offerings of convertible bonds are underpriced. For primitive (non-derivative) securities, under pricing is defined as the excess return obtained from buying the security at its issue price, then immediately selling it at the first market determined price.
Under pricing has been well documented across a wide range of primitive securities. For initial public offerings of common stock, studies have found wildly varying degrees of under pricing for different data sets, ranging from under pricing in the range of 10-15% of the issue price (see Ibbotson, Sindelar and Ritter (1994) for a review of the topic. For seasoned offerings of common stock, studies have found much smaller under pricing, averaging between 27 and 82 basis points. For straight corporate debt securities, studies have found a small degree of under pricing of between 15 and 50 basis points.
Authors have proposed a variety of explanations for the existence of under pricing. The hypotheses can be loosely divided into asymmetric information based theories and market structure explanation. The information based theories typically posit that various players (firm managers, investment bankers, and potential investors) have different information pertaining to the value of the securities. Various configurations of asymmetric information can then lead to under pricing: (i) as an efficient mechanisms to induce investors to reveal their information prior to final pricing, or (ii) to prevent a “winner’s curse” type market failure or (iii) at attract the first few orders, in an attempt to create an “information cascade” where previously undecided investors take these early orders as a positive signal, inducing further orders and ultimately resulting in a fully subscribed offering, or finally (iv) as a method for the underwriters to exploit superior market knowledge to appropriate part to the value of the offering.
Among the more commonly cited market structure explanations resulting in under pricing are: (i) imperfect price competition among underwriters, allowing them to impose below market prices on issues; (ii) high costs to the underwriter of a failed offering, where under pricing results from underwriter risk-aversion; and (iii) price pressure where investors require a discount to fair value to compensate them for the transaction costs incurred as a result of the necessary portfolio rebalancing after absorbing a large new issue. The asymmetric information theories are more typically applied to under pricing of IPOs of common stock, whereas the market structure arguments tend to be applied more frequently to explain under pricing of corporate bonds. However, nothing in the logic of these arguments precludes them from being applied to other asset classes, and all of them are potential explanations for under pricing of convertible bonds new issues.
The data sample
Our data sample will consist of 50 issuances of convertible bonds issued by 50 different firms between 2000 to 2010. The data will include all relevant contractual specifications for the bonds (e.g. call schedules, put schedules, conversion provisions, etc.). The issue data will include issue size, issue price and the stock price against which the issuance was priced for each of issuances the data will include a traded bond price within 10 trading days of the issuance. The researcher will eliminate from the sample any issuance for which the first available trade is more than 10 trading days after issuance date. The data will be obtained from the Roberstson Stephens (RSCo) convertible security database, which contain complete issuance data on all publicly announced convertible issuances, as well as traded prices for all convertible securities for which RSCo made a market.