Forecast model
Post on: 16 Март, 2015 No Comment
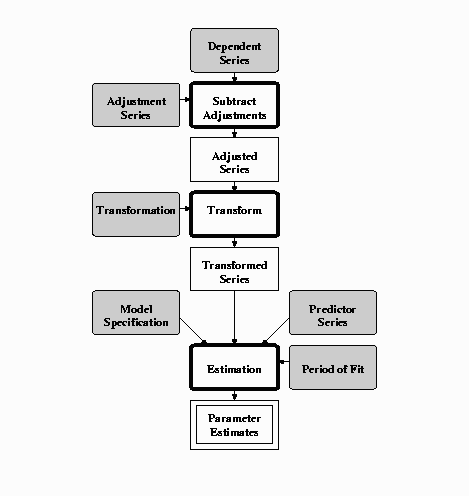
ANN exploits the full capacity of the brain
Artificial neural networks, ANN, is a system created to mimic neural ability to process information. Practically all knowledge which people acquire during their lives is created by neurons making statistical analyses of information from the senses.
Information processing in this way is decentralised and each neuron works independently on analysing the information in its immediate surroundings, but by virtue of its structure also assists the other neurons in processing information. The result is a highly robust statistical analysis, which can simultaneously process enormous quantities of information and extremely complex connections.
More than state-of-the-art within research
Calejo Business Intelligence develops its products together with people at the very forefront of the field of research and tries to break through the line. The company has developed the algorithms beyond the most recent scientific articles and specifically adapted them for specially selected markets. This enables us to circumvent the problem of choosing variables by allowing the networks themselves to select what is relevant and also partially use correlated independent variables without predictions as a whole being disturbed.
Methods and sources of error
Calejo’s model identifies patterns of historical price evolution and its fundamental factors. These are then used for forecasting future prices.
An artificial neural network finds trends in historical data and makes extremely accurate forecasts of price evolution for the forthcoming 10 to 30 months. All purely statistical models have one main source of error: models are designed to fit historical evolution are all too specifically, instead of finding patterns and being representative of the future. Unfortunately it is more the rule than the exception for financial forecast models not to deal with this source of error. Often their historical accuracy is based entirely on the models suiting the history and the results thus obtained are completely illusory.
Calejo compensates for this using an automatic preventive method. This so-called regularisation automatically prevents the model from identifying unlikely and all too detailed patterns in price movements. This, together with the flexibility of neural networks, results in extremely good forecasting ability. Networks without regularisation have outperformed competing financial models in almost all scientific studies of real markets.
In order to evaluate the model we then carry out an extremely strict test – cross validation. This involves the model being forced to identify price changes from a number of time periods entirely on its own and then evaluating this pattern for a separate time period. This process is repeated with various combinations of time periods for identifying patterns and for evaluation. Therefore, the result is expected to be completely representative for future forecasts as well.
Competing alternatives are based on extremely simple linear models without regularisation or on differential equations, the forecasting ability of which lack scientific basis and probably have no forecasting capacity. In general, they have not be tested in a structured manner.
The Market and competition
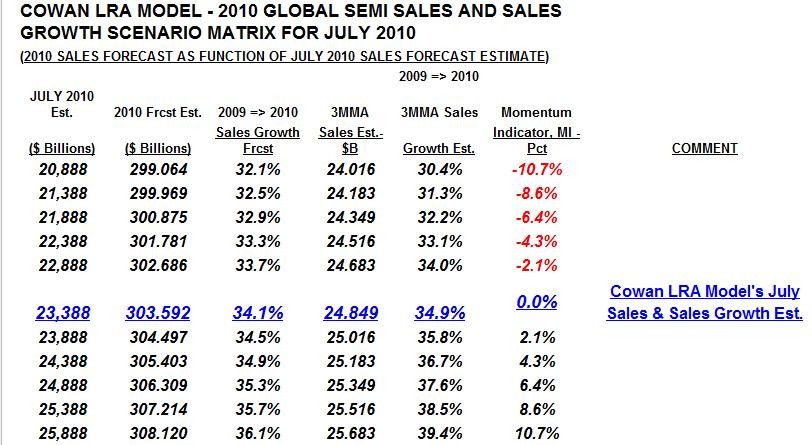
The human being has a poor ability to generalise and predict the future. Various scientific studies show that simple statistical methods almost always exceed the human analyst’s and expert’s predictions or diagnoses. There are many reasons for this, one of which is that analysts often base their conclusions on simple rules of thumb. Moreover, these rules of thumb seldom or never are validated against historical data.
In a number of published research studies, neural networks have been compared with linear models, ARIMA models, human analysts etc. These studies clearly show that, neural networks are superior to other known models, especially in the longer term. Even in applications which are not financial in nature, neural networks prove to be the most successful of self-learning algorithms with countless applications within industry and research. For example, the most efficient known algorithm for automatic classification of text is a type of ANN.
Simpler forecast models based on Artificial Neural Networks (ANN) are much used today as forecast tools within the currency and equity markets, but judging by the published documentation they are rough hewn. For forecasting prices of commodities, industry is still forced to turn to human and so-called technical regression analyses. Due to the long-term prospects, historical series are not long enough to use neural networks without regularisation, a unique method which is today still extremely unusual and no competition is expected within the commodity market.
The tool Calejo offers to industry is entirely unique and revolutionary.
- Improved knowledge of the future creates greater stock and trading profits.
- Better insight and knowledge also helps corporate managements to plan organisational changes, take restructuring measures, make purchases and investments in good time.
Savings are considerable.