Open Interest Volume and Volatility Evidence From Taiwan Futures Markets Vol 34 Nbr 2 April
Post on: 26 Май, 2015 No Comment
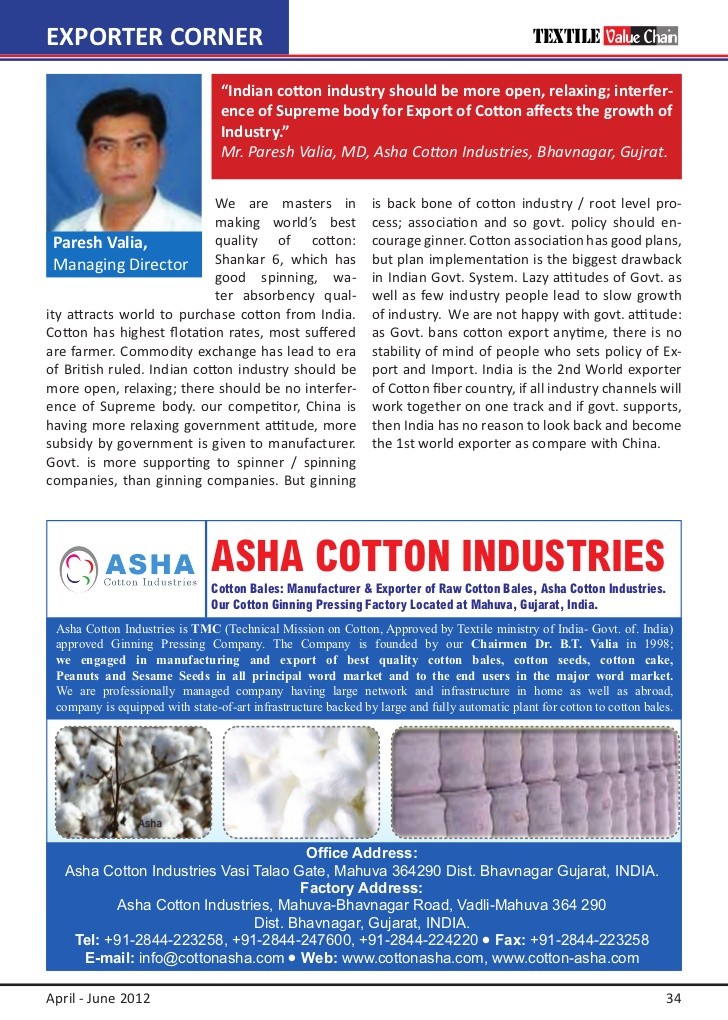
Open Interest, Volume, and Volatility: Evidence From Taiwan Futures Markets
FREE EXCERPT
1 Introduction
The literature has seen a chunk of studies dedicated to explore the relationship between the volatility of futures contracts and their trading volume and open interest. Several theories predict a positive relationship between return volatility and trading volume, and for futures markets open interest is considered to be another important variable. As noted by Bessembinder and Seguin (1993 hereafter BS), for example, a bi-directional causality relationship between volatility and trading volume (or open interest) would support the ‘mixture of distribution’ hypothesis (see Clark 1973). The ‘sequential arrival of information’ theory of Copeland (1976, 1977) would hold if volatility is dependent upon the lagged volume and/or lagged open interest. Also, Admati and Pfleiderer (1988)’s ‘traders with trade timing discretion tend to trade in heavy liquidity’ theory would apply if trading volume affects volatility.
Figlewski (1981) suggests that monthly trading volume and open interest of the GNMA futures help explain its monthly volatility. Karpoff (1987) argues that price changes and volume measures should exhibit positive contemporaneous correlation with each other and reviews eighteen studies that examine evidence with regard to this relationship. Tan and Gannon (2002) find that, apart from the return-volume relationship, the interrelationships between return, volatility and volume upon information arrival are generally consistent with what theory would anticipate.
The literature on modeling the relationship amongst these three variables has seen three main streams of development. First of all, BS suggest that open interest is a proxy for market depth (see Kyle 1985, for the definition of market depth). BS argue that open interest of futures markets apart from trading volume provides an additional measure of trading activity generated by large hedges (or commercial traders in the language of Chartrath et al. 2003). Partitioning the trading volume and open interest, respectively, into their expected and unexpected components, BS study eight physical and financial futures markets based on a model similar to GARCH-inmean. They find a strong positive relation between contemporaneous volume and volatility. To the contrary, they find volatility to be negatively related to both expected and unexpected open interest. Their results appear to suggest that an unexpected increase in open interest alleviates the effect of an unexpected increase in volume on volatility. BS ‘s method attracts some subsequent studies on futures volatility based on their model. Amongst others, Ragunathan and Peker (1997) provide evidence that positive volume shocks have a greater impact on volatility than negative shocks and reach a similar conclusion regarding open interest. They suggest that, therefore, both trading volume and market depth, as measured by open interest, do have an effect on volatility. Using BS’s method, Watanabe (2001) also presents evidence of a significant positive relationship between volatility and unexpected volume, as well as a significant negative relationship between volatility and expected open interest for Nikkei 225 stock index futures. However, they find that the relationship amongst price volatility, volume and open interest may vary depending on the market’s regulatory structure.
BS’s study also gives rise to research on the relationship between the futures market and the spot market. For instance, Chang et al. (2000) use BS’s method to examine the S&P 500 futures and find that the open interest of the S&P 500 futures increases as the volatility of its spot index increases. This finding tends to suggest that the increase in open interest of the S&P 500 futures induced by the increase in volatility of the spot index reflects higher hedging demand in index conditions of spot market uncertainty. This conclusion is echoed by Chen et al. (1995) who use an AR(2) model with the current implied volatility as one of the independent variables to study the same S&P 500 index spot and futures markets. Following BS, Chartrath et al. (2003) re-examine the effects of trading activity for the S&P 500 index futures on the volatility of S&P 500 spot index by classifying futures traders into four categories: commercial traders (large hedgers or institutional traders), non-commercial traders (larger speculators), small traders and spreaders. They find that unexpected change in lagged open interest of commercial traders is positively related to interday and intraday stock market volatility. However, such evidence is not replicated for the other three types of traders. Following, Motladiile and Smit (2003) also use Chen et al. (1995)’s method to examine three South African stock index futures contracts and find that positive shocks in open interest increases the volatility of the spot index.
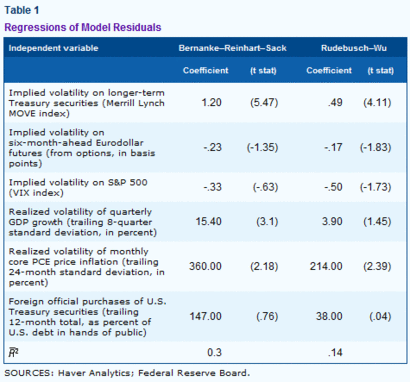
Another main stream of literature on volatility of futures markets concerns the use of the vector autoregressive (VAR) model. Fung and Patterson (1999) use the VAR model to examine the relationships amongst volatility, volume and open interest for five currency futures markets. They find mixed results: only trading volume is positively and significantly dependent upon volatility and open interest for all five currencies. Stated otherwise, volatility and open interest, respectively, Grangercauses trading volume. Similarly, Ferris et al. (2002) use the VAR model to study the interactions amongst pricing error, change in open interest, change in volume and change in implied volatility for the S&P 500 futures market. They document a bidirectional causal relation between the changes in open interest and trading volume. Nonetheless, the change in implied volatility is not related to either open interest or volume. Interestingly, moreover, the mis-pricing of the S&P 500 futures contract seems to affect all other variables above.
The third main type of models used to discuss the relations amongst volatility, volume and open interest involves the GARCH-type models. Girma and Moutgoué (2002, hereafter GM) add the current or the first lag of volume and/or open interest to the GARCH(1,1) model when studying four series of spread returns for the New York Mercantile Exchange (NYMEX) petroleum markets. They find that contemporaneous volume and open interest help explain the futures spreads volatility, when volume and open interest are added separately. This is also true for the lagged forms of volume and open interest. Their parameter estimates in the GARCH(1,1) model are all positive. However, when these two explanatory variables are entered simultaneously, the results are mixed. Only one spread’s volatility is significantly explained by the current volume and none of the four spreads has its volatility related to the current open interest. However, lagged volume is explanatory for two of the four spreads and lagged open interest for three of the four spreads.
Despite the number of studies on the relationship amongst volatility, trading volume, and open interest we in the literature discussed above, they are all conducted from an ex-post perspective. However, in-sample relationships amongst any variables, though significant, do not guarantee to happen again in the future. So one of this paper’s goals is to study this issue from an ex-ante perspective, i.e. by testing out-of-sample forecasting performance of the models examined. Given the importance of volatility in modern financial markets, we focus on volatility forecasting using other two variables, i.e. trading volume and open interest.
Turning to the literature on estimation and modeling of financial market volatility, which is also a key issue in econometrics and empirical finance, a plethora of efforts has been dedicated to this area. Amongst others, Chu and Freund (1996) compare the degree of option mispricing resulting from using volatility estimates from existing option prices’ implied standard deviations (ISD) and from GARCH and IGARCH models fit to the underlying assets’ returns and find that the ISD method is best. Similarly, Poon and Granger (2005) find that the ISD model tends.