Financial Mathematics Course Descriptions Stanford University
Post on: 11 Апрель, 2015 No Comment

These are the course descriptions for the required courses. The course descriptions for the prerequisite and elective courses can be found in the Stanford Bulletin.
MATH 227 Partial Differential Equations and Diffusion Processes
Parabolic and elliptic partial differential equations and their relation to diffusion processes. First order equations and optimal control. Emphasis is on applications to mathematical finance. Prerequisites: Math 131 and Math 136/Stats 219, or equivalents.
MATH 236 Introduction to Stochastic Differential Equations
Brownian motion, stochastic integrals, and diffusions as solutions of stochastic differential equations. Functionals of diffusions and their connection with partial differential equations. Random walk approximation of diffusions. Prerequisite: 136 or equivalent and differential equations.
MATH 238/STATS 250 Mathematical Finance
Stochastic models of financial markets. Forward and futures contracts. European options and equivalent martingale measures. Hedging strategies and management of risk. Term structure models and interest rate derivatives. Optimal stopping and American options. Corequisites: MATH 236 and 227 or equivalent.
MATH 239 Computation and Simulation in Finance
Monte Carlo, finite difference, tree, and transform methods for the numerical solution of partial differential equations in finance. Emphasis is on derivative security pricing. Prerequisite: 238 or equivalent.
MATH 240 Topics in Financial Mathematics: Fixed Income Models
Continuous time models for arbitrage-free pricing of interest rates derivatives. Bonds, yields and the construction of yield curves. Short rates models. Yield curve models. Forward measures. Caps, floors, swaps, swaptions and bond options. LIBOR market model. Prerequisite: MATH 238
STATS 237 Theory of Investment Portfolios and Derivative Securities
Asset returns and their volatilities. Markowitzs portfolio theory, capital asset pricing model, multifactor pricing models. Measures of market risk. Financial derivatives and hedging. Black-Scholes pricing of European options. Valuation of American options. Implied volatility and the Greeks. Prerequisite: STATS 116 or equivalent
STATS 238 Policy & Strategy Issues in Financial Engineering
(Same as LAW 564). This is a non-technical course that will focus on a series of case studies each designed to illuminate a serious public policy issue raised by the evolution of modern financial engineering. These will include discussions of Freddie Mac, Fannie Mae, sub-prime and Alt-A mortgages and the flaws of AAA CDOs; the spectacular losses by Orange County and the Florida Local Government Investment Pool and the challenges posed by unregulated investment pools; how credit default swaps are likely to change with central clearing using the PIIGS (Portugal/ Ireland/ Iceland/ Greece/ Spain), the monolines, AIG, Lehman and MF Global as examples; views of rogue trading using the similarities and disparities of Askin, Madoff, Barings, Soc Gen and UBS for discussion; and Risk Management 101. the why/ how/ where/ when firms went wrong plus what to keep and what to throw out in the next phase of risk programs among other case studies. The subject matter, by necessity, is multi-disciplinary and so the course is particularly suited to those students having an interest in public policy and the evolution of modern financial markets. This includes students from the law or business schools, or the public policy, economics, EES, political science, or financial math and engineering programs among others. Several themes will tie the case studies, reading and discussions together:-Is this an example of an innovation that got too far ahead of existing operations, risk management, legal, accounting, regulatory or supervisory oversight?-How might temporary infrastructure be implemented without stifling innovation or growth?-How might losses be avoided by requiring permanent infrastructure sooner? Will Dodd-Frank, Basel III, etc. help to prevent such problems? What are the potential unintended consequences?-Is this an example of improperly viewing exposures that are subject to uncertainty or incorrectly modeling risk or both? Guest speakers will be invited to share their experiences. This course will aim to provide a practitioner(s) view of financial engineering over the past 3 decades as well as a broad understanding of what went right and what went wrong plus cutting edge views of the future of financial engineering. Prerequisite: STATS 237 or equivalent and consent of instructor.
STATS 240 Statistical Methods in Finance
Regression analysis and applications to investment models. Principal components and multivariate analysis. Likelihood inference and Bayesian methods. Financial time series. Estimation and modeling of volatilities. Statistical methods for portfolio management. Prerequisite: STATS 200 or equivalent.
STATS 241 Econometric Modeling Methodology and Applications to Financial Markets
Substantive and empirical modeling approaches. Statistical trading strategies and their evaluation. Nonparametric regression. Advanced time series modeling and forecasting. Options and interest rate markets. Credit markets and default risk modeling. Prerequisite: 240 or equivalent. (2014-15)
STATS 242 Algorithmic Trading and Quantitative Strategies
An introduction to financial trading strategies based on methods of statistical arbitrage that can be automated. Methodologies related to high frequency data and stylized facts on asset returns; models of order book dynamics and order placement, dynamic trade planning with feedback; momentum strategies, pairs trading. Emphasis on developing and implementing models that reflect the market and behavioral patterns. Prerequisite: STATS 240 or equivalent.
STATS 243 Financial Models and Statistical Methods in Risk Management
Banking and bank regulation. Market risk and credit risk, asset and liability management. Logistic regression, generalized linear models and generalized mixed models. Censored data and survival analysis, loan prepayment and default as competing risks. Back testing, stress testing and Monte Carlo methods. Risk surveillance, early warning and adaptive risk control methodologies. Prerequisite: STATS 240 or equivalent.
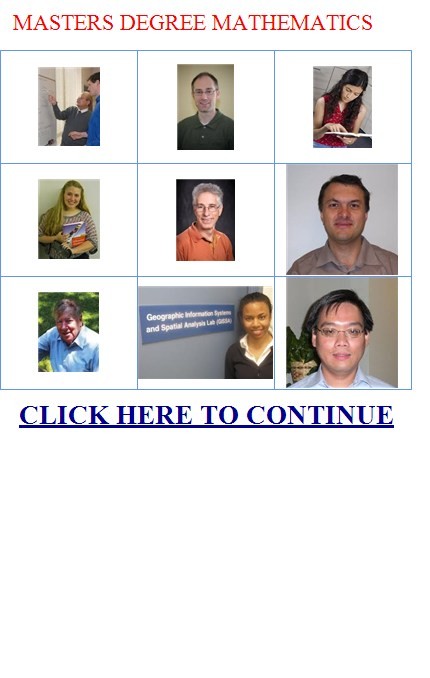
STATS 315A Modern Applied Statistics: Learning
Over view of supervised learning. Linear and related methods. Model selection, least angle regression and the lasso, stepwise methods. Classification. Linear descriminat analysis, logistic regression, and support vector machines (SVMs). Basis expansions, splines and regularization. Kernel methods. Generalized additive models. Kernel smoothing. Gaussian mixtures and the EM algorithm. Model assessment and selection: crossvalidation and the bootstrap. Pathwise coordinate descent. Sparse graphical models. Prerequisites: STATS 305, 306A, B or consent of instructor.
STATS 315B Modern Applied Statistics: Data Mining
Two-part sequence. New techniques for predictive and descriptive learning using ideas that bridge gaps among statistics, computer science, and artificial intelligence. Emphasis is on statistical aspects of their application and integration with more standard statistical methodology. Predictive learning refers to estimating models from data with the goal of predicting future outcomes, in particular, regression and classification models. Descriptive learning is used to discover general patterns and relationships in data without a predictive goal, viewed from a statistical perspective as computer automated exploratory analysis of large complex data sets.
STATS 362 Monte Carlo
Random numbers and vectors: inversion, acceptance-rejection, copulas. Variance reduction: antithetics, stratification, control variates, importance sampling. MCMC: Markov chains, detailed balance, Metropolis-Hastings, random walk Metropolis,
independence sampler, Gibbs sampling, slice sampler, hybrids of Gibbs and Metropolis, tempering. Sequential Monte Carlo. Quasi-Monte Carlo. Randomized quasi-Monte Carlo. Examples, problems and motivation from Bayesian statistics,
machine learning, computational finance and graphics.
MS&E 347 Credit Risk: Modeling and Management
Credit risk modeling, valuation, and hedging emphasizing underlying economic, probabilistic, and statistical concepts. Point processes and their compensators. Structural, incomplete information and reduced form approaches. Single name products: corporate bonds, equity, equity options, credit and equity default swaps, forwards and swaptions. Multiname modeling: index and tranche swaps and options, collateralized debt obligations. Implementation, calibration and testing of models. Industry and market practice. Data and implementation driven group projects that focus on problems in the financial industry. Prerequisites: stochastic processes at the level of MSE 321, 322 or equivalent, and financial engineering at the level of MSE 342, MATH 180, MATH 240, FINANCE 622 or equivalent.
FINANCE 622 Dynamic Asset Pricing Theory
This course is an introduction to multiperiod models in finance, mainly pertaining to optimal portfolio choice and asset pricing. The course begins with discrete-time models for portfolio choice and security prices, and then moves to a continuous-time setting. The topics then covered include the Black-Scholes model of asset pricing and some of its extensions, models of the term structure of interest rates, valuation of corporate securities, portfolio choice in continuous-time settings, and finally, general-equilibrium asset pricing models. Students should have had some previous exposure to general equilibrium theory and some basic courses in investments. Strong backgrounds in calculus, linear algebra, and probability theory are recommended. Problem assignments are frequent and, for most students, demanding. Prerequisite: FINANCE 620 or permission of instructor.