Hedge Fund Portfolio Risk
Post on: 21 Июнь, 2015 No Comment
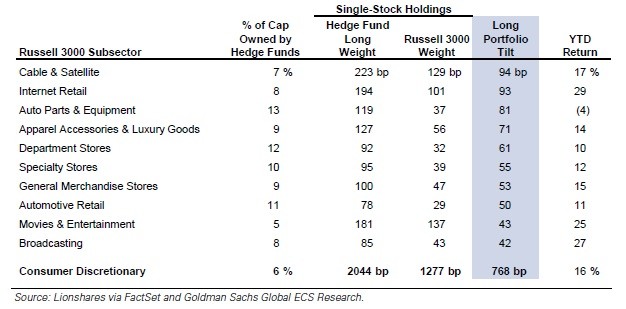
Hedge Fund Portfolio Risk
Funds of Hedge Funds have long been challenged by the lack of transparency offered by the managers in whom they invest. Many different approaches are used in practice when dealing with transparency, ranging from full daily transparency to only monthly return information. In this paper, we discuss some of the more popular techniques that Funds-of-Hedge-Funds (FoHFs) use to address transparency, and we present an advanced methodology that allows the FoHF manager to better understand what factors drive their portfolio’s and their managers’ risk and returns. Note that in this white paper, we use the term Fund of Hedge Fund because of its popularity, but the same analysis holds for any multi-manager platform. Asset allocators, pension funds, endowments and family offices investing in hedge funds all face the same issues, and this paper addresses their needs as well.
Before we begin the analysis of FoHF transparency and risk issues, there is an important point to make about the use and role of quantitative risk management in a multi-hedge fund environment. The tools and techniques that risk management brings to bear on the investment management process are at their best when they are used in conjunction with the professional judgment of the portfolio manager/risk manager. No model or technique, regardless of its sophistication, should be used as a replacement for good judgment. The proper role of risk management for FoHFs is to help the manager understand the portfolio’s sensitivities and the drivers of risk, ultimately leading to a better understanding of the portfolio dynamics and to asking better questions of the underlying managers.
While some hedge fund managers are willing to share their holdings on a daily basis, many do not provide such detail and limit their transparency in some way: perhaps they send a delayed snapshot of the positions or allocations into some set exposure buckets. Some provide top 10” longs/shorts or some other partial view into their investments. Others provide no details and offer to inform their investors about their performance, typically on a monthly basis. An Individual FoHF may have managers in each category, leading to a variety of levels of transparency, ranging from full daily positions to only monthly returns. Collecting and analyzing such disparate pieces of information can be quite a challenge.
We collaborated with Fund of Hedge Funds using real data on real Hedge Fund returns.
While some in the hedge fund and FoHF community subscribe to the notion that meaningful quantitative risk management requires access to full daily position level transparency, that option may not always be available. The trend emerging in the industry is the use of managed returns as a basis for performing risk analysis. This paper presents a significant step forward and shows the clear merits of that process.Dealing with transparency is among the first topics a FoHF manager needs to address. Below, we discuss a variety of methods that deal with some level of transparency before addressing the main topic of the whitepaper – a new returns-based technique to identify meaningful drivers of hedge fund risk. The levels we consider below fall into four categories:
- Full daily position transparency
- Limited position transparency (e.g. monthly positions, “top 10” positions, lagged positions)
- Exposure transparency (e.g. allocations to exposure buckets, risk factor allocations)
- No holding transparency (returns only)
Position level transparency clearly provides the best means of monitoring the activities of the underlying hedge fund managers. It allows the FoHF manager to use those positions in a risk management platform for the most up- to-date and complete modeling of the portfolio’s risks including scenarios, what-if analyses and stress tests. It is very rare for a FoHF to have 100% compliance for transparency from every manager unless that is an unconditional requirement of the investment. While there are FoHFs that invest only in managers that provide complete transparency that is far from the norm. The challenge then becomes how to measure the risk of a portfolio with mixed levels of transparency.
Partial position transparency, such as top 10” long positions and “top 10” short positions can result in misleading analyses if those top 10 do not comprise a meaningful portion of the portfolio’s total exposure. If the “top 10” positions account or only 50% of the portfolio, risk analysis based solely on the “top 10” is far from adequate, but certainly better than no risk management at all. In practice, FoHF managers typically assign proxy positions to account for the remaining exposure not included in the “top 10.”
When a manager provides exposure buckets, the firm typically sends the output from some risk management system that assigns allocations to different risk types. The challenge here is that each manager will typically send completely different buckets. Even if two managers send the same general buckets, such as a geographical breakdown, those buckets rarely overlap well and do not lend themselves to aggregation. For example, one manager may send geographical categories of US, Europe and Asia, while another sends Developed and Emerging. In these situations, a large degree of manual data massaging is necessary in order to make use of the information. Even then, when one manager sends vega risk and another sends credit exposure, there is only so much one can do to aggregate and analyze the overall portfolio.
Returns based analyses come in a variety of forms, and one common approach is to use factor models. The goal is to reproduce the manager’s or FoHFs historical returns using a series of mathematical functions of return drivers. In general, the returns of the fund can be modeled as:
where the portfolio’s returns can be broken in an alpha term, a series of betas to driving factors and a residual term. The factors can be individual time series returns (for example, of an index or factor) or a function of a time series, allowing for quite complex structures.
This type of analysis is used in many fields – from econometrics to engineering – and has been a staple of the long-only investment management field for quite some time. The long-only industry typically uses only one (linear) factor in its analyses and tends to speak of the fund’s beta (as if there were only one possible factor). In the alternatives industry, where absolute returns are sought rather than returns relative to one index, multiple factors are employed. Since hedge fund returns are known to have non-linear relationships to major market indices and factors, refinements of this model are needed to make it applicable to FoHFs.
However, the general concept of decomposing a FoHF’s returns into driving factors takes the form of equation (1).
Several academic studies have been conducted, and several commercial firms have worked on the theoretical issue of hedge fund return analysis, normally accessing large databases of manager returns in order to study which techniques and effects are important to FoHFs. We find these studies quite useful on theoretical grounds, but materially lacking in practicality.
For illustrative purposes, consider a FoHF manager invested in six different commodity funds. Most of the underlying managers trade futures on natural gas, crude and metals. There is some minimal equity exposure. We use an appropriate collection of commodity specific indices as the initial set of candidate risk factors. Since there is a strong crude and natural gas component, we also use rolling generic futures contracts of varying maturities. In total, we consider 50 different return histories.
For this reason, our approach has been to study the academic and commercial literature and to develop a practical and useful tool by working with real FoHF managers. Our techniques are not only theoretically sound, but they also pass the test of usefulness among practitioners.
The goal of our sensitivity analysis is to reproduce a FoHF’s historical (real) returns using the best basket of driving factors, comprising both quantitative and qualitative analyses. We then work closely with the FoHF manager to identify historical periods of interest and ranges of stresses on those drivers that are both realistic and potentially damaging. Note that the goal is not to predict how a FoHF will behave in future markets, but rather to give the FoHF portfolio manager and risk manager a good sense of the sensitivity of the portfolio to different factors. The return replication is not meant for predicting future returns, but as a means to identify the main drivers of risk and return.
Suppose that a particular FoHF is modeled with a quadratic relationship to some driving factor – for example, the US equity market. In this case, a 1% drop in US markets results in only a 1% fall in the FoHF, but a 3% drop in US equities translates into a 10% decline in the FoHF. Once such a fat tail is revealed, the prudent risk manager will identify which of the underlying hedge funds, or which combination of hedge funds, has the largest sensitivities to large movements in the equity markets and then works to limit the overall FoHF’s sensitivity to such extreme movements.
Our methodology is quite simple in summary, but rigorous and complex in detail. There are three main steps in our process:
- Determine the list of potential factors.
- Build a list of driving factors – perform multi-pass regression between the fund’s returns and individual factors, consider linear and non-linear terms, consider lagged returns, use Principal Component analysis, ensure independence of residual terms, and evaluate goodness of fit using proprietary scoring mechanism.
- Cull the list with qualitative input: examine the appropriateness of each factor against stated objectives and the guidelines of each manager.
We start with a list of potential risk drivers that is determined by considering the asset classes and strategies of the underlying managers. The list can include market indices, exchange rates, interest rates, individual securities, and so on. It can also include proprietary risk factors that have been transformed into a time series. Each individual FoHF can choose to include or exclude any particular factors. Building the basket of driving factors is an iterative process that considers inclusion of different combinations of factors while it keeps track of the best combination.
In order to have reasonable statistical significance, most FoHF managers use a minimum of 24 data points (two years’ of monthly returns), but 36 data points are generally preferred (three years’ worth). It is practically unheard of for a FoHF to have not turned over a single manager in a two- or three-year time span – meaning that practically every FoHF has to rely on the manager’s returns prior to their investment as part of their return history.
This is typically achieved by using an historical return series from the manager prior to the investment period. If the older returns are from the same fund that the FoHF is invested in, then the analysis is on solid ground. If, however, the manager does not have an actual track record and has to rely on pro-forma data, then significant care needs to be taken in examining that data before including it in the analysis.
Our multi-pass approach starts by examining all possible factors for inclusion in the basket. It then iterates through each of the possible factors, performing regression analysis to maximize the resultant score using IA’s proprietary scoring mechanism. The scoring mechanism is shared with our clients and sometimes modified, in consultation with them, to take into account the nuances of their portfolio. For each iteration, only the highest scoring factor is added to the good basket. We iterate the possible factors several times until the inclusion of any additional factors results in a lower overall score, indicating that we have already found the best fit.
Non-linear behavior is a hallmark of hedge funds. Sometimes a fund will exhibit linear behavior for small movements but non-linear behavior for large movements. Similarly, it may exhibit a higher sensitivity to the positive returns of some factors than to that factor’s negative returns. IA examines these sorts of relationships in the process of building he basket using both quantitative analyses and visualization tools that allow for quick identification and confirmation of the results.
Once the “best” basket of drivers is determined from this quantitative analysis, a crucial next step is to apply qualitative analysis to the results. Even though a particular basket may have the best overall score and reproduce the fund’s (or the managers’) returns quite well, the basket itself may not sit well with the FoHF manager. It may be time to cull the list and examine alternative factors. Removing one or more factors from consideration (and re- running the iterative analysis) may result in a lower score, but the new basket may make more sense as a set of driving factors. In other words, qualitative results that do not pass the “sniff test” should be scrutinized and removed. Spurious results do sometimes crop up, and it is important to use good judgment in accepting any qualitative analysis.
Again, the goal of the analysis is to provide a meaningful assessment of the FoHF’s sensitivity to driving factors, and no one should be tempted to rely on a quantitative method just because the numbers turn out a particular way. However, it is also important to note that sometimes a manager or fund shows significant sensitivity to a surprising factor or set of factors. In these cases, it is best to examine the portfolio, usually in consultation with the managers, and determine whether such sensitivity is real or coincidental. After the manager’s risk drivers have been identified, the analysis should be updated each month as new returns are generated. It is important for the FoHF manager to be aware of changes in a manger’s set of risk drivers. While this may or may not be an indication of style drift, being aware of a material change in the driving factors can lead to better questions in the course of regular conversations with the hedge fund manager.
Once the best set of drivers is established, the next step is to examine the history of the factors to identify periods and ranges of typical and atypical returns and volatilities for the indices. This step is important because it assesses the range of movements that have happened over the past several years and provides guidance on which possible combinations of market movements can lead to serious consequences for the fund. This information is then used in deciding not only which historical periods to replay but also which markets to stress when constructing nightmare scenarios. The basket of risk drivers is also used for the non- transparent managers in lieu of actual positions for all the expected standard risk analytics — Value at Risk, expected shortfall, correlations, volatility analysis, etc.
In this paper, we have shown how a FoHF can perform meaningful risk analysis even if a significant portion of the book is invested in non-transparent managers.
The key is to analyze the different parts of the portfolio on equal footing: synthesizing transparency for those managers who do not offer it themselves. Part of this process is incorporating the quantitative and qualitative analyses of the individual hedge funds. By combining the analysis offered by a methodology such as ours with the professional judgment and experience of its managers, a FoHFs can have state of the art risk management practices. In conclusion, by using the synthesized transparency for the non-transparent managers, the FoHF can take advantage of all the risk management tools normally reserved for those only with position level transparency.