Journal of Derivatives & Hedge Funds Value at risk GARCH modelling and the forecasting of hedge
Post on: 12 Июнь, 2015 No Comment
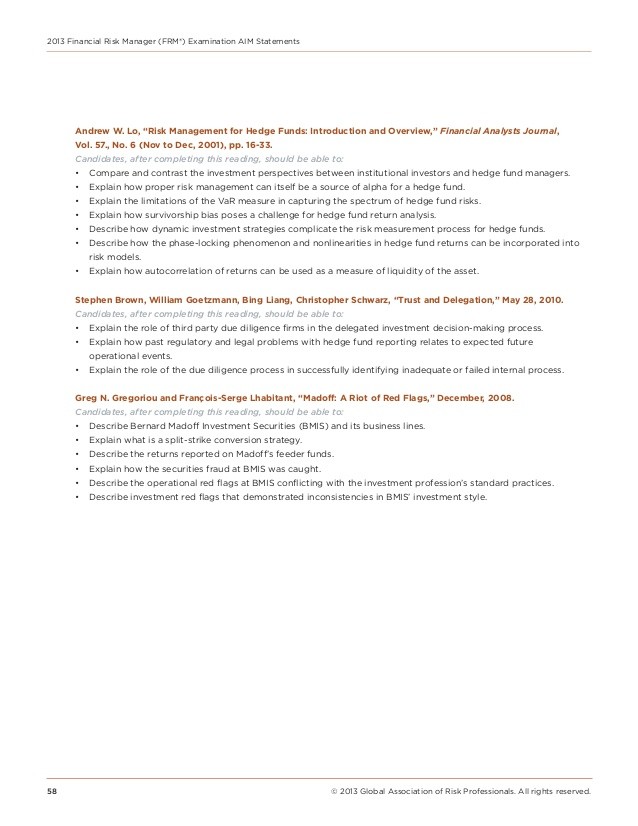
Journal of Derivatives & Hedge Funds (2007) 13, 225. doi:10.1057/palgrave.jdhf.1850048
Value at risk, GARCH modelling and the forecasting of hedge fund return volatility
Practical applications
The Value at Risk (VaR) approach is widely used within the asset and risk management of traditional and alternative investments. From the investor’s point of view, an adequate VaR model should indicate how the positions of the hedge fund portfolio should be sized for the best protection against downside risk. Due to the skewness and excess kurtosis of daily financial return distributions, the normal VaR has its drawbacks particularly when it is applied to hedge funds. In addition to the CornishFischer VaR, which explicitly accounts for non-normally distributed returns, we examine the conditional volatility characteristics of daily hedge fund management style returns. The inclusion of time-varying conditional volatility into the VaR measurement enables us to trace the actual return process more effectively. We show that such a GARCH-type VaR is a superior measure of downside risk, especially for trading strategies that exhibit negative skewness and excess kurtosis and offer daily pricing.
Roland Fss 1. Dieter G Kaiser 2 and Zeno Adams 3
Correspondence: Roland Fss, Department of Empirical Research and Econometrics, University of Freiburg, Platz der Alten Synagoge, Freiburg im Breisgau, D-79085, Germany. Tel: +49 0 761 203 2341; Fax: +49 0 761 203 2340; E-mail: roland.fuess@vwl.uni-freiburg.de
1 Dr Roland Fss is Lecturer at the Department of Empirical Research and Econometrics and Assistant Professor at the Department of Finance and Banking at the University of Freiburg, Germany. He holds an MBA from the University of Applied Science in Lrrach, M.Ec. and PhD degree in Economics from the University of Freiburg. His research interests are in the field of applied econometrics, alternative investments as well as international and real estate finance. He has (co-) authored several articles in finance journals and book chapters. Further, he is a member of the Verein fr Socialpolitik and of the German Finance Association.
2 Dieter G. Kaiser is responsible for the institutional research at Benchmark Alternative Strategies in Frankfurt, Germany. He has worked for Dresdner Kleinwort Wasserstein and Crdit Agricole Asset Management in Frankfurt where he was responsible for the fund-of-hedge-funds Marketing Support. He has written several articles on the subject of alternative investments that have been published in professional as well as academic journals. He is the co-author and co-editor of five books on alternative investments published by John Wiley & Sons, Risk Books and Gabler. He holds a Diploma in Business Administration and a Master of Arts in Banking & Finance from the HfB — Business School of Finance and Management in Frankfurt, where he has also lectured on alternative investments since 2003.
3 Zeno Adams is Research Assistant at the Department of Empirical Research and Econometrics, University of Freiburg, Platz der Alten Synagoge, D-79085 Freiburg im Breisgau, Germany.
Received 29 August 2006; Revised 29 August 2006.
Abstract
This paper examines the conditional volatility characteristics of daily management style returns and compares the out-of-sample forecasts of different Value at Risk (VaR) approaches, namely, the normal, CornishFisher (CF), and the so-called GARCH-type VaR. The examination of the conditional volatility of hedge fund styles and composite returns shows important differences concerning persistence, mean reversion and asymmetry in the period under consideration. Hedge fund returns exhibit significant negative skewness and excess kurtosis, which cannot be captured in the normal VaR whereas the CF-VaR results in a systematic downward shift of the conventional VaR. The GARCH-type VaR, however, includes the time-varying conditional volatility and is able to trace the actual return process more effectively. Since the forecast performance cannot detect which of the three VaR types can match the time-varying risk adequately, an adjusted hit ratio takes the size of the hits as well as the average VaR into account. According to this, the GARCH-type VaR outperforms the other VaRs for most of the hedge fund style indices.
Keywords:
hedge funds, Value at Risk, GARCH models, forecasting
INTRODUCTION
Since the breakdown of Long Term Capital Management (LTCM), risk management and the transparency of hedge funds have become dramatically important and evolved into outstanding fields for practitioners and academic researchers. Value at Risk (VaR) is one of the most important concepts widely used for risk management by banks and financial institutions. Since VaR can be easily computed by capturing risk in only one figure, it has gained increasing popularity in the past. Although there are several forms of financial risk, we focus on market risk in this paper, that is, the unexpected changes in stock returns.
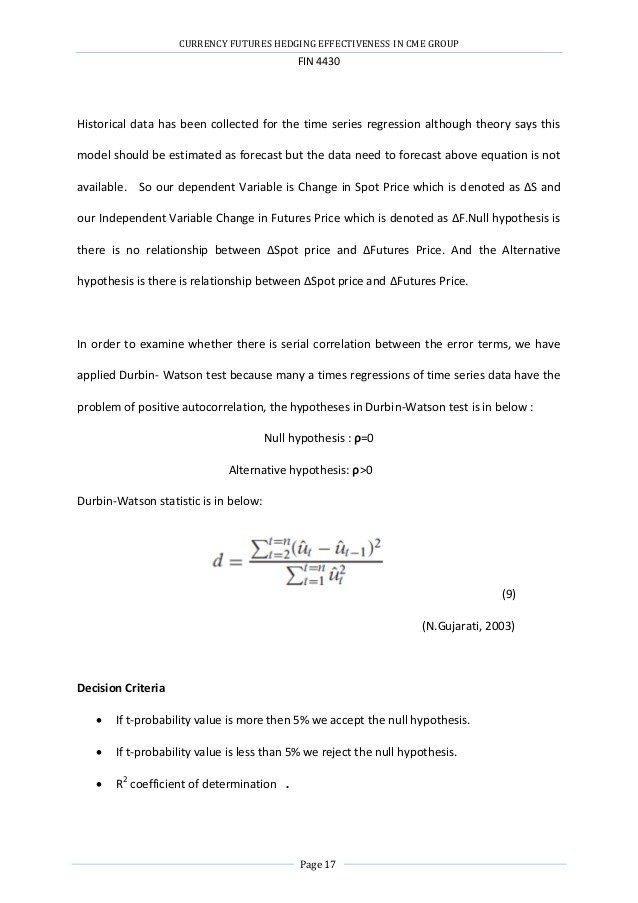
The literature on VaR has become quite expansive (eg Hendricks 1. Beder 2. Marshall and Siegel 3 ). However, the conventional VaR assumes that returns follow a normal or conditional normal distribution. Particularly, in the case of skewed and fat-tailed returns, the assumption of normality leads to substantial bias in the VaR estimation and results in an underestimation of volatility.
In contrast to mutual funds, different trading instruments, such as arbitrage, leverage and short selling, characterise hedge funds. These trading instruments are highly dynamic and often exhibit low systematic risk (Fung and Hsieh 4 ). Since hedge funds use options or option-like trading strategies or strategies that lose money during down-market phases, they may generate non-normal payoffs. In addition, Liang 5 emphasises the higher Sharpe ratios and lower market risk as well as the higher abnormal returns of hedge funds investments. Moreover, it has been well documented that monthly return distributions of most hedge fund indices show extremely high negative skewness, positive excess kurtosis and, significantly, positive first-order serial correlation. In the context of the frequently used meanvariance approach, these return properties inevitably result in an underestimation of the true volatility.
Favre and Galeano 6 suggest a modified method of VaR by implementing a CornishFisher (CF) expansion, which is used to control for skewness and kurtosis. 7 Agarwal and Naik 8 introduce a mean-conditional VaR (CVaR) framework that explicitly accounts for negative tail risk. 9 As the conventional VaR refers only to the frequency of extreme events, the CVaR focuses on both frequency and size of losses in the case of extreme events. Kellezi and Gilli 10 introduce a risk capital measure based on the Extreme Value Theory (EVT). The EVT focuses only on extreme values, that is, the tail of the distribution, rather than the whole distribution. However, Danielsson and de Vries 11 show that the EVT can be accurately used only for very extreme events and often does not provide good results at more conventional 5 per cent VaR levels. Furthermore, EVT assumes an identically and independently distributed (iid) framework that is not consistent with most financial data.
In this paper, we use a GARCH-type VaR by modelling and forecasting conditional volatility, using GARCH and EGARCH, and then implementing the time-varying volatility in the VaR. In doing so, we also control for skewness and kurtosis. Volatility forecasting is important not only in risk management and market timing for single hedge funds, but also in the context of portfolio diversification including hedge funds. The knowledge of future volatilities allows portfolio managers to control the risk temporally, for example, sell an asset or portfolio before a dramatic increase in volatility takes place (Engle and Patton 12 ). Furthermore, by means of information on the volatility process in general, and the development of volatility in particular, the risk pricing of the market can be determined.
To our knowledge, there are no empirical studies that introduce GARCH-type forecasts into the conventional VaR framework to simultaneously account for time-varying volatility, serial correlation, skewness and kurtosis in hedge fund returns.
This paper is organised as follows: Firstly, the next section describes different hedge funds strategies from the data provider, Standard &Poor’s. according to the different management styles. The concepts of conventional VaR and CF expansion are then briefly introduced. Following this, the stylised facts of volatility and the two conditional volatility models, GARCH(p ,q ) and EGARCH(p ,q ), that should capture these features are discussed. Subsequently the conditional variances for the hedge funds styles under consideration are estimated using alternative model specifications, and their volatility characteristics are analysed. The GARCH-type models are then applied to estimate the daily VaR of the different hedge fund styles. The accuracy of one-step-ahead VaR forecasts is evaluated by different ratios that measure the distance between the observed and forecasted VaR values. Some concluding remarks are offered in the final section.
HEDGE FUNDS STYLES AND THEIR STRATEGIES
Investment strategies used by hedge funds tend to be quite different from those of traditional mutual funds. Basically, every hedge fund embarks on its own preferred strategy, which leads to a very heterogeneous asset class ‘hedge funds’. However, hedge funds may be classified into a number of different strategy groups depending on the main type of strategy followed (Kat and Lu 13 ). In referring to market exposure as classification criteria, Purcell and Crowley 14 distinguish between three different styles of hedge funds. These broader categories encompass the relative value, the event-driven and the opportunistic strategy. Based on market exposure to traditional asset classes, Agarwal and Naik 15 also classify hedge fund strategies into categories: directional and non-directional. Ineichen 16 conducts a subdivision on the hedge funds styles: arbitrage, event-driven and directional (see Figure 1 ). In this study, we adapt the classification proposed by the latter to match the system of the hedge funds index provider S&P, as we use their indices later in the empirical section of this paper.