The Statistics of Sharpe Ratios
Post on: 8 Май, 2015 No Comment
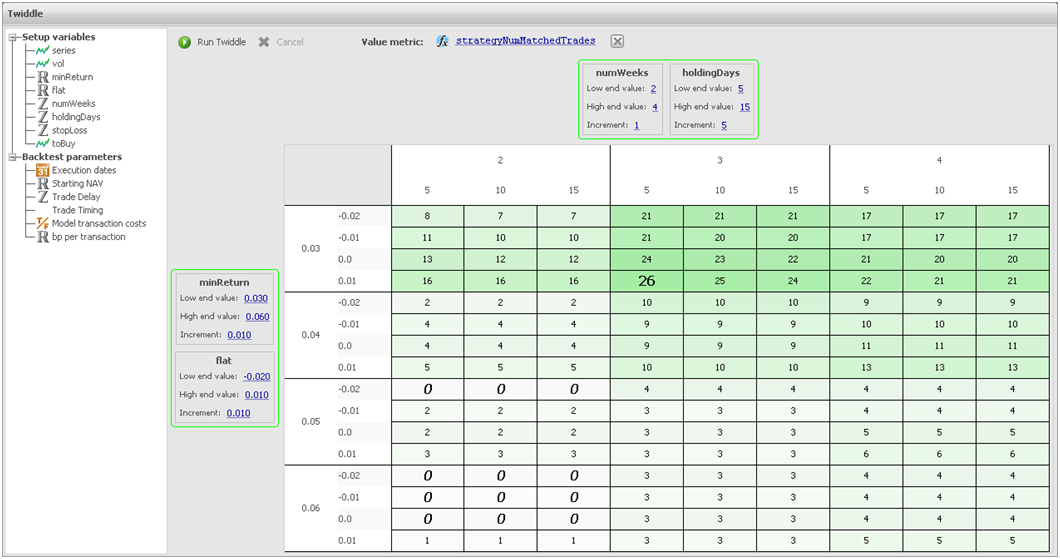
Page 1
©2002, AIMR®
The Statistics of Sharpe Ratios
Andrew W. Lo
The building blocks of the Sharpe ratio—expected returns and volatilities—
are unknown quantities that must be estimated statistically and are,
therefore, subject to estimation error. This raises the natural question: How
accurately are Sharpe ratios measured? To address this question, I derive
explicit expressions for the statistical distribution of the Sharpe ratio using
standard asymptotic theory under several sets of assumptions for the
return-generating process—independently and identically distributed
returns, stationary returns, and with time aggregation. I show that
monthly Sharpe ratios cannot be annualized by multiplying by
under very special circumstances, and I derive the correct method of
conversion in the general case of stationary returns. In an illustrative
empirical example of mutual funds and hedge funds, I find that the annual
Sharpe ratio for a hedge fund can be overstated by as much as 65 percent
because of the presence of serial correlation in monthly returns, and once
this serial correlation is properly taken into account, the rankings of hedge
funds based on Sharpe ratios can change dramatically.
except
ne of the most commonly cited statistics in
financial analysis is the Sharpe ratio, the
ratio of the excess expected return of an
investment to its return volatility or standard devi-
ation. Originally motivated by mean–variance
analysis and the Sharpe–Lintner Capital Asset Pric-
ing Model, the Sharpe ratio is now used in many
different contexts, from performance attribution to
tests of market efficiency to risk management.1
Given the Sharpe ratio’s widespread use and the
myriad interpretations that it has acquired over the
years, it is surprising that so little attention has been
paid to its statistical properties. Because expected
returns and volatilities are quantities that are gen-
erally not observable, they must be estimated in
some fashion. The inevitable estimation errors that
arise imply that the Sharpe ratio is also estimated
with error, raising the natural question: How accu-
rately are Sharpe ratios measured?
In this article, I provide an answer by deriving
the statistical distribution of the Sharpe ratio using
standard econometric methods under several dif-
ferent sets of assumptions for the statistical behav-
ior of the return series on which the Sharpe ratio is
based. Armed with this statistical distribution, I
show that confidence intervals, standard errors,
and hypothesis tests can be computed for the esti-
mated Sharpe ratio in much the same way that they
are computed for regression coefficients such as
portfolio alphas and betas.
The accuracy of Sharpe ratio estimators hinges
on the statistical properties of returns, and these
properties can vary considerably among portfolios,
strategies, and over time. In other words, the
Sharpe ratio estimator’s statistical properties typi-
cally will depend on the investment style of the
portfolio being evaluated. At a superficial level, the
intuition for this claim is obvious: The performance
of more volatile investment strategies is more dif-
ficult to gauge than that of less volatile strategies.
Therefore, it should come as no surprise that the
results derived in this article imply that, for exam-
ple, Sharpe ratios are likely to be more accurately
estimated for mutual funds than for hedge funds.
A less intuitive implication is that the time-
series properties of investment strategies (e.g.,
mean reversion, momentum, and other forms of
serial correlation) can have a nontrivial impact on
the Sharpe ratio estimator itself, especially in com-
puting an annualized Sharpe ratio from monthly
data. In particular, the results derived in this article
show that the common practice of annualizing
returns—can yield Sharpe ratios that are consider-
ably smaller (in the case of positive serial correla-
Using a set of techniques collectively known as
“large-sample’’ or “asymptotic’’ statistical theory
in which the Central Limit Theorem is applied to
estimators such as and
and other nonlinear functions of
in large samples
data, a more general distribution is derived in the
“Non-IID Returns” section, one that applies to
returns with serial correlation, time-varying condi-
tional volatilities, and many other characteristics of
historical financial time series. In the “Time Aggre-
gation” section, I develop explicit expressions for
“time-aggregated’’ Sharpe ratio estimators (e.g.,
expressions for converting monthly Sharpe ratio
estimates to annual estimates) and their distribu-
tions. To illustrate the practical relevance of these
estimators, I apply them to a sample of monthly
mutual fund and hedge fund returns and show that
serial correlation has dramatic effects on the annual
Sharpe ratios of hedge funds, inflating Sharpe ratios
by more than 65 percent in some cases and deflating
Sharpe ratios in other cases.
IID Returns
To derive a measure of the uncertainty surrounding
the estimator. we need to specify the statistical
properties of Rt because these properties determine
the uncertainty surrounding the component estima-
tors and. Although this may seem like a theo-
retical exercise best left for statisticians—not unlike
the specification of the assumptions needed to yield
well-behaved estimates from a linear regression—
there is often a direct connection between the invest-
ment management process of a portfolio and its
statistical properties. For example, a change in the
portfolio manager’s style from a small-cap value
orientation to a large-cap growth orientation will
typically have an impact on the portfolio’s volatility,
degree of mean reversion, and market beta. Even for
a fixed investment style, a portfolio’s characteristics
can change over time because of fund inflows and
outflows, capacity constraints (e.g. a microcap fund
that is close to its market-capitalization limit),
liquidity constraints (e.g. an emerging market or
private equity fund), and changes in market condi-
tions (e.g. sudden increases or decreases in volatil-
ity, shifts in central banking policy, and
extraordinary events, such as the default of Russian
government bonds in August 1998). Therefore, the
investment style and market environment must be
kept in mind when formulating the assumptions for
the statistical properties of a portfolio’s returns.
Perhaps the simplest set of assumptions that we