DSstar DATA MINING AND RETURN ON INVESTMENT (PART II)
Post on: 25 Апрель, 2015 No Comment
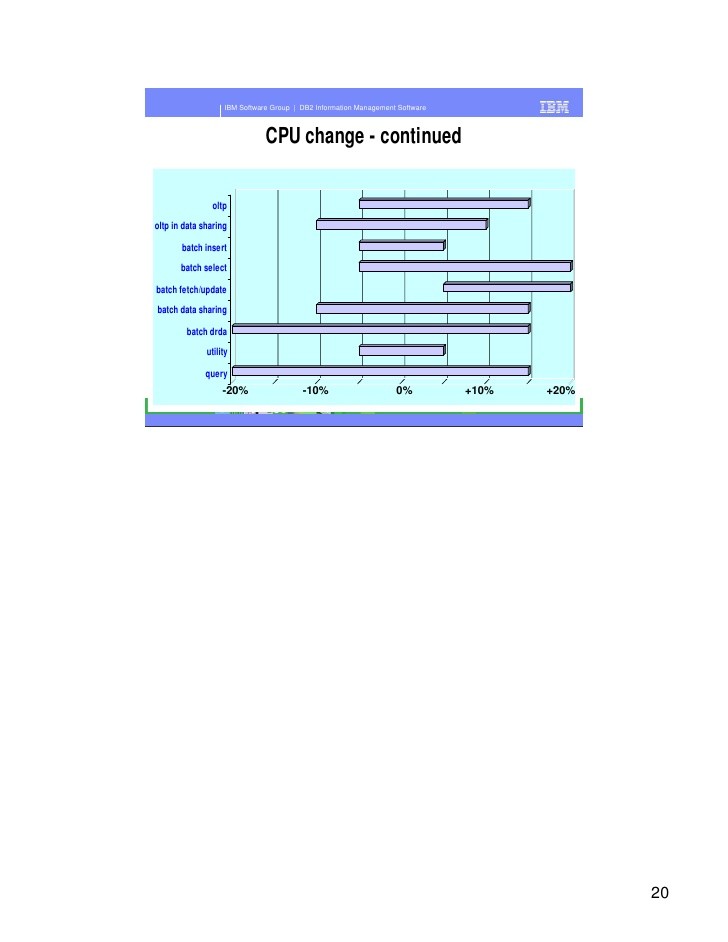
DATA MINING AND RETURN ON INVESTMENT (PART II)
By Ed Colet
Data mining tools will discover interesting patterns buried within the data and develop predictive models. These models will have various measures for indicating how well they fit the data. But quite often, it’s not clear how to make a decision on the basis of some of the measures reported as part of data mining analyses. How does one interpret things such as support, confidence, lift, interestingness and other things in a practical way? As a result, there is often a disjoint between the output of an analysis and the decision-making considerations that follow. One way to narrow this gap is to cast data mining results into terms that the decision-maker (who is often not the analyst) can understand. This is usually by framing information in terms of financials and return on investment issues. There are two general options to do this, both of which can be greatly improved through the use of data mining: (1) include financial data to be mined directly, (2) convert metrics into financial terms.
Access Financial Information During Data Mining
The most straightforward and simplest way to frame decisions in financial terms is to augment the raw data that’s typically mined to also include financial data. Many organizations are investing and building data warehouses, and data marts. The design of a warehouse or mart includes considerations about the types of analyses and information required for expected queries. Designing warehouses in a way that enables access to financial data along with access to more typical data on product attributes, customer profiles, etc can be helpful. Addressing this issue during the design of the warehouse is much easier than trying to re-engineer the warehouse after it’s implemented to include financial information.
Sound data warehouse / datamart design can save time in making analysis more powerful and more comprehensible. This is true for both descriptive as well as quantitative results. For example, a data mining tool can discover patterns such as: females between ages 35-44, in NY, that did not attend graduate school and have less than 2 children, are more likely to purchase items from the Spring catalog. But if financial information is included as part of the raw data that is mined, then the reporting of the same pattern can include information that this subgroup generates $50,000 per month, and 68% of the total revenue. Formal quantitative models also become more. For example, a simple regression equation may now have a financial attribute such as revenue as the dependent variable (Y). Other variables, such as the number of pages of advertising purchased as independent variable (X) need not change. Then, in the model, Y=aX+c, ‘a’ is readily interpreted as the additional revenue generated for each additional page of advertising that’s purchased, over an initial constant rate, ‘c’.
Converting Data Mining Metrics Into Financial Terms
But it is not always the case that financial data is readily available to be mined. In this case, the next option is to base decision-making on the metrics that is reported by data mining models. But as the following example illustrates, good predictive models are not necessarily good business models.
A common data mining metric is the measure of Lift. Lift is a measure of what is gained by using the particular model or pattern relative to a base rate in which the model is not used. High values mean much is gained. It would seem then that one could simply make a decision based on Lift. But one must be cautious because lift measures are sensitive to the base rates and sample size. For example, a pattern may find an association and rule for responding to promotional mailings: If GroupA, then Response. Lift is calculated as Probability of a Response given GroupA divided by the base probability of Response. But if the base response rate is low, and the number of people classified as GroupA also low, then it’s possible to have a high lift measure. What this means is that mailing to this small subgroup will result in a high response rate, but quite possibly a lower total number of responses — and therefore fewer sales and less revenue.
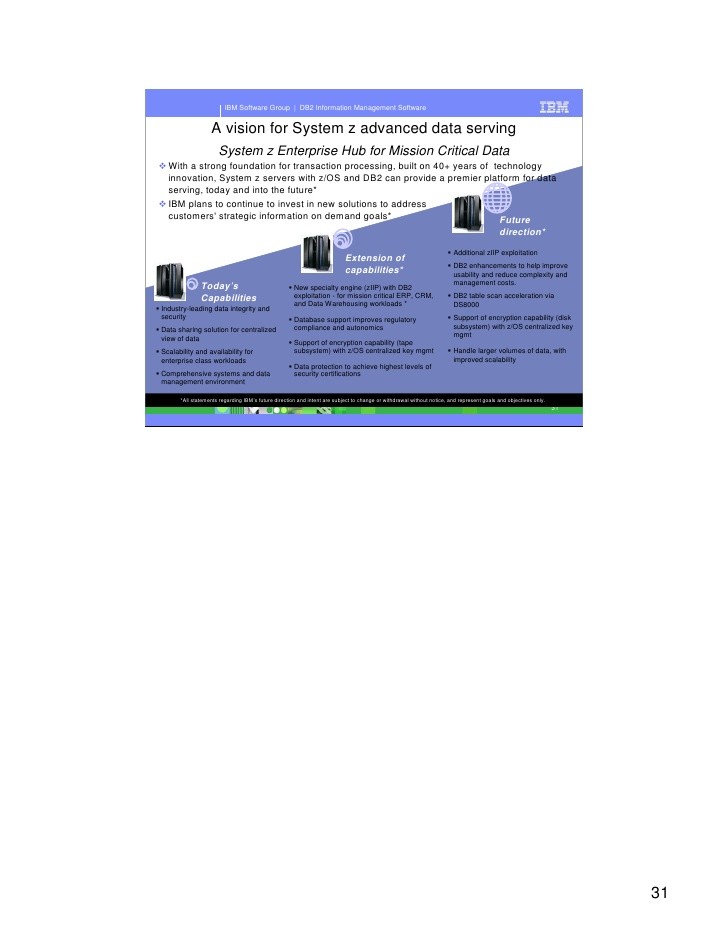
The way to link predictive models with business models is by incorporating financial information. Strong business models will typically consider costs of analytical research that supports the building of predictive models, as well as other factors. (See last week’s column, Formal computations and considerations in calculating a return on investment (ROI)www.tgc.com/dsstar/99/0817/100942.html. DSstar, Aug 17, 1999: Vol. 3, No. 33).
Data mining software can also refine an understanding of business costs. For example, churn (customer turnover) is a problem in various industries (telecommunications, insurance, etc). A Company can know it’s general churn rate, and typically wants to decrease it. They may generally know the costs to attract a customer, the cost to retain the customer, and the lost opportunity cost if the customer leaves. But data mining can discover markedly different churn rates for sub groups within the data. It may then be better to encourage customers with certain characteristics to leave while spending more on retaining more profitable customers. Many organizations, with the help of data mining models tied to financial information, are discovering and acting on such patterns.
Discovering more refined patterns that are tied to the financial costs and business models can all translate to positive benefits for organizations that use data mining software effectively.
Ed Colet is the Acting Director of Research at Virtual Gold Inc. responsible for developing analytical methods for data mining and for investigating human factors and usability issues of business intelligence systems. At present, he is in the final stage of completing a doctoral dissertation in the Cognition and Perception program at New York University’s Department of Psychology. Ed has also worked for IBM Research at the T.J. Watson Research Center. At IBM, Ed was a member of the group that developed Advanced Scout, the data mining application for NBA teams. His research interests focus on statistical methods and human factors.