The use of decision tree analysis
Post on: 16 Март, 2015 No Comment
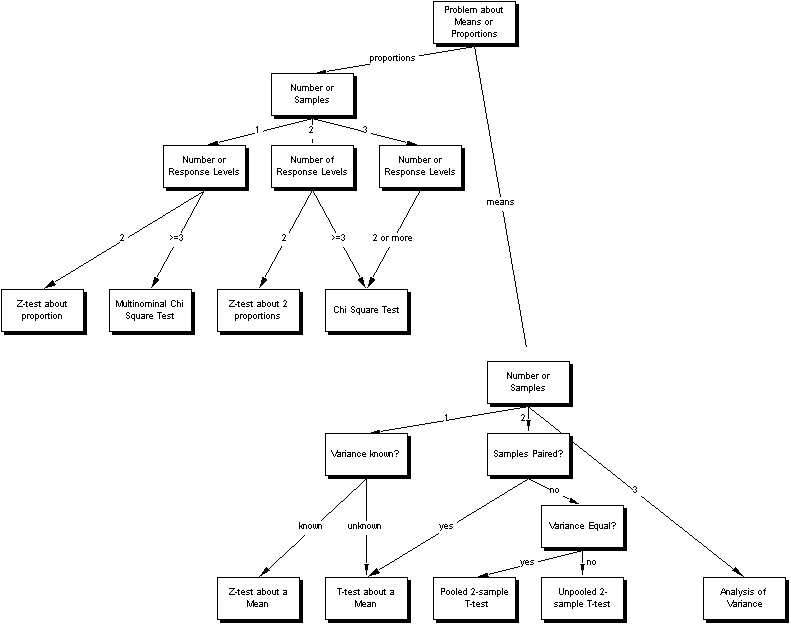
The main focus of this seminar paper is on decision tree analysis and its applications in various industries. It also discusses about issues of decision making under uncertainty and risks involved in some of the projects.
Statement of the problem
objectives of the study
Significance of the study
about decision tree analysis
Scope and Limitations of the study
Scope
The scope of the study is learning about decision tree analysis, its role in decision making, its applications in various industries and the issues of decision making under uncertainty and risks involved in some of the projects.
limitations
This study does not include techniques of decision making under certainty. It does not provide in-depth knowledge of all techniques of decision making under uncertainty and risk.
Review of literature
Basics of decision tree analysis
Decision tree is a tree-like graph or model of decisions and their possible consequences, including chance event outcomes, resource costs, and utility. Its common application is in operations research, especially in decision analysis, for identifying a strategy to attain an objective. Other applications include its use as a descriptive means for calculating conditional probabilities. It acts as visual tool where the expected values (or expected utility) of competing alternatives are calculated and guides in choosing the best alternative.
Decision trees depict the sequence of interrelated decisions and the expected results of making choice among alternatives. When a decision is to be made, there are generally more than one choices or options. The available choices are depicted in tree form starting at the left with the risk decision branching out to the right with possible outcomes. Decision trees are usually used for risk events associated with time or cost.
A decision tree consists of 3 types of nodes:-
1. Decision nodes which are commonly represented by squares
2. Chance nodes which are represented by circles
3. End nodes which are represented by triangles
Decision tree is drawn from left to right and has splitting paths but no converging paths. Therefore, in cases of elaborate future events, it is often difficult to draw manually. Nowadays various solutions and softwares are provided by many companies for drawing decision trees, analyzing the results and defining the best alternative amongst the available choices.
Steps in decision tree analysis
Main steps in decision tree analysis are as follows:
1. Identifying the problem and alternatives
To understand the problem and develop alternatives, it is necessary to acquire information from different sources like marketing research, economic forecasting, financial analysis, etc. As the decision situation unfolds, various alternatives may arise which are to be identified. There would also be kinds of uncertainties in terms of market size, market share, prices, cost structure, availability of raw material and power, governmental regulation. Technological change, competition, etc. Recognising that risk and uncertainty are inherent characteristics of investment projects, persons involved in analyzing the situation must be encouraged to express freely their doubts, uncertainties, and reservation and motivated to suggest contingency plans and identify promising opportunities in the emerging environment.
2. Delineating the decision tree
The decision tree represents the anatomy of decision situation. It illustrates
decision points along with the alternative options available for experimentation and action at these decision points
chance points where outcomes are dependent on a chance process and the likely outcomes at these points
This decision tree diagrammatically reflects the nature of decision situation in terms of alternative courses of action and chance outcomes which have been identified in the first step of the analysis.
If myriad possible future events and decisions are considered, it can become very complex and cumbersome. As a result, it would not be a useful tool of analysis. If many elaborate events are taken into account then it may obscure the critical issues. Hence it is necessary to simplify the decision tree so that focus can be given on major future alternatives.
3. Specifying probabilities and monetary outcomes
After delineating the decision tree, probabilities corresponding with each of the possible outcomes at various chance points and monetary value of each combination of decision alternative and chance outcome have to be gathered.
The probabilities of various outcomes can be defined objectively. For instance, based on objective historical data the probability of good monsoon can be defined. On the other hand, probabilities for real life outcomes are somewhat difficult and cannot be obtained. For example, one cannot determine the probabilities for success of a new automobile launch. These have to be defined subjectively and based on experience, judgment, understanding of informed executives and their intuition. Also, it is difficult to assess cash flows corresponding to these outcomes. So again judgment of experts helps in defining these cash flows.
4. Evaluating various decision alternatives
The final step in decision tree analysis includes evaluation of various alternatives. This can be done as follows:
Starting with the right- hand end of the tree and then we calculate the expected monetary value at various chance points that come first as we proceed leftward.
Given the expected monetary values of chance points in step 1, evaluate the alternatives at the final stage decision points in terms of their expected monetary values.
At each of the final stage decision points, select the alternative which has the highest expected monetary value and truncate the other alternatives. Each decision point is assigned a value equal to the expected monetary value of the alternative selected at that decision point.
Proceed backward (leftward) in the same manner, calculating the expected monetary value at chance points, selecting the decision alternative which has the highest expected monetary value at various decision points, truncating inferior decision alternatives, and assigning values to decision points, till the first decision point is reached.
Advantages
Amongst decision support tools, decision trees have several advantages:
Easy to interpret and understand
With availability of little hard data this method helps in generating important insights
Result provided by a model/ software can be easily explained
Can be used in combination with other decision techniques
Example
Decision trees can be used to optimize an investment portfolio. The following example shows a portfolio of 7 investment options (projects). The organization has $10,000,000 available for the total investment. Bold lines mark the best selection 1, 3, 5, 6, and 7, which will cost $9,750,000 and create a payoff of 16,175,000. All other combinations would either exceed the budget or yield a lower payoff.
Decision Making Tools: Decision Tree Analysis and EMV
Decision Tree Analysis
In decision tree analysis, a problem is depicted as a diagram which displays all possible acts, events, and payoffs (outcomes) needed to make choices at different points over a period of time.
Example of Decision Tree Analysis: A Manufacturing Proposal
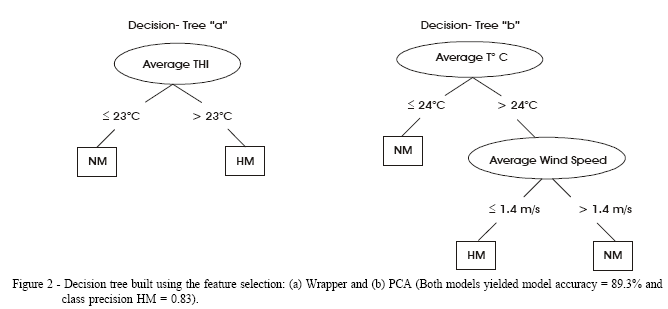
The company is assessing a new product development proposal. The cost of the development project is $500,000. The probability of successful development is projected to be 70%. If the development is unsuccessful, the project will be terminated. If it is successful, the manufacturer must then decide whether to begin manufacturing the product on a new production line or a modified production line. If the demand for the new product is high, the incremental revenue for a new production line is $1,200,000, and the incremental revenue for the modified production line is $850,000. If the demand is low, the incremental revenue for the new production line is $700,000, and the incremental revenue for the modified production line is $150,000. All of these incremental revenue values are gross figures, i.e. before subtracting the $500,000 development cost, $300,000 for the new production line and $100,000 for the modified production line. The probability of high demand is estimated as 40%, and of low demand as 60%.
The development of a decision tree is a multi step process. The first step is to structure the problem using a method called decomposition, similar to the method used in the development of a work breakdown structure. This step enables the decision-maker to break a complex problem down into a series of simpler, more individually manageable problems, graphically displayed in a type of flow diagram called a decision tree. These are the symbols commonly used:
The second step requires the payoff values to be developed for each end-position on the decision tree. These values will be in terms of the net gain or loss for each unique branch of the diagram. The net gain/loss will be revenue less expenditure. If the decision to not develop is made, the payoff is $0. If the product development is unsuccessful, the payoff is — $500,000. If the development is successful, the decision is to build a new production line (NPL) or modify an existing production line (MPL). The payoff for the NPL high demand is ($ 1,200,000 — $500,000 development cost -$300,000 build cost) or $400,000. For a low demand, the payoff is ($700,000 — $500,000 development cost -$300,000 build cost) or -$100,000. The payoff for the MPL high demand is ($850,000 -$500,000 development cost — $100,000 build cost) or $250,000. For a low demand, the payoff is ($720,000- $500,000 development cost — $100,000 build cost) or $120,000.
The third step is to assess the probability of occurrence for each outcome:
Development Successful = 70% NPL High Demand = 40% MPL High Demand = 40%
Development Unsuccessful = 30% NPL Low Demand = 60% MPL Low Demand = 60%
Probability Totals* 100% 100% 100%
*Probabilities must always equal 100%, of course.
The fourth step is referred to as the roll-back and it involves calculating expected monetary values (EMV) for each alternative course of action payoff. The calculation is (probability X payoff) = EMV This is accomplished by working from the end points (right hand side) of the decision tree and folding it back towards the start (left hand side) choosing at each decision point the course of action with the highest expected monetary value (EMV).
Decision D2:
New Production Line vs. Modified Production Line
high demand + low demand = EMV high demand + low demand = EMV
(4 0% X $400,000) + (60%X -$100,000) (40% X $250,000)+(60% X $120,000)
$100,000 $172,000
Decision Point 2 Decision: Modified Production Line with an EMV of $172,000
Decision 1: Develop or Do Not Develop
Development Successful + Development Unsuccessful
(70% X $172,000) (30% x (- $500,000))
$120,400 + (-$150,000)
Decision Point 1 EMV=(-$29,600)
Decision: DO NOT DEVELOP the product because the expected value is a negative number.
When doing a decision tree analysis, any amount greater than zero signifies a positive decision. This tool is also very useful when there are multiple cases that need to be compared. The one with the highest payoff should be picked.
Example
The project manager can use ‘decision tree analysis’ when a decision involves a series of several interrelated decisions. The project manager computes the ‘Expected Monetary value’ (EMV) of all strategies and chooses the strategy with the highest EMV.
Assume that the project manager has four alternative strategies, S1, S2, S3, and S4. The resultant values for each strategy at different probability levels are R1, R2, and R3. Assume that the probability of occurrence of these results is 0.5, 0.2 and 0.3. The payoff matrix for this problem is given in table 1.