Bayesian Financial Methods
Post on: 16 Март, 2015 No Comment
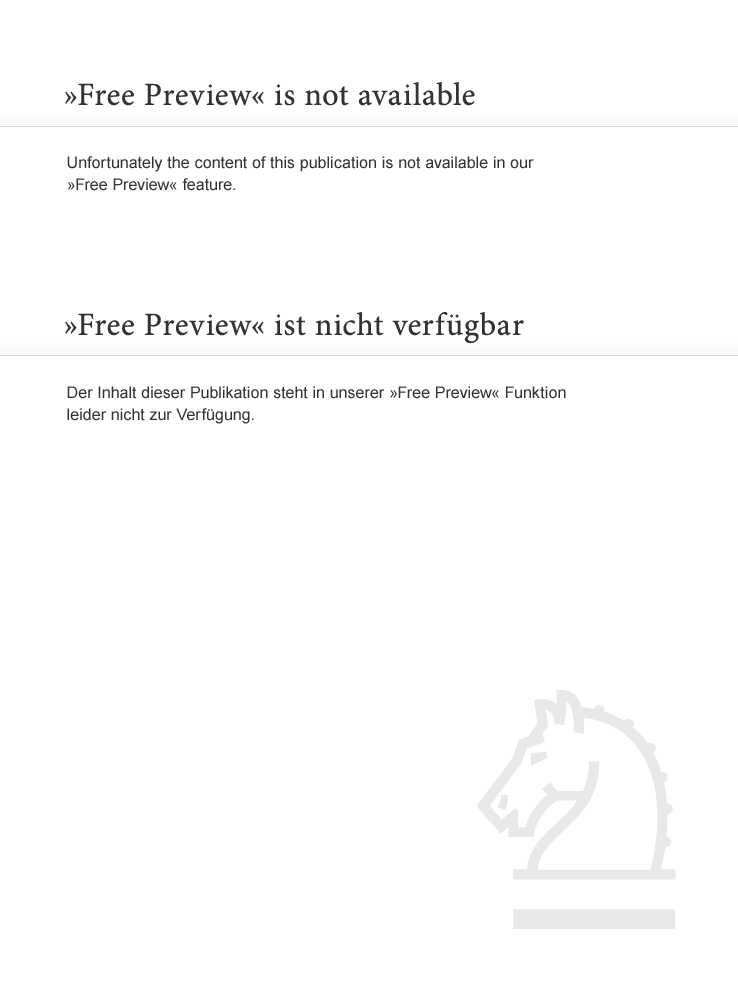
Bayesian Financial Methods
Bayesian Financial Methods
Thomas Bayes was an English mathematician and Fellow of the Royal Society of London. He lived in the 18th century and laid the foundations to probability theory. For a discussion of Bayesian test of portfolio efficiency and likelihood approach to low default portfolios see Kandel et al. (1995). Bayes Theorem (also called Bayes Law or Bayes Rule) of conditional probabilities, is widespread in finance:
P(A|B) = P(B|A)P(A)/P(B)
Geweke & Zhou (1996) discuss providing Bayesian background for experimenting with the Arbitrage Pricing Theory (APT), used to estimate the return on an investment. The postulate is tested with traditional factor analysis among other procedures.
Statistics can be inferential and descriptive, and statistical inference is designed to predict population parameters from sample statistics. Bayesian inference Using Gibbs Sampling (BUGS software package) can be used with Markov Chain Monte Carlo (MCMC) methods. Gibbs Sampler is a statistical method that refers to deriving a number using the probability distribution. Advances in computer science have solved the computational problem, since a solid knowledge of integrals is required.
Literature on BUGS and the Metropolis-Hastings algorithm is abundant (e.g. Arminger & Muthen, 1998; Billera & Diaconis, 2001; Chib & Jeliazkov, 2001). Surprisingly many free e-books are available for download on the net.
Bayesian econometrics is used in finance to determine option price, forecasting, market, credit and operational risk management, as well as portfolio allocation. The Bayesian framework is hospitable to return distribution, expected utility computation and Sharpe ratios.
Definition, condition and parameters are crucial in econometric modeling. For instance, the disparity between the money market (short-term borrowing and lending only) and the bond market (long-term investment and financing) could affect a computational outcome (Raible, 1998).
Wylie et al. (2006) sheds light on positivism, interpretivism and constructivism. Bayesian modeling quantifies how human information processing and decision-making work. An alternative construct is the frequentist methodology used in business and management studies. The meaning each method assigns to probability is dissimilar. Bayesianism places more emphasis on plausibility and uncertainty.
Because the condition is the new information, or a prior, these have been classified into three categories: informative, noninformative and hierarchical.
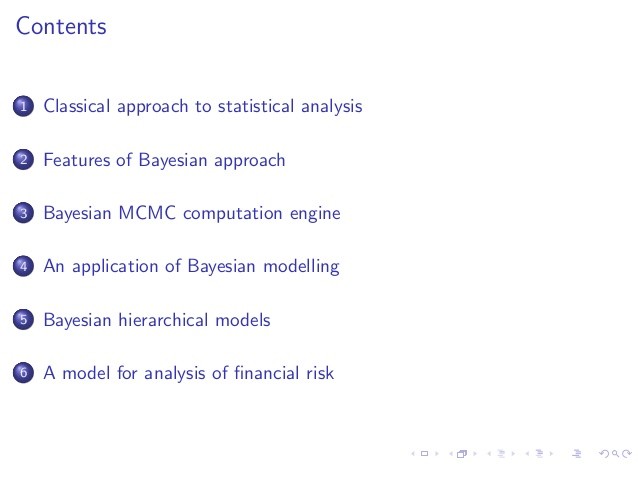
Bayesian Belief Networks (BBNs) are models to measure operational risk as depicted by Alexander (2000). Bayesian analysis can be applied to human risk. Human risk (losses due to staffing deficiencies) is part of operational risk management.
Bayesian learning is the method of selecting the best hypothesis (ex. nave Bayes learner). Geweke & Tanizaki (2001) narrate such topics as Bayes estimator, non-Gaussian and/or Bayesian state space models and rejection sampling as well as their usefulness in finance for market and portfolio analysis.
References:
- Alexander, C. (2000). Bayesian methods for measuring operational risk. Discussion Papers in Finance. Social Science Research Network (SSRN).
- Arminger, G. & Muthen, B. O. (1998). A Bayesian approach to nonlinear latent variable models using the Gibbs Sampler and the Metropolis-Hastings algorithm. Psychometrika. 63 (3): 271-300.
- Billera, L. J. & Diaconis, P. (2001). A geometric interpretation of the Metropolis-Hasting Algorithm. Statistical Science. 16 (4): 335-339.
- Chib, S. & Jeliazkov, I. (2001). Marginal likelihood from the Metropolis-Hastings output. Journal of the American Statistical Association. 96 (453): 270-281.
- Geweke, J. & Tanizaki, H. (2001). Bayesian estimation of state-space models using the Metropolis-Hastings algorithm within Gibbs sampling. Computational Statistics & Data Analysis. 37 (2): 151-170.
- Kandel, S. McCulloch, R. & Stambaugh, R.F. (1995). Bayesian inference and portfolio efficiency. Review of Financial Studies. 8 (1): 1-53.
- Wylie, J. Muegge, S. &Thomas, R.D. (2006). Bayesian methods in management research: An application to logistic regression. Administrative Sciences Association of Canada (ASAC). 1-17.
Bayes Theorem formulas an intuitive idea: we adjust our perspective (the probability set) given new, relevant information. Formally, Bayes Theorem helps us move from an unconditional probability (what are the odds the economy will grow?) to a conditional probability (given new evidence, what are the odds the economy will grow?). Youtube, uploaded by bionicturtledotcom