Cluster Analysis as a Funds of Hedge Funds Portfolio Tool The Finance Professionals Post
Post on: 8 Июль, 2015 No Comment
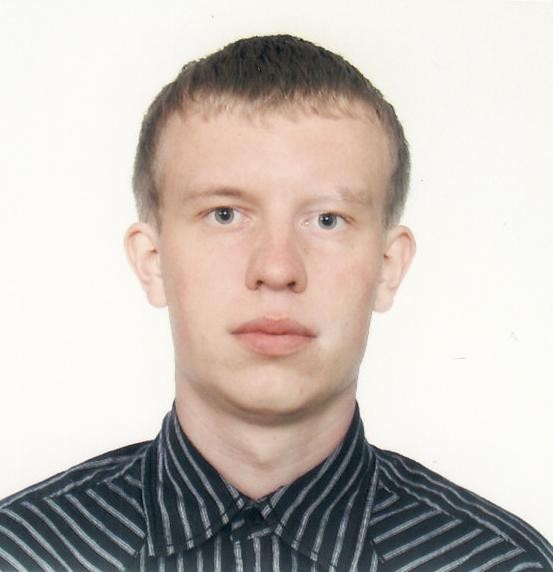
The Finance Professionals’ Post educates readers in the finance and banking sectors on the forces that shape their business. The FPP is a publication of the New York Society of Security Analysts (NYSSA).
04/20/2010
Cluster Analysis as a Funds of Hedge Funds Portfolio Tool
Constructing a diversified portfolio of managers in a fund of funds requires a method for determining how the different exposures complement each other. We show how cluster analysis can be an important tool in this process, especially during a period of market turmoil. Cluster analysis complements the qualitative assessment of a manager’s style and can be used to develop a view of changing markets and manager responses to these changes.
Figure 1: Cluster Analysis Results
Using cluster analysis to group investments has been an overlooked tool among money managers, long accustomed to applications of stochastic modeling. As shown in many nonfinance studies (see for instance Hartigan 1975), cluster analysis has the power to reveal patterns that researchers, analysts, and decision makers might not easily uncover in the data—patterns that provide insights that aid problem solving.
Miceli and Susinno (2003, 2004) use cluster analysis to classify various hedge funds based on the fund returns. They argue that clustering can be used to monitor potential style drifts, conduct peer group analysis, and identify a proper benchmark for groups of funds. They argue that clusters are easier to interpret than large correlation matrices and can be helpful during portfolio construction.
Martin (2000) analyzes monthly returns for hedge funds and concludes that there is significant heterogeneity in individual fund returns within clusters, so aggregate data are likely to be only weakly applicable to individual funds. Kazemi, Gupta, and Daglioglu (2003) argue that a principal components analysis on returns of global strategy funds revealed a structure that was different from that of standard equity and hedge fund indices. Das (2003) clustered managers based on asset class, style of hedge fund, incentive fee, risk level, and liquidity. She found that the cluster analysis was more successful than the ZCM/Hedge classification in categorizing manager return histories.
Gibson and Gyger (2007) conclude that clustering analysis shows that certain managers do not follow their investment style consistently over time. They also use fuzzy clustering to illustrate the degree of misclassification that exists in the industry-accepted investment-style classifications.
In this paper, we show how cluster analysis can reveal important insights into a current issue: portfolio management behavior during the financial crisis of 2008–2009. Cluster analysis can supplement the classic tools of qualitative management interviews and this tool can, over time, help us to better understand a manager’s exposures and how a group of managers adapts to changing market opportunities. To permit greater focus on the discussion of our method, we concentrate on strategies utilized by managed futures hedge funds (also referred to as Commodity Trading Advisors, or CTAs). We construct our initial analysis by looking at monthly returns from January 2004 to December 2007 and at our out-of-sample comparison between January 2008 and March 2009.
To conduct our analysis, we employ several statistical tools. We use cluster analysis as our main tool to separate various CTA strategies into a number of categories and examine how those categories change during different time periods.
Data and Methodology
During the last decade, funds of hedge funds have become increasingly popular with the investors who look to allocate capital to hedge funds, but do not have the resources to research, monitor, and manage a number of stand-alone hedge fund investments. Despite the fact that the fund of funds sector has been substantially affected by the current financial crisis, fund of funds’ investment in hedge funds still accounted for an estimated $606 billion of the $2.1 billion hedge fund industry at the end of the fourth quarter of 2009 (according to BarclayHedge Alternative Investment Databases).
Funds of hedge funds vary in their mix of qualitative and quantitative methodologies they use to construct portfolios. The qualitative methods include in-depth interviews of the manager’s investment style and operations. Quantitative methods include the calculation of the Sharpe ratio and other performance measures, portfolio optimization, and analysis of the correlation matrix of returns. Rules governing maximum exposures to specific investment strategies are also often used to encourage portfolio diversification.
Dividing the world into specific investment strategies requires a well-established set of categories or factors that are easily identified and are known to be good predictors of the pattern of returns. In parts of the hedge fund universe these categories have been created and successfully implemented. Jaeger and Wagner (2005) argue that each fund style has three or four factors that explain a statistically significant portion of returns. These factors can be used to build replicating factor strategies at lower fee levels. Additionally, the returns of the replicating strategies can be useful to the fund of funds managers to measure the skill of hedge fund managers.
However, some strategies are heterogeneous, and organizing hedge funds into static groups may be problematic during periods of financial stress. For these reasons, we have found it useful to supplement our qualitative-style due diligence by grouping managers who have similar performance histories. We have found cluster analysis to be especially useful for building peer groups and examining the change in strategies over time.
The hedge fund returns used in this study were extracted from the Barclay Managed Futures (CTA) Database, which is one of the most comprehensive hedge fund return databases providing information on hedge funds in the managed futures space.
We selected 59 CTA managers for our analysis. We wanted to include funds that represented the full spectrum of managed futures strategies, as well as various time frames and markets. To that end, we included managers from each of the three investment strategies:
- Systematic CTA managers conduct their trading based on signals generated by a computer program or system. Most systematic CTAs tend to use technical indicators in their models. Once the models are in place, a pure systematic CTA does not require any additional human input regarding trading decisions.
- Discretionary CTA managers use their own decision-making skills to determine when to enter and exit trades. Some discretionary managers tend to rely more on fundamental analysis than technical analysis.
- Hybrid CTA managers use a combination of systematic and discretionary approaches. This usually consists of screening the investment universe by a computer algorithm, and then using a manager’s discretion to make the final trading decision.
All funds in our study had return histories going back to at least January 2004. Each fund had assets under management of at least $10 million. Additionally, all candidate funds met two criteria: (1) we had interviewed the fund’s manager, and (2) the fund had a high level of familiarity and transparency in regards to the investment process and the stated exposures and expertise of the manager. Further details of the characteristics of the managers selected for the study are presented in Table 1.
Table 1: Descriptive Statistics on the 59 Programs Selected as of January 2008
Clustering Methods
Clustering methods can be divided into various groups based on their procedures for arriving at clusters and the criterion used to evaluate whether funds cluster together. The clustering methods can be divided into two main categories of hierarchal and nonhierarchical methods. Agglomerative methods and divisive methods are the two hierarchical clustering techniques. Agglomerative methods start with clusters consisting of individual managers and combine similar clusters until all funds are grouped in a single cluster. Divisive methods proceed in the opposite direction, starting with all funds in a single cluster and cleaving until each cluster contains a single fund. Nonhierarchical methods start with a fixed number of target clusters and attempt to group all funds into these target clusters.
To group the funds, we wanted a cluster procedure that did not require us to specify in advance the number of the clusters or their size. Also we wanted a method that would be robust to the specifics of alternative measures of fund similarity and would generate tight clusters in which all members of the cluster were more similar to each other than to any nonmembers. This is in contrast to methods in which members might be very different but be grouped due to common intermediate links.
For these reasons we used a popular method called complete-linkage agglomerative cluster analysis. The analysis proceeds using the following steps:
- Start the process with each manager as its own cluster.
- Join the two closest clusters into a new cluster with the difference between the managers defined as one minus the correlation. The higher the correlation, the closer the clusters: di(j,k) = max(dij, dik)
- Recalculate the distance between the new cluster and all other clusters (maximum distance).
- Repeat steps 2 and 3 until all managers are grouped into a single cluster, or you are satisfied that you have a set of clusters that are most reflective of the data characteristics.
Each clustering method also requires a criterion for determining the similarity of funds so that funds can be clustered. Similarity measures depend on the types of input data and the goals of the clustering. For some characteristics, Euclidean distance measures can be used. For discrete characteristics, a count of the number of overlapping characteristics, also known as the Hamming distance, can be used. We used the one minus the correlation matrix of the pattern of historical returns, because correlation is a commonly used measure of association for comparing performance histories. Correlations also emphasize the timing of the relative best and worst performance controlling for volatility.
The results of the cluster analysis appear in Figure 1 (see above).
We next labeled funds that were grouped together to determine how the groupings lined up with our qualitative assessments of each manager and if there were any indications of the number of groups. We identify five major clusters within our data set. From our due diligence interviews we extracted the main characteristics of the managers comprising each cluster in Figure 1. The groupings allowed us to focus on the bigger picture of different sensitivities to market events.
Table 2: Main Characteristics of Clusters
Cluster A
- Systematic trend followers Multiple time frames
Cluster B
- Single sector (e.g. energy) Discretionary/hybrid
Cluster C
- Long-term systematic trend followers Emphasize financial commodities Globally diversified Use daily data (open-high-low-close)
Cluster D
- Multiple time frames Fundamentally driven systematic approach
Cluster E
- Discretionary Short-term time horizon Mean reversion/pattern recognition/counter trend Technical analysis