Gaussian Estimation of OneFactor Mean Reversion Processes
Post on: 16 Март, 2015 No Comment
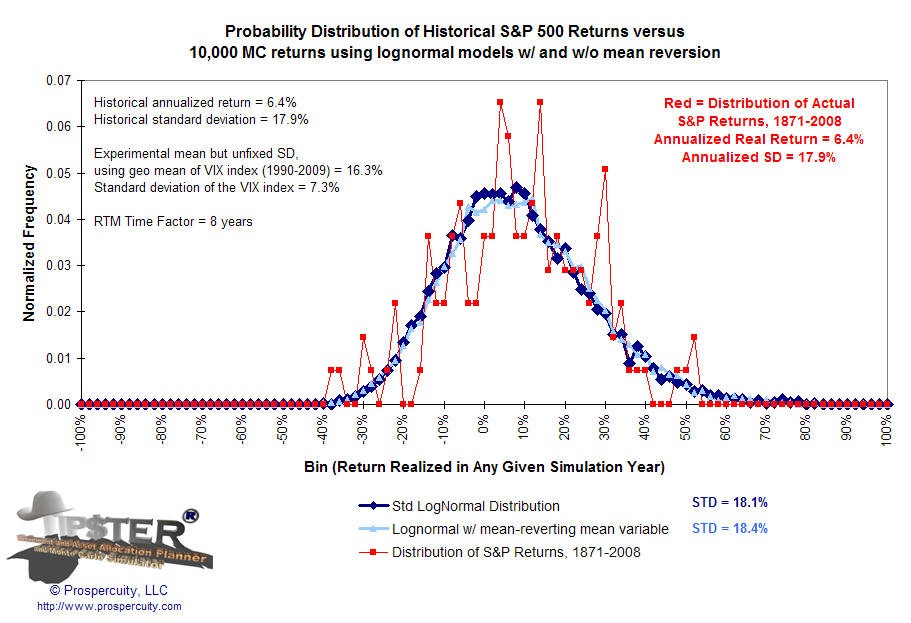
Gaussian Estimation of One-Factor Mean Reversion Processes
Basic Science Department, Eafit University, Carrera 49 No. 7 Sur 50, Medellin, Colombia
Received 25 April 2013; Accepted 28 August 2013
Academic Editor: Aera Thavaneswaran
Abstract
We propose a new alternative method to estimate the parameters in one-factor mean reversion processes based on the maximum likelihood technique. This approach makes use of Euler-Maruyama scheme to approximate the continuous-time model and build a new process discretized. The closed formulas for the estimators are obtained. Using simulated data series, we compare the results obtained with the results published by other authors.
1. Introduction
Different applications of stochastic differential equations have attracted the attention of many researchers in relation to the theoretical advances needed for modeling and simulation of the dynamic behavior of the main variables affecting the evolution of some phenomena. Applications in fields of economics, finance, physics, insurance, and others have been drivers of these developments. Particularly, through diffusion processes have been carried out modeling interest rates and commodity prices, where they used in their modeling processes mean reversion. Some trends of these studies are based on some a priori knowledge to determine the structure of the model to be used, while other alternatives are part of a general structure, and statistical procedures through the particular structure are determined to represent the phenomenon. Whichever approach is used, in general, in the specification of the model, it is necessary to estimate parameters to achieve a proper fit of the model.
Although some parameter estimation methods have been extensively studied in diffusion processes, this has a serious problem, which is that the specification of the models is done in continuous time but the data that is available for adjusting the parameters are discretely sampled in time. A common solution to this problem is to discretize the continuous-time model based on Euler-Maruyama scheme and perform procedures on the theoretical formulation resulting in discrete time. From this scheme alternatives can be proposed for parameter estimation approach among which stands out the maximum likelihood estimation.
Following the classification proposed by Yu and Phillips [1 ], different parameter estimation procedures can be classified globally into three categories according to how to proceed from the model formulation: discretization of continuous process and estimation of the parameters with the resulting discretized process, estimation of the transition probability function for the continuous-time model, and independent estimations of the trend and volatility components by kernel methods.
Thus, among the methods that are based on the discretization of the continuous-time model is Nowman [2 ], who considers a stochastic differential equation with two unknown variables in the drift term and two in the volatility, elasticity allowing the variance. Similarly, Yu and Phillips [1 ] present an improvement to the method proposed by Nowman [2 ], which is based on a nonuniform sampling of the observations, and the estimation process makes use of the equivalence of the methods of maximum likelihood and least squares under the conditions laid down in the model. Alternatively, Yu [3 ] proposes a new scheme that is based on the idea of transforming a stochastic differential equation (EDE) in its discrete-time equivalent, an
to determine the formulas that approximate the bias error present in the estimation of one of the parameters.
Some of the works that could be classified as methods in which it seeks to find the transition probability function and use Martingale properties to approximate the likelihood function are Durham and Gallant [4 ], Valdivieso et al. [5 ], and Elerian et al. [6 ]. Durham and Gallant [4 ] propose a scheme based on simulation. Establishing different model modifications, Pedersen [7 ] improves computational performance, and characteristics of the model are studied using simulated synthetic series. Koulis and Thavaneswaran [8 ] study combined martingale estimation functions for discretely observed interest rates models. They derive closed-form expressions for the first four conditional moments of the CIR model via Ito’s formula and for extended versions of the CIR model using the Milstein’s approximation and construct the optimal estimating functions. They also show that combined estimating functions have more information when the conditional mean and variance of the observed process depend on the parameter of interest. Valdivieso et al. [5 ] proposed a method for estimating the transfer function through the inversion of the characteristic function of the process and the use of Fourier transform. This methodology is developed for some particular cases of D-OU processes (Ornstein-Uhlenbeck processes with broken car distribution D). Elerian et al. [6 ] used simulation-based techniques for estimating the transition probability depending on the sample to incorporate auxiliary dummy observations. The parameters are obtained by simulation and optimization using numerical algorithms.
Methods based on kernel techniques approximate density functions of the components and spreading tendency of the process. The works of Jiang and Knight [9 ] and Florens-Zmirou [10 ] are within this framework.
In this paper, we propose an alternative scheme for parameter estimation in one-factor mean reversion models, using the Euler-Maruyama discretization. Using the properties of discretized error normality, the maximum likelihood technique to obtain closed formulas for the estimators applies. We present some performance measures of the maximum likelihood estimators obtained with the proposed method in simulated processes, and we compare them with the results published by Nowman [2 ], Yu and Phillips [1 ], and Durham and Gallant [4 ].
This paper is organized as follows. In Section 2. a brief description of mean reversion processes of one factor with constant parameters. Some Gaussian estimation techniques are presented in Section 3. Section 4 develops the proposed method, and Section 5 presents some experimental numerical results with different sampling rates.
2. Mean Reversion Processes
Mean reversion processes have been considered as a natural choice to describe the dynamic behavior of interest rates in Vasicek [11 ], Brennan and Schwartz [12 ], Cox et al. [13 ], and Chan et al. [14 ]; daily prices of some commodities such as oil and natural gas in Lari-Lavassani et al. [15 ] have a fundamental role in modeling spot prices when they exhibited long-term trends, such as electrical energy (Pilipovic [16 ]).
Mean reversion processes of one factor with constant parameters can be written as