QUANTEXT monte carlo FAQ
Post on: 17 Август, 2015 No Comment
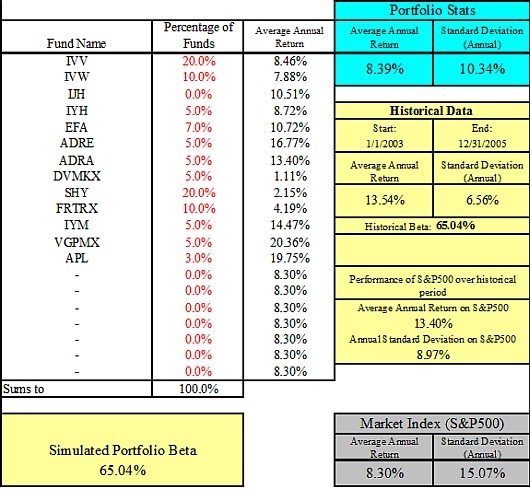
Quantext’s Frequently Asked Questions on Monte Carlo simulations for Retirement Planning
1. What is Monte Carlo? Monte Carlo simulations use historical statistics and mathematical models for the variability in returns on the market and various asset classes as well as individual stocks to forecast the range of possible outcomes associated with a saving, investment, asset allocation, and income plan. Monte Carlo simulations account for the correlation between different assets and asset classes in calculating total portfolio risk. Monte Carlo simulations actually generate hundreds of possible futures and then allow you to look at the probability of funding a specific income goal, running out of money, becoming very wealthy, etc. This approach is called Monte Carlo because it involves using the computer to generate many rolls of the dice (i.e. random numbers) to simulate many possible futures. This does not mean that these models assume that the future is random—just that some component of the future is random. The challenge is coming up with the statistics to describe the magnitude of random drivers for various investments and also the relationships between the random drivers for the diverse investments in a portfolio.
Monte Carlo models are used extensively in science and engineering applications, as well as in finance. Many situations that people want to understand and predict can only be properly understood in statistical or probabilistic terms. Portfolios of risky investments are one such situation. Monte Carlo models are standard for risk management in many industries but they have been slow to catch on for portfolio management for individuals.
2. Why is Monte Carlo useful or important for retirement planning?
Monte Carlo allows you to develop a financial plan that accounts for risk. It will show, for example, whether your investments yield a fairly stable portfolio or a high risk portfolio. It is remarkable that in this day and age, most investors (even those who are well off) have no solid sense of how risky their portfolios are. In Quantext’s Monte Carlo models, we calculate the probability that you will run out of money at some age, given your projected retirement age and income, current portfolio, future savings, portfolio allocation and other factors. The probability of running out of money by a certain age in retirement is a very solid risk metric and one that is important for planning. The Monte Carlo model allows the user to tailor the portfolio to achieve a risk-return balance that is right for them. If you have a portfolio with very high risk and return, such as being heavily invested in technology, you can grow a portfolio fast but you can also lose a lot fast. On the other hand, if you are too conservative, you have low volatility in your portfolio but will not generate enough return to meet your retirement needs. Our Monte Carlo model allows you to vary the allocation between bond funds, stock funds, and even individual stocks to examine the overall impact on retirement plans.
Simple ‘calculators’ that just assume an average rate of return and project forward carry a dangerous flaw. First, they do not show the effects of risk. Second, they cannot help you to figure out an appropriate allocation across assets. These simple projections will tend to give the user a false sense of security because risk is not accounted for. In essence, these calculators make all market investments look risk-free, which of course they are not.
By way of example, the chart below shows a hypothetical case for a 40-year old who is investing for retirement. He has $100K in his portfolio and it is invested in a conservative portfolio. The chart shows the evolution of the value of his portfolio with his age. The heavy line shows what this wealth accumulation will look like if we run a simple calculator that does not account for risk. He retires at age 65 and you can see that his portfolio evolution changes as he draws out each year. Assuming that he lives until age 90, he still has more than $1 Million in his portfolio using this approach. The Monte Carlo simulation generates all of the other possible portfolio outcomes, with each line showing the possible evolution of the portfolio, and we have shown only 30 of these paths but a real Monte Carlo model simulates hundreds. Each line is a portfolio outcome.
Some of the possible outcomes for this portfolio have its owner becoming quite well off. The problem, however, is that a fair number of these portfolios become completely depleted at ages much younger than the simple calculation based only on average rate of return. Notice that three of these possible outcomes (out of 30) do not even make it to age 85 and many more do not survive until age 90. Without using a Monte Carlo model, this person will have a false sense of security. The real problems come up if he does not use Monte Carlo and only has the simple solution (heavy line) and decides that he can actually draw a good bit more out of his portfolio.
Monte Carlo models can provide you with estimates of how much you can realistically lose or gain within a specific period of time—which can help you to determine whether your portfolio is within your comfort zone. To paraphrase Warren Buffett, you can determine if your portfolio is not so risky that it will keep you from sleeping at night. A good Monte Carlo model can also account for the contributions of stock options to your overall portfolio.
We have written a number of papers discussing asset allocation using Monte Carlo model—with one example looking at various risk-return outcomes for a portfolio built out of iShares ETFs.
There is another important reason to use Monte Carlo tools. Each investor needs to find a portfolio with the right level of risk and return that will give him or here the best odds of successfully funding retirement. If you are too conservative, you will not get the capital appreciation that you need to support your future income needs. If you are too aggressive, you can end up drawing income on a portfolio that has suffered major declines and thus endanger your ability to support future income. The best balance of equities and bonds, growth and value, etc. is a highly personalized analysis. Monte Carlo analysis is the right tool to help investors find the right balance. We have written a paper on this topic that also provides links to similar research by Alliance Bernstein.
3. How is Monte Carlo different or better than just looking at a long market history?
This question is very interesting. Many studies and projections for retirement planning look over some period in history to account for risk effects in planning. This is called historical analysis or backtesting (in the case of specific strategies) and is very useful. When you test a portfolio’s performance against history, no model is necessary. History is really our best information about the future when it comes to the markets. The parameters that drive Monte Carlo models come from historical data. The only problem with historical data is when you want to look at the risk associated with the long-term performance of a strategy. One of the most important examples of problems with historical data is the projection of ‘safe’ withdrawal rates from a retirement portfolio. Historical studies tend to suggest that with a portfolio of 60% stocks and 40% bonds you can take out about 4% of the value of your retirement portfolio at retirement (i.e. if you can take out an inflation adjusted $40,000 per year if you retire with $1,000,000, this is called a 4% withdrawal rate) each year and be 100% confident that your money will last for 30 years of retirement (see this discussion ). Monte Carlo studies tend to be more conservative, as the linked article shows. Our Retirement Planner model suggests that you would probably have a 10-15% chance of running out of money within 30 years. That said, the Monte Carlo is sensitive to the input assumptions, as is the historical analysis.
Monte Carlo models allow more flexibility in your assumptions, but they will give results that are consistent with historical analysis if you use inputs derived directly from history. If you are exploring the impact of a given allocation of your retirement portfolio into your company’s stock and your company is ten years old, Monte Carlo is very useful—pure historical analysis will not provide enough data. There are many other examples like this. Also, as your time horizon expands, it is more useful to apply Monte Carlo. If you have 20 or more years to retirement, and then you may be in retirement for 20-30 years, there is simply not enough pure historical data to allow you to explore a wide range of different scenarios.
Is there any reason to assume that the next 20 years will be just like the last 20 years? Many economists believe that we will see a market yielding closer to 8% than the 10+% per year that we saw in the last bull market. Monte Carlo models can account for this. If you have stock options, you can’t simply look at the past—you must project forward to value them and their contribution to your portfolio.
We have written a paper that shows how a Monte Carlo model differs from simply looking at market history. The paper is called The Writing on the Wall: What Portfolio Management Tools Can Tell You . The paper looks at how the Monte Carlo model looked at a portfolio of stocks during the bull market and how the simulation model provided very different perspectives on the optimal portfolio than simply looking at market history.
The bottom line is that the world changes. Market history informs the future, but a forward-looking perspective will combine recent data with long-term data on the risk and return associated with different asset classes.
4. Is Monte Carlo planning compatible with stock / fund selection?
This is one of the most interesting and least discussed topics in the Monte Carlo (hereafter called MC) portfolio planning literature. While most discussions look at broad classes of assets—such as investing in a stock index fund and a diversified bond portfolio—MC methods are extensively used in professional risk management for a portfolio of individual instruments. You may want to look at whether it makes sense to add individual stocks to your portfolio—and which ones. Our Portfolio Planner and Retirement Planner accomplish this task easily. For the more sophisticated investor, this is an interesting and productive pursuit—looking at how your individual stock selections work together in a portfolio. This is a more advanced topic than basic planning. We have written a number of articles on this issue that are available in our library . QPP and QRP analyze any combination of stocks, funds, and ETF’s and projects total portfolio risk and return. This is very useful information. We feel that this is much more relevant than simply talking about stocks as if they were a generic asset (like the proverbial 70% stock / 30% bond portfolio). In fact, the Pension Protection Act of 2006 specifically states that computer models for asset allocation should be able to handle a specific mix of real funds.
Many MC planning tools cannot account for a portfolio of individual stocks and funds properly. Some commercially available tools assume that any domestic individual stocks in your portfolio will perfectly track the S&P500, for example. Such a tool would not distinguish between investing in Boeing and investing in Yahoo!.
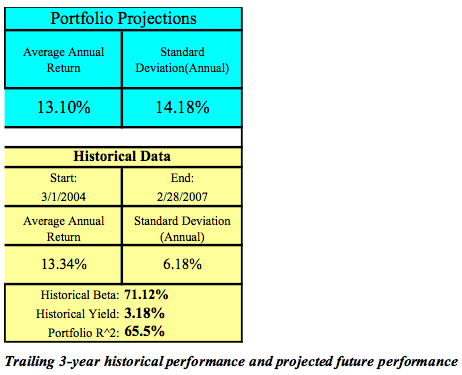
5. How long has this technology been around?
Monte Carlo models are computer simulations that use probability statistics to simulate many possible paths into the future. They have been around since World War II because that is when the first computers were developed that were capable of such fast computations. Monte Carlo modeling requires considerable computer power and the growth in computer power in the last decades has led to a vast growth in such simulations. Monte Carlo methods form the basis of modern risk management in financial applications. Geoff Considine has worked on financial applications of Monte Carlo for the last 15+ years for a range of large and medium sized firms. The last couple of decades has seen enormous growth in financial applications of Monte Carlo and this is a growth field—one in which we are actively involved. For personal portfolio management, Monte Carlo is becoming a standard. FinancialEngines.com was one of the pioneers in bringing this technology to the masses and have been doing so since 1996.
6. Where did the Quantext’s Monte Carlo model come from? Quantext developed the Monte Carlo model that drives the Quantext Retirement Planner and Quantext Portfolio Planner in 2004. We built this product because we perceived a need for a product and training materials to help people improve their own portfolio planning. A wide range of studies have shown that people simply do not have the right kinds of tools to plan effectively for retirement, and Quantext’s Planners are designed to meet this need. There are many papers about financial planning using this model, as well as comparisons to other Monte Carlo tools, and basic financial statistics in our library .
The Quantext Monte Carlo tools are designed to help people with personal portfolio management and allows for a wide range of different planning functions.
7. Why should I believe a Monte Carlo model’s statistical projections? This is a great question and one that is not asked often enough. If the value of Monte Carlo model is in accounting for uncertainty about the future, you want to have some confidence that the models have some rational basis for their projections of volatility. Monte Carlo models typically use historical data to develop statistical parameters and assume that the future will be somewhat like the past. Some models assume that the future will be just like the past 20-30 years (or some other period), in which case they generate results that are very similar to simply running a test portfolio against history. Better models tend to adjust the past statistics to be more general. One of the standard ways to do this in professional applications is to look at the way that the Monte Carlo model simulates the values of options on stocks and ETFs. The value of stock options is largely determined by the market’s consensus opinion on the future volatility of a stock or ETF. By using standard options models in our Monte Carlo models, we have tested to determine that our projected volatility for a range of stocks and ETF’s is consistent with long-term options trading in the market. See, for example, our paper on testing risk projections for index ETF’s. This type of analysis is called Mark-to-Market testing and it is standard in professional portfolio models to test this way, but not common in personal portfolio management applications. As far as we know, we are the first firm to provide Mark-to-Market testing and analysis of a Monte Carlo tool for personal portfolio management.
A more recent validation study of projected risk and return for an equity portfolio is shown here .
8. Are all Monte Carlo planning tools basically the same?
The answer to this is a resounding NO! While many articles about Monte Carlo planning tools discuss these simulations as though they were generic, different models will produce different results. Some of the other available commercial tools (aside from Quantext Monte Carlo tools) use what is called Style Analysis, which means that they do not explicitly model the individual components of your portfolio. Style Analysis assume that the return on a mutual fund or any other portfolio can be largely described by attributing the variations in return on that fund or portfolio in various measures to a set of ‘indices.’ In a Monte Carlo simulation, this means that you actually simulate a small set of indices and then describe the investor’s portfolio as being made up of a combination of these indices in various weights. This approach often works quite well for analyzing a portfolio of mutual funds but is not well suited for analyzing a portfolio of individual stocks. There is a very good review paper by Bill Sharpe (the Nobel Laureate in Economics and the a proponent of Style Analysis) here. Style analysis sometimes works well, and sometimes not, and there are statistical issues that make Style Analysis somewhat problematic on a forward-looking basis. Dr. Sharpe’s paper articulates these issues—pointing out a case in which Style Analysis can only explain 60% of the variation in return for a mutual fund. There is also a good interview about Style Analysis with Dr. Sharpe here . FinancialEngines.com uses Style Analysis for its simulations, but there are no documented tests or validations that I have seen. It is not at all clear to me how FinancialEngines, using Style Analysis—a method designed for mutual funds—deals with a portfolio made up of individual stocks. Style analysis will not capture the portfolio impacts of specific asset classes such as REIT’s, energy utilities, or emerging markets unless these are specifically built into the style analysis model—and I have yet to see a demonstration of style analysis that included all of these classes.
Quantext’s Monte Carlo uses historical data on every stock or fund in a portfolio to derive statistical parameters that describe each asset. This is quite different from Style Analysis. Our simulation models calculate forward-looking risk and return measures for each portfolio component and account for both market risk and non-market risks and correlation effects explicitly. See a paper on this topic and demonstrations here. Aside from the issue of whether you use some form of style analysis of analyze individual assets, there is the additional challenge of estimating forward-looking parameters for either the indices or the individual assets.
The important thing is for Monte Carlo planning tools to be validated in a range of ways. If you peruse the wide array of papers on our site, you will see that we have performed a wide range of tests and validation studies. These tests give us confidence that our simulations are performing properly. In particular for Monte Carlo simulations, you can test the projected volatility in returns using options prices in the market and we have done so for a range of offerings. Samples of this type of validations are shown here .
We have written another paper (start of October 2006) that looks at potential problems with a Monte Carlo tool provided to millions of clients by one of the largest fund management / 401(k) provider firms. This firm’s model uses a method that is even simpler than style analysis. This model simply lumps holdings into a small number of generic classes (U.S. equity = S&P500, international equity = EAFE index, etc.). This article discusses how projections from a model like this will not be able to account for portfolio impacts of such broad classes as REITs, utilities, energy-focused funds, emerging markets funds, and technology funds. This paper is available here .