Journal of Derivatives & Hedge Funds Risk analysis reporting and control of equity trading
Post on: 8 Апрель, 2015 No Comment
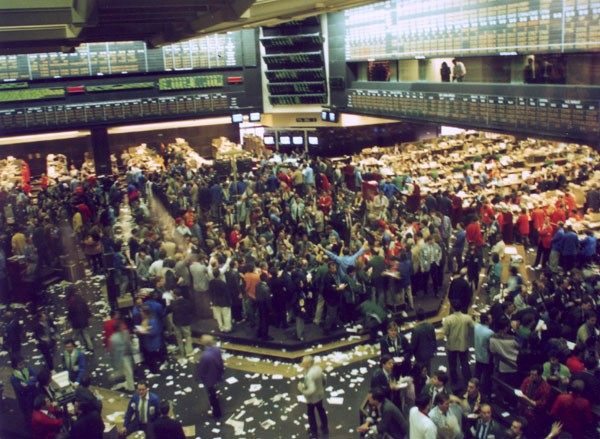
Journal of Derivatives & Hedge Funds (2007) 13, 3358. doi:10.1057/palgrave.jdhf.1850059
Risk analysis, reporting and control of equity trading exposure: Viable applications to the Mexican financial markets
Practical applications
Even though considerable literatures have investigated the statistical and economic significance of Value-at-Risk (VAR) models, this paper provides real-world techniques and optimum asset allocation strategies that are useful for trading/investment portfolios in emerging and illiquid stock markets. This is with the objective of setting up the basis of a methodology/procedure for the measurement, management and control of equity trading exposures in the day-to-day trading and/or asset management operations. The proactive risk management procedures that are discussed in this work will be of value to financial entities, regulators and policymakers operating within the context of emerging markets.
Mazin A M Al Janabi 1
Correspondence: Mazin A M Al Janabi, Department of Economics and Finance, College of Business and Economics, United Arab Emirates University, P.O. Box 17555, Al-Ain, United Arab Emirates. Tel: +(971) 3 7133384; Fax: +(971) 3 7624384; E-mail: m.aljanabi@uaeu.ac.ae
1 Mazin A. M. Al Janabi is Associate Professor of Finance and Banking and has several years of real-world experience in financial markets and banking sectors. He has worked for top international financial groups like ING-Barings (Dutch financial group) and BBVA-Bancomer (Spanish financial group), where he has held several senior positions, such as Head of Trading of Financial Derivative Products, Head of Trading Risk Management, Director of Asset and Liability Management and Director of Global Market Risk Management.
Received 8 December 2006; Revised 8 December 2006.
Abstract
This paper provides real-world techniques and optimum asset allocation strategies that can be applied to equity trading portfolios in emerging and illiquid financial markets. Key market risk management methods and procedures that financial entities, regulators and policymakers should consider in formulating their daily market risk management objectives are examined and are adapted to the specific needs of emerging countries. The aim of this paper is to fill a gap in the trading risk management literature and particularly from the perspective of emerging and illiquid markets, such as in the context of the Mexican financial markets. In this paper, we demonstrate a comprehensive and proactive approach for the measurement, management and control of equity trading risk exposure, which takes into account proper adjustments for the illiquidity of both long and short trading/investment positions under normal and severe market conditions and within a multi-security setting. Our approach is based on the renowned concept of Value-at-Risk (VAR) along with the innovation of a software tool utilising matrix-algebra and other optimisation techniques. To illustrate the proper use of VAR and stress-testing (scenario analysis) methods, real-world examples and practical reports of market risk management are calculated and presented for a selected portfolio from the Mexican Stock Market (BMV). To this end, several case studies were achieved with the objective of creating a realistic framework of trading risk measurement and control reports in addition to the inception of procedures for the calculation of the maximum authorised VAR limits.
Keywords:
emerging markets, financial engineering, financial institutions, financial risk management, Mexican stock market (BMV), stress testing, value at risk
INTRODUCTION AND OVERVIEW
Trading of financial instruments stocks (equities), bonds (fixed income instruments), derivative products and structured products, etc has been on uninterrupted expansion in emerging economies such as in the context of the Mexican financial markets. These markets are characterised in general as illiquid, volatile, politically unstable, lacking prudential regulations and historical financial databases. In spite of all these drawbacks, the potential of high expected rewards are tremendous and so the vast unforeseen risks. The management of inherited risks must, however, be dealt with by exercising both art and science techniques. Accordingly, the task and duties of risk managers, treasurers and CFOs in an emerging-market environment will, of course, be multiplied several-fold. The role of market risk management and its proper implementations are essential factors in the success of emerging markets’ financial trading activities.
Today, emerging markets of cash instruments and derivative products service a huge number of investors all of whom have different capabilities, needs and constraints, as well as different perspectives on the market and different attitudes toward risk. The variety of instruments (both cash and derivative markets) now available means that each of these investors should be able to implement a risk/return strategy that corresponds to their needs and expectations. At this time in several emerging economies, major commercial banks, investment banking firms and insurance companies have established new units of specialised traders and financial engineers to design and structure tailormade risk management products for corporate and retail customers. The continued success of these new emerging markets, as well as the satisfaction of each user, depends on a clear understanding of what these markets can offer of diverse product-lines and consequently the trade-off between the expected returns and their uncertainties (risks).
Trading consists of the proprietary positions in financial instruments which are held for resale (available for sale) and/or which are taken on by the financial entity with the intention of benefiting from actual and/or expected differences between purchase and sale prices or from other price variations (such as spread differentials). Market risk (or trading) is defined as the risk that the trading income will decrease due to an adverse price change in the traded financial instruments. To have a choice between a certain loss and a speculation with cash-markets or derivative instruments, one should set his organisation objectives and decisions utilising modern financial risk measurement tools to estimate worst-case scenarios. Thereafter, the level of the measured risk should be compared with the entity risk appetite. This is with the objective to ascertain if the risk falls within its risk limits and also to reveal if there are enough economic capital cushions to withstand unforeseen surprises. After all, what is most needed is a better understanding of the market risk management process. This can be accomplished by striking a number of institutional changes that will help reduce the uncertainties in the trading of securities. Naturally, this has to be accompanied with clearer legal environment, risk management and accounting standards, in addition to greater disclosures of trading transactions. Accordingly, this will make users, dealers and regulators better off and can improve their assessments of all kinds of risks they may encounter.
The lack of adequate market risk measurement, management and control tools are one of the contributing factors that have led to major financial losses among national/multinational corporations in emerging countries. The new Basle accord (so-called Basle II), for the establishment of adequate internal models of risk management, has motivated several emerging countries to be part of the agreement at different implementation levels. Several emerging markets, in the Asian and Latin American continents, would like to be Basel-compliant and hence are already in advanced steps to implement, by the end of the year 2007, modified versions of the Basle agreement with its suggested internal models.
A number of Latin American countries, such as Mexico, are also voluntarily joining the implementation of modified versions of the Basle II accord. In fact, the Mexican financial markets, in general, are in progressive stages vis—vis other emerging markets of implementing advanced risk management regulations and techniques. Moreover, in recent years local regulatory authorities have made certain progress in cultivating the culture of risk management among local financial entities and regulatory/supervisory institutions.
The Mexican financial industry is generally sound, and the country continues to develop its financial system to attract more foreign portfolio investors, and to expand the opening of its financial system to the exterior world. Consequently, several local financial institutions have already sold major parts of their equity to foreign banks’ subsidiaries and have started the process of modernising their internal risk management capabilities. Despite the latest progress in the Mexican financial markets to become a Basel-compliant country, recently it has been deemed necessary (by local regulatory authorities) to adapt proper internal risk models, rules and procedures that financial entities, regulators and policymakers should consider in setting-up their daily trading risk management objectives.
Set against this background and as a result of the previous discussion, equity risk management has become an important theme in emerging and illiquid markets, such as in the case of the Mexican financial markets. Accordingly, the goals of this work are to demonstrate the necessary analytical steps and internal risk management procedures that a market’s participant (dealer or market-maker) will need in his day-to-day positions’ taking.
In particular, the main aim of this paper is to add to the risk management literature and moreover to fill a gap in the equity trading risk management literature and especially from the perspectives of emerging and illiquid markets. This paper attempts to provide a practitioner viewpoint on how to lay down a proactive equity risk management methodology and procedures that can be applied to the day-to-day equity trading activities and specifically within the prospect of emerging economies. In this work, key equity market risk management methods, rules and procedures that financial entities, regulators and policymakers should consider in setting-up their daily equity trading risk management objectives are examined and adapted to the specific needs of emerging and illiquid markets, such as in the context of the Mexican Stock Market (BMV). Specifically, the study investigates the application of modern financial theory and financial risk management tools and techniques to the case of emerging markets’ trading portfolios that contain vast amount of illiquid equity cash securities. It also provides an insight on how to measure and report daily trading risk of both long and short trading positions, within its authorised risk limits constraints, in an innovative and proactive ways to senior management in financial entities. In order to illustrate the proper use of Value-at-Risk (VAR) and stress-testing methods, real-world examples and reports of equity trading risk management are presented for a selected stock portfolio and several indices of the Mexican Stock Market (BMV).
LITERATURE REVIEWS AND OBJECTIVE OF PRESENT WORK
Risk management has become a major endeavour by academics, practitioners, and regulators, and a cornerstone of recent interest is a class of models called VAR techniques. The concepts of VAR and other advanced risk management techniques are, in fact, not new and are based with some modifications on modern portfolio theory. The VAR method can alert you to the maximum loss that your portfolio (investment or trading portfolios) could experience at a specified probability so that you can evaluate such a loss’s potential on your business and to aid you in the decision of where and when to trim redundant risk(s). The most common VAR models estimate variancecovariance matrices of asset returns using historical time series, and assume that the distributions of asset returns are normal. Portfolio risk is a function of the risk of each asset and the correlation factors among the returns of all trading assets within the portfolio. The VAR is then calculated from the standard deviation of the portfolio, given the appropriate investment/liquidation horizon, and the specified confidence interval.
In the 1950s, Markowitz 1 described the theoretical framework for modern portfolio theory and the creation of efficient portfolios. The solution to the Markowitz theoretical models revolves around the portfolio weights, or the percentage of asset allocated to be invested in each instrument. Sharpe, 2 developed the single-index model, which relates returns on each security to the returns on a common index abroad market index of common stock returns such as S&P 500 is generally used for this purpose.
Despite many criticisms and limitations of the VAR method, it has proven to be a very useful measure of market risk, and is widely used in financial and nonfinancial markets. The RiskMetrics TM system, 3 developed and popularised by J.P. Morgan, has provided a tremendous impetus to the growth in the use of VAR concept and other modern risk management techniques and procedures. Since then the VAR concept is well known and scores of specific applications are adapted to credit risk management and mutual funds investments. The general recognition and use of large-scale VAR models has initiated a considerable literature including statistical descriptions of VAR and assessments of different modelling techniques and approaches.
For a comprehensive survey, and the different VAR analysis and techniques, one can refer to Jorion. 4 For the most part, VAR analyses in the public domain have been limited to comparing different modelling approaches and implementation procedures using illustrative portfolios (for instance, Hendricks, 5 Marshall and Siegel, 6 Pritsker 7 ). In their paper, Berkowitz and O’Brien 8 questioned how accurate VAR models are at commercial banks. Owing to the fact that trading accounts at large commercial banks have considerably grown and become increasingly diverse and complex, the authors presented statistics on the trading revenues from such activities and on the associated VAR forecasts internally estimated by banks.
Several other authors have attempted to tackle the issues of extreme events and fat tails phenomena in the distribution of returns. Nonetheless, although most of their approaches and techniques are good exercises for academic purposes, they do lack evidence of real-world applications with actual market portfolios. For instance, more rigorous mathematical treatment of VAR analysis with dynamic copula models and extreme value theory has received considerable treatment from Embrechts et al. 9
In their paper, Angelidis and Degiannakis, 10 enumerate the accuracy of parametric, nonparametric and semi-parametric methods in predicting the one-day-ahead VAR in three types of markets (namely, stock exchanges, commodities and foreign exchange rates) and for both long and short trading positions. According to Culp et al.. 11 VAR can be adapted for the use in asset management and for the estimation of market risk in the long-term horizon. In their study, they explore the application of VAR to asset management and with particular attention on the importance of VAR for multi-currency asset managers. In another relevant study, Dowd et al. 12 tackle the problem of the estimation of VAR for long-term horizon. In their paper, they offer a different, however, a rather straightforward approach that avoids the inherited problems associated with the square root of time rule, as well as those associated with attempting to extrapolate day-to-day volatility forecasts over long horizons.
In his research papers, Al Janabi 13. 14 has established a practical framework and other relevant parameters for the measurement, management and control of market (trading) risk. The effects of illiquid assets, that are dominant characteristics of emerging markets, were also incorporated in his models. The established models and general framework were mainly based on matrix-algebra techniques. This approach can facilitate the calculation of market risk for several trading assets and it can easily handle short selling of financial assets in day-to-day market risk measurements.
Set against this background, the objectives of this research paper are to provide practical and robust quantitative measurements and procedures of market risk (frequently it can be called trading risk or price risk) for emerging markets’ equity trading portfolios. This is with the objective of assisting these countries in the establishment of sound risk management practices within a prudential framework of policies and procedures. This study makes an important contribution to the trading risk management literatures by introducing a comprehensive and proactive approach for the measurement, management and control of equity trading risk exposure, which takes into account proper adjustments for the illiquidity of both long and short trading/investment positions (under normal and severe market conditions) and within a multi-security setting. To this end, the parameters required for the construction of appropriate and simplified VAR and stress-testing methods are reviewed from previous works and refined to the specific applications of these methods to emerging markets. Moreover, a simplified approach for the incorporation of illiquid assets, in daily trading risk management practices, is defined and is appropriately integrated into the VAR and stress-testing models.
The theoretical mathematical/analytical models that are adopted herein are based on matrix-algebra approach. The latter approach can in fact simplify the programming process in EXCEL TM worksheets and can also permit easy incorporation of short selling of assets in the equity trading process. The latter effect on trading positions can, in fact, aid in the setting-up of optimum structure of VAR limits. In this work, a genuine model for the measurement of the illiquidity of both short and long trading position is incorporated. In contrast to other commonly used liquidity models, the liquidity model that is applied in this work is more appropriate for real-world trading practices since it considers selling small fractions of the long/short trading securities on a daily basis. Although the risk measurement method that is adopted in this work is based mainly on the variancecovariance approach (that assume normal distribution of returns), for emerging and illiquid markets, it is possible to correct for the assumption of normality by including stress testing (under severe market conditions) along with the aggregation of a realistic risk factor that takes into account illiquid trading securities.
Market risk management models, which are implemented in this work, were applied to a selected number of stocks, sector indices and main market indicators of the Mexican Stock Market (BMV). Databases of daily stocks’ prices movements and market indices (such as the main market’s indictor, IPC index) were all gathered, filtered and processed in such a manner to create meaningful quantitative analysis and conclusion of market risk measurement. Several case studies were carried out with the objectives of calculating VAR numbers under various possible scenarios in addition to the inception of a practical framework for the establishment of VAR limits setting. The different scenarios were performed, first with distinct asset allocation percentages, secondly by studying the effects of liquidity of trading assets (unwinding period of assets), and finally by taking into account the possibilities of short selling in daily trading operations. Furthermore, several tests of abnormal (asymmetric) distributions of returns were performed. To this end, various tests of skewness and kurtosis were implemented on the sample stocks and the various markets’ indices. This was followed by a study of daily and annual volatilities along with the calculations of betas of the sample stocks against the main market’s indicator, namely the IPC index.
The remainder of the paper is organised as follows. The following section lays down all the mathematical/quantitative infrastructures of VAR, and its limitations, and a model that incorporates the effects of illiquid assets in daily market risk management. The results of the empirical tests are drawn in the final section along with conclusions and recommendations. Full set of all market risk management reports are included within Appendix A .
IMPLEMENTATION OF VAR WITH THE VARIANCE/COVARIANCE (PARAMETRIC) APPROACH
VAR is a method of assessing market risk that uses standard statistical techniques routinely used in other technical fields. Formally, VAR measures the worst expected loss over a given time interval under normal market conditions at a given confidence level. The standard deviation of the daily rate of return is used as an estimation of the potential loss or gain the firm may incur. Assuming that the return of a financial product follows a normal distribution, linear pay-off profile and a direct relationship between the underlying product and the income, the VAR is to measure the standard deviation of the income for a certain confidence level. In reality, the VAR is a forecast of the standard deviation. Although the method relies on several assumptions and has been heavily criticised and is often discouraged, it has gained wide acceptance for the quantification of financial risks within financial trading units. As a result of the generalisation of this method, capital allocations for trading activities tend to be calculated and adjusted with the VAR method.
A financial entity might say that the ‘daily ‘ VAR of it trading portfolio is $1 million at the 99 per cent confidence level. This means, there is only 1 chance in a 100 (or one day in every 100 trading days), under normal market conditions, for a loss greater than $1 million to occur. This single number summarises the bank’s exposure to market risk as well as the probability of an adverse move. Equally important, it measures risk using the same units as the bank’s bottom line dollars. Shareholders and managers can then decide whether they feel comfortable with this level of risk. If the answer is no, the process that led to the computation of VAR can be used to decide where to trim the risk. While this method is powerful for day-to-day risk management, it is no substitute for the wider risk management process of analysing crash scenarios and keeping control on operational and legal risks.
In order for this method to perform properly, accurate trading positions should be gathered and historical database of these positions should be built accordingly. Once the position data are centralised, the overall risk has to be calculated by aggregating the risks from individual contracts across the whole portfolio. This is done by working out the effect of moves in individual ‘risk factors ‘ (eg equities, money market instruments, foreign exchange rates) across the portfolio, which may involve large currencies and, with each currency, different asset classes. VAR is worked out from the relationships between the individual risk factors and the effect on the portfolio of moves in each risk factor.
So far, there is no industry consensus on the best method for calculating VAR. As with any statistical model, VAR depends on certain assumptions. The choice of which method of calculation is used is normally dictated by the user’s aversion to unrealistic or over-simplistic assumptions. There are three popular methods: the ‘variance/covariance ‘ method (also known as the ‘correlation ‘ or ‘parametric ‘ method), the ‘historical simulation ‘ method and the ‘Monte-Carlo simulation ‘ method. Each of these methods has its own set of assumptions and each is a simplification of reality.
The variance/covariance method is the simplest one in terms of application to financial practices and computer time consumption. This method assumes that the returns on risk factors are ‘normally distributed ‘ and the correlations between risk factors are constant. For risk management purposes, and although it is often warned against, using the normal distribution assumption is generally considered to be acceptable. Deviation from normality usually does not significantly alter the results of the VAR calculations under the assumption of normal market conditions. Within this method, a bell-shaped curve (Gaussian distribution) is essentially assumed and it also assumes that extreme price swings, such as market crashes, occur too rarely to contribute to an accurate picture of the likelihood of future events.
To calculate VAR using the variance/covariance method, the volatility of each risk factor is extracted from a pre-defined historical observation period. The potential effect of each component of the portfolio on the overall portfolio value is then worked out. These effects are then aggregated across the whole portfolio using the correlations between the risk factors (which are, again, extracted from the historical observation period) to give the overall VAR value of the portfolio with a given confidence level.
Many financial institutions have chosen a confidence interval of 95 per cent (or 97.5 per cent if we only look at the loss side (one-tailed)) to calculate VAR. This means that once every 40 trading days, a loss larger than indicated is expected to occur. Some banks use a 99 per cent (one-tailed) confidence interval, which would theoretically lead to larger loss once every 100 trading days. Owing to fat tails of the probability distribution, such a loss will occur more often. Some financial institutions feel that the usage of a 99 per cent confidence interval would place too much trust on the statistical model and, hence, some confidence level should be assigned to the ‘artside ‘ of the risk measurement process.
A simplified calculation process of the estimation of VAR risk factors (using variance/covariance method) for a single and multiple assets’ positions is illustrated 13. 14 as follow:
From elementary statistics, it is well known that for a normal distribution, 68 per cent of the observations will lie within 1 (standard deviation) from the expected value, 95 per cent within 2 and 99 per cent within 3 from the expected value, thus the VAR of a single asset in dollar terms is:
where is the confidence level (or in other words, the standard normal variant at confidence level ) and i is the standard deviation (volatility) of the security that constitutes the single position. The value of the position, is the amount of investment in dollars, of instrument i.
For multiple assets or portfolio of assets, VAR is a function of each individual security’s risk and the correlation factor between the returns on the individual securities as follows:
This formula is a general one for the calculation of VAR for any portfolio regardless of the number of securities. It should be noted that this formula is presented in terms of matrix-algebra a useful form to avoid mathematical complexity, as more and more securities are added. This approach can, in fact, simplify the programming process in EXCEL worksheets and can also permit easy incorporation of short selling in the market risk management process.
This means, in order to calculate the VAR (of a portfolio of any number of securities), one needs first to create a matrix of the individual VAR positions, a transpose matrix (indicated above by the letter ‘T’ on the top of the matrix) of the individual VAR positions and finally a matrix of all correlation factors ( ). Once one multiplies the three matrices and then takes the square root of the result, he ends up with the VAR P of any portfolio with any n -number of securities. This simple number summarises the portfolio’s exposure to market risk. Investors and senior mangers can then decide whether they feel comfortable with this level of risk. If the answer is no, then the process that led to the estimation of VAR can be used to decide where to reduce redundant risk. For instance, the riskiest securities can be sold, or one can use derivative securities such as futures and options to hedge the undesirable risk.
Illiquid securities such as equities are very common in emerging markets. Customarily, these securities are traded infrequently (at very low volume). Their quoted prices should not be regarded as a representative of the traders’ consensus vis—vis their real value but rather as the transaction price that arrived at by two counterparties under special market conditions. This, of course, represents a real dilemma to anybody who seeks to measure the market risk of these securities with a methodology which is based on volatilities and correlation matrices. The main problem arises when the historical price series are not available for some securities or, when they are available, they are not fully reliable due to the lack of liquidity.
The choice of the ‘time -horizon ‘ or number of days to liquidate (unwind) a position is very important factor and has big impact on VAR numbers, and it depends upon the objectives of the portfolio and the liquidity of its positions. For a bank’s trading portfolio invested in highly liquid currencies, a one-day horizon may be acceptable. For an investment manager with a monthly re-balancing and reporting focus, a 30-day period may be more appropriate. Ideally, the holding period should correspond to the longest period for orderly portfolio liquidation.
In fact, if one assumes normal distribution, then he can convert the VAR horizon parameter from daily to any t-day horizon. The variance of a t-day return should be t times the variance of a 1-day return or 2 =f (t). Thus, in terms of standard deviation (or volatility), =f (t 1/2 ) and the daily VAR number can be adjusted for any horizon as:
The above square root of time rule was proposed and used by J.P. Morgan in their earlier RiskMetrics TM methodology. 3 Unfortunately, the latter approach does not consider real-life-trading situations, where traders can liquidate (or re-balance) small portions of their trading portfolios on a daily basis. Moreover, this could generate unreliable risk assessments and can lead to considerable overestimates of VAR figures, especially for the purposes of economic capital allocation between trading and/or investment units.
In order to perform the calculation of VAR under more realistic illiquid market conditions, one can define the following: 13. 14
where t is the number of liquidation days (t-day to liquidate the entire asset fully), VAR is VAR under liquid market conditions and VAR adj is VAR under illiquid market conditions.
A linear liquidation procedure of the asset is assumed in the above formula, that is selling equal parts of each asset every day till the last trading day (t ), where the entire asset is sold. The above model is more appropriate for daily trading circumstances where traders can unwind part of their positions on a daily basis. Moreover, the above approach can also be used to calculate the VAR for any time horizon.
As described earlier, VAR method is only one approach of measuring market risk and is mainly concerned with maximum expected losses under normal market conditions and within a specified level of confidence. For prudent risk management and as an extra management tool, firms should augment VAR analysis with stress testing and scenario procedures. The VAR methodology gives a probabilistic measure of loss that is exceeded, say 2.5 per cent of the time. From a risk management perspective, however, it is desirable to have an estimate for what potential losses could be under severely adverse conditions where statistical tools do not apply.
Stress testing estimates the impact of unusual and severe events on the entity’s value and should be reported to senior management on a daily basis as part of the risk reporting process. For emerging-market countries with extreme volatility, the usage of stress testing should be highly emphasised and full description of the process should be included in any policy and procedure manual. Stress testing usually takes the form of subjectively specifying scenarios of interest to assess changes in the value of the portfolio and it can involve examining the effect of past large market moves on today’s portfolio.
In this paper, risk management procedure was developed to assess potential exposure due to an event risk (severe crisis) that is associated with large movements of the Mexican Stock Market during periods of financial turmoil. The task here is to measure the potential risk exposure that is associated with a pre-defined leap under several correlation factors assumptions.
MARKET RISK MANAGEMENT THE MEXICAN STOCK MARKET (BMV) CASE
Market (trading) risk management is a unit within global risk management that is responsible for monitoring all risks related to proprietary trading (or position taking) of the financial institution. Market risk deals with risk within short-term time horizon positioning, where all trading positions are marked-to-market and risk is re-evaluated on a daily basis and performance is measured via daily profit and loss and impacts immediately the financial institution’s income statement. This is so only for financial trading units that operates on short horizons.
In measuring the market risk of a trading position, the first step is to identify the market risk factors that affect its mark-to-market value. For certain trading positions, the identification of the market risk factors is quite straightforward. For instance, for a trading position in cash equities, the prices of the individual stocks determine the value of the position and, therefore, the equity prices could be taken as the market risk factors. There is, however, a problem with this approach: for a large and diversified trading book the number of risk factors becomes very large and the risk measurement and aggregation becomes unmanageable. Fortunately, financial theory and related empirical research provide ways of simplifying the number of market risk factors for equity positions.
In the study reported herein, databases of some of the most liquid stocks (11 stocks in total) and market/sectors indices that are encountered in the Mexican Stock Market were gathered, filtered and adequately adapted for the creation of relevant inputs for the calculation of all risk factors. These 11 stocks are the main components of the major local stock market index (IPC index). A brief description of the 11 stocks’ industrial sectors and the market/sectors indices (all are capitalisation weighted indices) are included in parentheses as follows:
- Grupo Televisa (Television and Media)
- Tv Azteca (Television and Media)
- Vitro (Glass and other Industries)
- Telfonos de Mxico (Telecommunication)
- Empresas Ica (Construction)
- Grupo Modelo (Beverages)
- Fomento Econmico Mexicano (Beverages)
- Grupo Continental (Soft Drinks)
- Cemex (Cement)
- Alfa (Steel and Conglomerate)
- Walmart de Mxico (Retailer Chain)
- Construccin Index (Cement, Construction and Housing Companies and contains 10 Stocks) Comunicaciones y Transportes Index (Telecommunication and Transportation Companies and consists of 13 Stocks)
- Servicios Index (Financial Institutions and other services and incorporates 11 Stocks)
- Extractiva Index (Mining Companies and includes 2 Stocks)
- Comercio Index (Retailer Chains and comprises of 17 Stocks)
- Transformacin Index (Chemical, Manufacturing, Beverages, Textiles and Metals Companies and consists of 35 Stocks)
- Varios Index (Holding and other companies and includes 15 Stocks)
- INMEX Index (Market Indicator, consists of 20 Stocks and is also used as an underlying asset for the underwriting of derivatives products)
- IMC30 Index (Index of Medium Size Companies and contains 30 stocks)
- IPC Index (Main Market Indicator and involves 35 Stocks).
Historical databases (for more than three years) of daily prices were assembled for the purpose of carrying out this research and further for the construction of market risk management parameters and risk limits. In the process of analysing the data, first, the daily stock’s returns of the 11 sample stocks as well as the daily returns of the market/sectors indices have been calculated. These daily returns are, in fact, essential ingredients for the calculation of standard deviations, correlation matrices, stocks’ betas, skewness and kurtosis of all the sample stocks/indices and their relationship vis—vis the IPC index.
A software package was contrived for the purpose of creating a trading portfolio of these stocks and consequently to carry out all VAR and scenario-analysis calculations and results. The approach that was used in building-up the software package was based on matrix-algebra and multiplication of matrices. In fact, EXCEL package has many built-in functions that can permit the performance of multiplication of matrices and other useful mathematical/statistical calculations.
The analysis of data and discussions of most of the relevant findings and results of this research will be organised and explained as follow.
Analysis of volatility, beta, skewness and kurtosis
In this section, the analysis of the particular risk of each stock/index (daily and annual volatility), the stocks/indices relationships with respect to the IPC index and finally a test of normality (symmetry) are performed on the sample stocks and the market/sectors indices.
Table A1 illustrates the daily volatility of each of the sample stocks under normal market and severe (crisis) market conditions. Crisis market volatilities were calculated by multiplying the normal volatilities by a factor of five. From the table, one can observe that the stock with the highest volatility is Empresas Ica, whereas Telfonos de Mxico stock has demonstrated the lowest volatility. Annualised volatilities are depicted in Table A2. and this was performed by adjusting (multiplication) the daily volatilities with the square root of 260 assuming that there are 260 trading days in the calendar year.
An interesting outcome of the study of betas (systematic risk) is the manner in which the results were varied across the sample stocks as indicated in Table A1. Grupo Televisa stock appears to have the highest beta (1.34) vis—vis the IPC index (ie the highest systematic risk) and Vitro stock seems to have the lowest beta (0.42). Moreover, Walmart de Mxico stock (with a beta of 1.06) is the best candidate of the entire sample stocks that appears to move very closely with respect to the IPC market index (with a beta of 1.0). In addition, as one might expect, the INMEX and Comunicaciones y Transportes Indices have very close relationship with the IPC index with betas of 1.06 and 1.11, respectively.
In another study, the measurements of skewness and kurtosis were achieved on the sample stocks and market/sectors indices. The results are also depicted in Table A1. It is seen that in general all stocks have shown asymmetric behaviour (both positive and negative values). Moreover, kurtosis studies have shown similar patterns of abnormality (ie peaked/flat distributions). At the upper extreme, Empresas Ica stock has shown the greatest positive skewness (0.69) which is combined with a very high kurtosis peakedness of (10.19). Some stocks, such as in the case of Alfa stock, has shown a close relationship to normality (skewness of 0.19 and kurtosis of 3.45). Likewise, the IPC index has also indicated some signs of normality with -0.18 and 2.59 of skewness and kurtosis, respectively.
The interesting outcome of this study suggests the necessity of combining VAR calculations which assumes normal distributions of returns with other methods such as stress testing and scenario analysis to get a detailed picture of other remaining risks that cannot be captured with the simple assumption of normality.
Matrices of correlations
Three matrices of correlations were created in this study, namely correlation=1, 0 and exact (or empirical) correlations. The objectives here were to establish the necessary quantitative infrastructures for advanced risk management analysis that will follow shortly. The assembled correlation matrix is depicted in Table A3 for the exact correlation case of all stocks and indices. The latter correlation matrix was an essential element along with volatilities matrices for the creation of VAR and stress-testing calculations for equity market risk management processes and procedures. The results of Table A3 confirm several well-known facts in the Mexican Stock Market such as the high correlation factor (72 per cent) of Telfonos de Mxico stock with the IPC index. Likewise, the INMEX and Comunicaciones y Transportes Indices have shown strong relationships with the IPC index. This is evidenced with the high correlation factors of 99 per cent and 90 per cent, respectively.
In this section, several case studies were carried out to emphasise the importance of market risk management reports for daily risk-taking practices. In the calculations reported herein, the effects of different portfolio combinations, various liquidation periods (unwinding horizons of stocks holdings) and short selling of stocks, were all investigated.
Table A4 illustrate a practical sample report for the coverage of equity market risk management activities of a hypothetical equity portfolio consisting of some of the most active stocks in the Mexican Stock Market. In this first case study, total portfolio value was P$110 millions with an equal asset allocation of 9.1 per cent and a liquidity horizon of one trading day that is the trading unit requires one day to unwind all trading positions. This table illustrates the effects of stress testing (VAR under severe market conditions) and different correlation factors on daily VAR calculations. The VAR report depicts also the overnight (daily) volatilities that were calculated as the volatility of the percentage price changes (daily returns) of these stocks and their respective betas.
The VAR results were calculated under normal and severe market conditions by taking into account different correlation factors (+1, 0, and exact correlations between the various risk factors). Under correlation +1, one is assuming 100 per cent positive relationships between all risk factors (risk positions) all the times, whereas for the 0-correlation case, there is no relationships between all positions at all the times. The last correlation case takes into account the empirical (exact) correlation between all positions and was calculated via variance/covariance matrix.
As one might expect, the case with correlation +1 gives the highest VAR numbers (P$4,959,166 and P$24,795,831), owing to the fact that under these circumstances the total VAR of the portfolio is the weighted average of the individual VAR of each trading position. It is essential to include various correlation factors in any stress-testing exercise, based on the fact that current trends in correlations may break down with severe market movements, caused by unexpected financial or political crises. The degree of diversification of this hypothetical trading portfolio can also be displayed as the difference in the value of the two greatest VARs, that is the VAR of correlation = +1 case versus the VAR of the exact correlation case (P$1,996,659 or 67.40 per cent for the normal market condition case). The beta of this portfolio is also indicated in this report as 0.915.
Since the variations in daily VAR are mainly related to the ways in which the assets are allocated in addition to the liquidation period of assets and the effects of short selling, it is instructive to examine the way in which the VAR figures are influenced by changes in such parameters. Table A5 illustrates the changes in VAR numbers when the liquidation period was increased to ten trading days for all stocks within the portfolio. In Table A6. it was assumed that the first six assets should be liquidated in five trading days, whereas the remaining five assets can be liquidated in ten trading days, respectively.