TraderFeed Prediction and Understanding in Generating Market Returns
Post on: 16 Март, 2015 No Comment
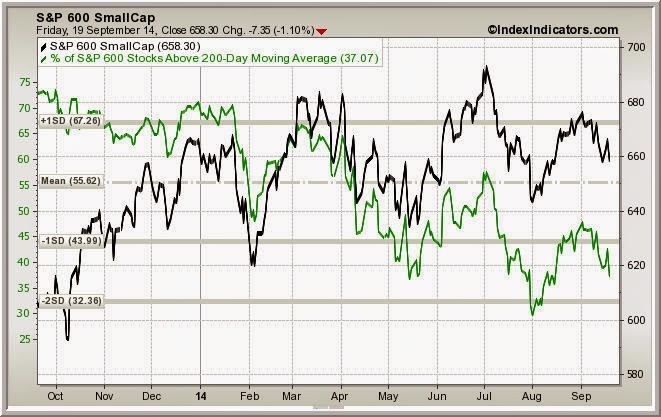
Prediction and Understanding in Generating Market Returns
The recent series of posts have reviewed a number of stock market measures that I have found to be useful in anticipating short-term price movement: buying and selling pressure ; pure volatility ; breadth ; sentiment ; and correlation. The question now arises: how do we make use of multiple measures such as these? How do we put them together in a coherent fashion?
Let’s address this by first surveying the territory. Traders attempt to anticipate market behavior by engaging in one of four modes: 1) bias; 2) superstition; 3) prediction; and 4) understanding.
Bias represents a way of thinking that is grounded, not in objective events as they are occurring, but in thoughts, feelings, and ways of processing information that are influenced by external and nonessential factors. For example, I have met a number of traders who display a persistent bearish bias. When asked for the reasons for their bearishness, they cite their disagreement with the political and economic policies of the government. Their political and economic beliefs are so strong that they cannot see markets through any other lens. Needless to say, this has led to disastrous returns over the long run.
Superstition is a set of beliefs that are not grounded in objective observation and measurement and—this is important—cannot be validated through such means. A while back I encountered a market analyst who declared that the stock market was headed lower because it was completing a head-and-shoulders pattern on the weekly chart. I quickly coded up market patterns of a similar nature and backtested the idea. I found no predictive validity to the head-and-shoulders formation whatsoever. I varied the coding to look at formations on different time frames and still found no predictive value to the pattern. When I explained this to the analyst, he replied that technical analysis was an art, not a science. The belief system that shapes on charts dictate forward price movement was grounded in superstition and—as a supposed art—was immune to attempts at validation.
Prediction is an attempt to quantify and systematize relationships among variables that we believe to be causal. Suppose I were to take the variables listed above, such as sentiment and volatility, and enter them into a stepwise regression equation to see which uniquely predict forward price movement. I might find, for instance, that one of the variables—say volatility—so overlaps with correlation and buying/selling power that it does not add significant predictive value whatsoever. The variables that do add significant predictive value comprise a model that spits out predictions of future price movement. While much prediction is quantitatively driven, a qualitative form of prediction occurs when experienced traders recognize recurrent market patterns and base quick decisions on the occurrence of these patterns. Because such intuitive pattern recognition can look so much like superstition, it can be challenging to differentiate skilled intuitive discretionary traders from superstitious amateurs. Ultimately, the arbiter of predictive value—whether quantitative or discretionary—is an objective, real-time track record.
Understanding is quite different from prediction. We can predict when the sun will rise in the morning, but not necessarily understand the reasons for the sun’s apparent movement. Understanding addresses the why behind prediction. Very often, we do not know precisely how causes lead to effects in nature. We develop our understanding by generating a theory, which attempts to explain how variables of interest lead to observed outcomes. Good theories account for existing findings, but also suggest new ones. One pitfall in the investing world is generating complex predictive models through means such as neural networks. The output of these models may not be clear—and certainly may not reflect any understanding of the underlying markets. A very useful brake on the overfitting of trading systems and models is the use of input variables that make sense within a plausible theoretical model. Otherwise, we have no way of determining whether our predictive model is truly grounded in an understanding of the world.
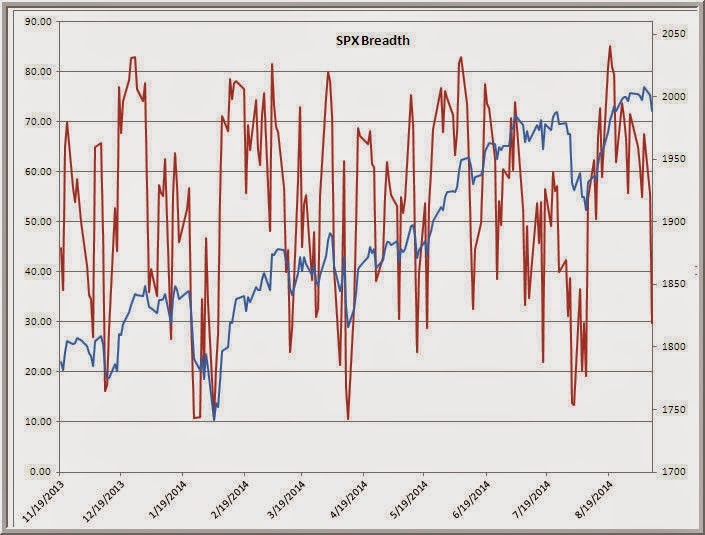
In important respects, the division between technical analysis and fundamental analysis in the trading world reflects a tension between the modes of prediction and understanding. Just as the predictions of technicians can reflect little underlying understanding of markets, the inputs of fundamental analysts can lack predictive rigor. A good example of the former is market queries that find a predictive edge when, say, we post a three-day high at the start of a calendar period. There is no proposed mechanism that explains why such a pattern would hold true. Conversely, when we hear a macro analyst declare that stock market returns will be dampened in Europe because of threats of war in the Ukraine, there is no predictive framework for testing and validating the assertion.
Ultimately we master a domain when we can explain observations and validate our explanations through tests of our predictions. Those tests either occur prospectively, as part of formal backtesting and forward testing, or they occur in retrospect via the analysis of real-time track records. Such mastery is rarely demonstrated in trading, where a great deal of activity is dominated by bias, superstition, and disjointed efforts at prediction and explanation. As a psychologist and trader for many years, my distinct impression is that much of what traders experience as emotional challenges in markets is the result of operating in an uncertain domain with less-than-adequate predictive and explanatory frameworks. Anxiety and frustration are the understandable consequence of needing to predict and understand, but having few tools to achieve those ends.
So what does a sound explanatory and predictive framework look like? One window on market success is offered via the Principles written by Ray Dalio of Bridgewater Associates, as well as his account of How the Economic Machine works. Another window on successful prediction and explanation can be found in the work of Clifford Asness of AQR, including his published papers. The dramatic success of these funds represents the power of joining prediction and explanation in a robust and replicable fashion. That is the hallmark of science.
This brings us back to the original question: Is there an explanatory framework that can bring together the variables covered in the recent blog posts? And what is the proper role of intuition in the joining of prediction and understanding? Those will be the topics of the next posts in this series.