Predicting Material Accounting Misstatements
Post on: 25 Апрель, 2015 No Comment
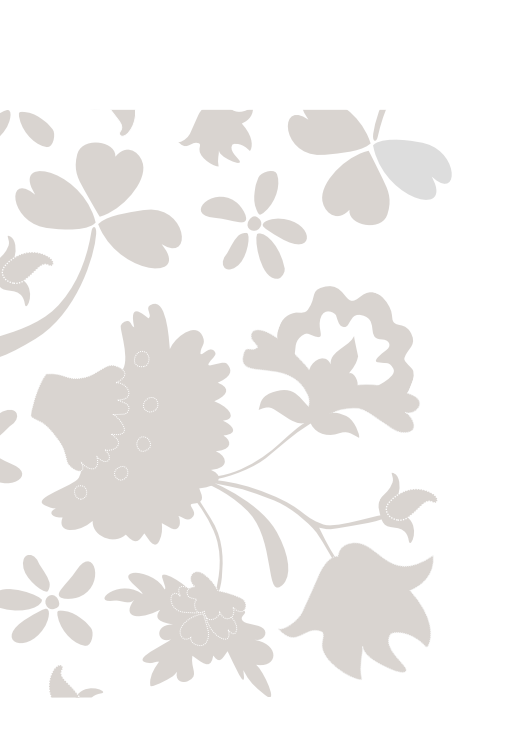
Page 1
Predicting Material Accounting Misstatements*
Patricia M. Dechow
The Haas School of Business
University of California, Berkeley
Berkeley, CA 94705
patricia_dechow@haas.berkeley.edu
Weili Ge
Michael G. Foster School of Business
Richard G. Sloan
The Haas School of Business
University of California, Berkeley
Berkeley, CA 94705
richard_sloan@haas.berkeley.edu
First version: January 8, 2007
This version: April 21, 2010
Contemporary Accounting Research, forthcoming
* We appreciate the comments of the workshop participants at the University of Michigan, the UBCOW Conference
at the University of Washington, New York University 2007 Summer Camp, University of California, Irvine and
University of Colorado at Boulder, Columbia University, University of Oregon, the Penn State 2008 Conference,
University of California, Davis 2008 Conference, American Accounting Association meetings 2007, FARS 2008
meetings, the University of NSW Ball and Brown Conference in Sydney 2008 and the 2009 George Mason
University Conference on Corporate Governance and Fraud Prevention. We thank Ray Ball, Sid Balachandran,
Sandra Chamberlain, Ilia Dichev, Bjorn Jorgensen, Bill Kinney, Carol Marquardt, Mort Pincus, and Charles Shi for
their comments and Seungmin Chee for research assistance. We would like to thank the Research Advisory Board
established by Deloitte & Touche USA LLP, Ernst & Young LLP, KPMG LLP and PricewaterhouseCoopers LLP
for the funding for this project. However, the views expressed in this article and its content are those of the authors
alone and not those of Deloitte & Touche USA LLP, Ernst & Young LLP, KPMG LLP or PricewaterhouseCoopers
LLP. Special thanks go to Roslyn Hooten for administering the funding relationship. This paper is dedicated to the
memory of our colleague, friend and research team member Nader Hafzalla, who was a joy to all who knew him.
Page 2
Predicting Material Accounting Misstatements
Abstract
We examine 2,190 SEC Accounting and Auditing Enforcement Releases (AAERs) issued
between 1982 and 2005. We obtain a comprehensive sample of firms that are alleged to have
misstated their financial statements. We examine the characteristics of misstating firms along
five dimensions: accrual quality, financial performance, non-financial measures, off-balance
sheet activities, and market-based measures. We compare misstating firms to themselves
during non-misstatement years and misstating firms to the broader population of all publicly
listed firms. We find that managers appear to be hiding diminishing performance during
JEL Classifications: G3, M4
Page 3
1. INTRODUCTION
What causes managers to misstate their financial statements? How best can investors,
auditors, financial analysts and regulators detect misstatements? Addressing these questions is
of critical importance to the efficient functioning of capital markets. For an investor it can lead
to improved returns, for an auditor it can mean avoiding costly litigation, for an analyst it can
mean avoiding a damaged reputation, and for a regulator it can lead to enhanced investor
protection and fewer investment debacles. Our research has two objectives. First, we develop a
comprehensive database of financial misstatements. Our objective is to describe this database
and make it broadly available to other researchers to promote research on earnings
misstatements. Second, we analyze the financial characteristics of misstating firms and develop
a model to predict misstatements. The output of this analysis is a scaled probability (F-Score)
that can be used as a red flag or signal of the likelihood of earnings management or
“misstatement.”
released between 1982 and 2005. Our examination identifies 676 unique firms that have
misstated at least one of their quarterly or annual financial statements.1
Using AAERs as a source to investigate characteristics of firms that manipulate financial
statements has both advantages and disadvantages. The SEC has a limited budget, so it selects
firms for enforcement action where there is strong evidence of manipulation. Firms selected
often have already admitted a “mistake” by restating earnings or having large write-offs (e.g.
Enron or Xerox); other firms have already been identified by the press or analysts as having
misstated earnings (see Miller 2006); in addition, insider “whistleblowers” often reveal problems
directly to the SEC. Therefore, one advantage of the AAER sample is that researchers can have
a high level of confidence that the SEC has identified manipulating firms (the Type I error rate is
low). However, one disadvantage is that many firms that manipulate earnings are likely to go
unidentified, and a second disadvantage is that there could be selection biases in cases pursued
by the SEC. For example, the SEC may be more likely to pursue cases where stock performance
declines rapidly after the manipulation is revealed, since the identifiable losses to investors are
greater. Selection biases may limit the generalizability of our results to other settings. It is
worth noting, however, that problems with selection bias exist for other samples of
“manipulators” identified by an external source (e.g. shareholder litigation firms, SOX internal
control violation firms, or restatement firms).2 Bias concerns also exist for discretionary accrual
measures (Dechow, Sloan, and Sweeney 1995). Thus selection bias is a general concern when
analyzing the determinants of earnings manipulation and is not unique to AAER firms.
1 Throughout the paper we use the terms earnings management, manipulation, and misstatement interchangeably.
Although fraud is often implied by the SEC’s allegations, we use the term misstatement since firms and managers
typically do not admit or deny guilt with respect to the SEC allegations.
2 Shareholder lawsuit firms are biased towards firms that have had large stock price declines, SOX internal violation
firms are biased towards younger firms with less developed accounting systems, and restatement firms are biased
towards firms that have made a mistake that is not necessarily intentional.
Page 5
In our tests we focus on variables that can be easily measured from the financial
statements since we want our analysis to be applicable in most settings facing investors,
regulators, or auditors. Our tests focus only on AAER firm-years that have overstated earnings.
We examine (i) accrual quality, (ii) financial performance, (iii) non-financial measures, (iv) off-
balance sheet activities, and (v) market-based measures for identifying misstatements.
We investigate several measures of accrual quality. We examine working capital
accruals and the broader measure of accruals that incorporates long-term net operating assets
(Richardson, Sloan, Soliman and Tuna 2005). We provide an analysis of two specific accruals:
changes in receivables and inventory. These accounts have direct links to revenue recognition
and cost of goods sold, both of which impact gross profit, a key performance metric. We
measure the percent of “soft” assets on the balance sheet (defined as the percent of assets that are
neither cash nor PPE). We predict that the more assets on the balance sheet that are subject to
changes in assumptions and forecasts, the greater the manager’s flexibility to manage short-term
earnings (e.g. Barton and Simko 2002; Richardson et al. 2005). We find that all measures of
accrual quality are unusually high in misstating years relative to the broad population of firms.
We also find that the percent of soft assets is high, which suggests that manipulating firms have
more ability to change and adjust assumptions to influence short-term earnings.
In time-series tests that focus only on misstating firms, we find that the reversal of
accruals is particularly important for detecting the misstatement. We find that in the years prior
to the manipulation, all accrual measures are unusually high and in fact are not significantly
different from those of manipulation years. There are two explanations for this finding. First,
managers are likely to utilize the flexibility within GAAP to report higher accruals and earnings
before resorting to the aggressive manipulation identified by the SEC. Therefore, growing
Page 6
accruals in earlier years is consistent with “within GAAP” earnings management. Second, the
positive accruals in earlier years could reflect an over-investment problem. Managers in
misstating firms could be relaxing credit policies, building up inventory and fixed asset capacity
in anticipation of future growth. When that growth is not realized, managers then resort to the
manipulation identified by the SEC. The two explanations are not mutually exclusive, since a
manager who is optimistic and over-investing is also likely to be optimistic in terms of
assumptions and forecasts that relate to asset values and earnings.
We examine various models of discretionary accruals developed in prior accounting
research including the cross-sectional modified Jones model (Dechow et al. 1995; DeFond and
Jiambalvo 1994), the performance matched discretionary accruals model (Kothari, Leone, and
Wasley 2005), and a “signed” version of the earnings quality metric developed by Dechow and
Dichev (2002). Our results indicate that the residuals from the modified Jones model and the
performance matched Jones model have less power to identify manipulation than unadjusted
accrual measures (i.e. working capital accruals and the broader measure of accruals) or the
“signed” Dechow and Dichev model. This suggests that conventional approaches of controlling
for industry and performance induce considerable estimation error into the estimation of
discretionary accruals.
We examine whether the manipulations occur to hide diminishing firm performance. We
find that returns on assets are generally declining; however, contrary to our initial expectations,
we find that cash sales are increasing during misstatement periods. We failed to anticipate the
cash sales result because we expected firms to boost sales by overstating credit sales. There are
two explanations for the unexpected cash sale result. First, misstating firms tend to be growing
their capital bases and increasing the scale of their business operations. The greater scale of
Page 7
operations should lead to increases in both cash and credit sales. Second, an inspection of the
AAERs reveals that many firms misstate sales through transaction management — for example,
encouraging sales to customers with return provisions that violate the definition of a “sale,”
selling goods to related parties, or forcing goods onto customers at the end of the quarter.
We find that one non-financial measure, abnormal reductions in the number of
employees, is useful in detecting misstatements. This measure is new to the literature and is
measured as year-over-year change in employee headcount less year-over-year change in total
assets. This result can be interpreted in two ways. First, reductions in the number of employees
are likely to occur when there is declining demand for a firm’s product. In addition, cutting
employees directly improves short-run earnings performance by lowering wage expenses.
Second, if physical assets and employees are complements, then a decrease in employees relative
to total assets could signal overstated asset balances.
Our examination of off-balance sheet information focuses on the existence and use of
operating leases and the expected return assumption on plan assets for defined benefit pension
management options before resorting to more aggressive financial misstatements.
Our final set of variables relates to stock and debt market incentives. Dechow et al.
(1995) suggest that market incentives are an important reason for engaging in earnings
Page 8
management. Teoh, Welch, and Wong (1998) and Rangan (1998) provide corroborating
evidence that accruals are unusually high at the time of equity issuances. However, the evidence
in Beneish (1999b) suggests that leverage and stock issuances do not motivate misstatements.
Therefore, revisiting this question using our more comprehensive data is warranted. We find that
the comparison group is critical for evaluating whether raising financing is a motivation for the
misstatement. Inconsistent with Beneish, we find that misstating firms are actively raising
financing in misstating years relative to the broad population of firms. However, consistent with
Beneish, we find no significant difference in the extent of financing when we compare earlier
years to manipulation years for the same AAER firm. These results can be reconciled by the fact
that we find misstating firms are actively raising financing before and during the manipulation
period. Thus, one interpretation of these findings is that managers of misstating firms are
concerned with obtaining financing and this motivates earnings management in earlier years, as
well as the more aggressive techniques identified by the SEC in misstating years. Also
consistent with Beneish, we do not find evidence that misstating firms tend to have higher
financial leverage than non-misstating firms.
We examine the growth expectations embedded in misstating firms’ stock market
valuations. We find that the price-earnings and market-to-book ratios are unusually high for
misstatement firms compared to other firms, suggesting that investors are optimistic about the
future growth opportunities of these firms. We also find that the misstating firms have unusually
strong stock return performance in the years prior to misstatement. This is consistent with
managers engaging in aggressive techniques in misstating years in the hopes of avoiding
disappointing investors and losing their high valuations (Skinner and Sloan 2000).
Our final tests aim at developing a prediction model that can synthesize the financial
statement variables that we examine and provide insights into which variables are relatively
more useful for detecting misstatements. The model is built in stages based on the ease of
obtaining the information, and compares the characteristics of misstating firm-years to other
public firms. Model 1 includes variables that are obtained from the primary financial statements.
These variables include accrual quality and firm performance. Model 2 adds off-balance sheet
and non-financial measures. Model 3 adds market-related variables. The output of these models
is a scaled logistic probability for each firm-year that we term the F-Score.
We show that while only 20 percent of the public firms have an F-Score greater than 1.4,
over fifty percent of misstating firms have F-Scores of 1.4 or higher. We also investigate the
time-series pattern of F-Scores for misstating firms. We show that average F-Scores for
misstating firms increase for up to three years prior to the misstatement, but decline rapidly to
more normal levels in the years following the misstatement. This is consistent with the F-Score
identifying within-GAAP earnings management as well as the more aggressive techniques
identified by the SEC. We discuss interpretation issues concerning Type I and Type II errors
related to the F-Score, and provide marginal analysis and sensitivity analysis showing that
variation in the F-Score is not driven by one specific variable. We also conduct several
robustness tests that confirm the stability of the variables selected for our models, our coefficient
estimates and the predictive ability of the F-Score over time.
The remainder of the paper is organized as follows. Section 2 reviews previous research
on this topic. Section 3 describes database construction and research design. Section 4 presents
our analysis of misstatement firms and develops our misstatement-prediction model. Section 5
concludes.
2. PREVIOUS LITERATURE
Understanding the types of firms that will misstate financial statements is an extensive
area of research. We briefly discuss some of the key findings but do not attempt to document all
literature examining characteristics of AAER firms. Dechow, Ge, and Schrand (2009) provide a
comprehensive review of this literature.
Early work by Feroz, Park, and Pastena (1991) examines 224 AAERs issued between
April 1982 and April 1989 covering 188 firms, of which 58 have stock price information. Feroz
et al. document that receivables and inventory are commonly misstated. Two pioneering papers
analyzing misstating firms are Beneish (1997 and 1999a). Beneish (1997) analyzes 363 AAERs
covering 49 firms and a further 15 firms whose accounting was questioned by the news media
between 1987 and 1993. The 64 firms are classified as manipulators. He creates a separate
sample of firms using the modified Jones model to select firms with high accruals that he terms
“aggressive accruers”. His objective is to distinguish the manipulators from the aggressive
accruers. Beneish (1997) finds that accruals, day’s sales in receivables and prior performance
are important for explaining the differences between the two groups. Beneish (1999a) matches
the sample of manipulators to 2,332 Compustat non-manipulators by two-digit SIC industry and
year for which the financial statement data used in the model were available. For seven of the
eight financial statement ratios that he analyzes, he calculates an index, with higher index values
indicating a higher likelihood of an earnings overstatement. Beneish shows that the day’s sales
in receivables index, gross margin index, asset quality index, sales growth index, and accruals
(measured as the change in non-cash working capital plus depreciation) are important. He
provides a probit model and analyzes the probability cutoffs that minimize the expected costs of
misstatements.
Page 11
Our research builds on and is complementary to Beneish (1997, 1999a). We take a
different perspective from Beneish that leads us to make a number of different choices.
However, such differences should not be viewed as a critique of his approach; rather, they stem
from our objectives. One of our objectives is to develop a measure that can be directly calculated
from the financial statements. Therefore, we do not use indexes for any of our variables. A
second objective is to enable researchers and practitioners to calculate an F-Score for a random
firm and to easily assess the probability of misstatement. Therefore, we do not match AAER
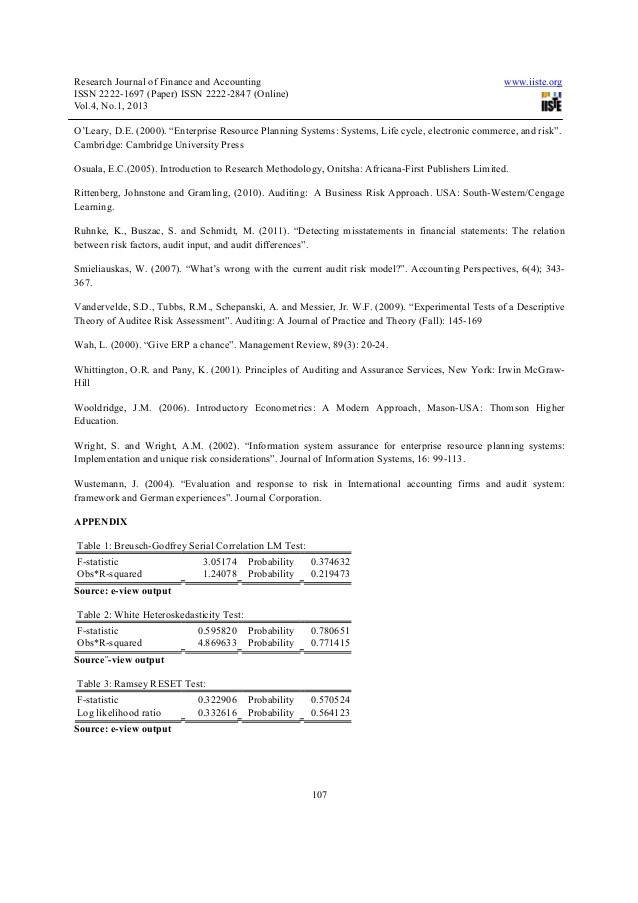
firms to a control group by industry or size. Matching by industry and size provides information
on whether a variable is significantly different relative to a control firm. However, it is more
difficult when matching to determine Type I and Type II error rates that users will face in an
unconditional setting. Models could be developed for individual industries and size categories.
We choose not to do this since it would add greatly to the complexity of our analysis and the
presentation of our results. A third objective is to evaluate the usefulness of financial statement
information beyond that contained in the primary financial statements; therefore we include other
information disclosed in the 10-K either in Item 1 (discussion of the business), Item 5 (stock
price information), or the footnotes.
Concurrent research provides additional insights into variables that are useful for
detecting misstatements. Ettredge, Sun, Lee, and Anandarajan (2006) examine 169 AAER firms
matched by firm size, industry and whether the firm reported a loss. They find that deferred
taxes can be useful for predicting misstatements, along with auditor change, market-to-book,
revenue growth and whether the firm is an OTC firm. Brazel, Jones, and Zimbelman (2009)
examine whether several non-financial measures (e.g. number of patents, employees, and
products) can be used to predict misstatement in 50 AAER firms. They find that growth rates
between financial and non-financial variables are significantly different for AAER firms. Bayley
and Taylor (2007) study 129 AAER firms and a matched sample based on industry, firm size and
time period. They find that total accruals are better than various measures of unexpected
accruals in identifying material accounting misstatements. In addition, they find that various
financial statement ratio indices are incrementally useful. They conclude that future earnings
management research should move away from further refinements of discretionary accrual
models and instead consider supplementing accruals with other financial statement ratios. We
agree with Bayley and Taylor and view our work as moving in the direction that they
recommend.
There has also been work using AAER firms to examine the role of corporate governance
and incentive compensation in encouraging earnings manipulation (see, for example, Dechow,
Sloan and Sweeney (1996), Beasley (1996), Farber (2005), Skousen and Wright (2006) and, for a
summary, Dechow et al. (2009)). We chose not to investigate the role of governance variables
and compensation because these variables are available for only limited samples or must be hand
collected. Therefore, adding these variables would have limited our analysis to a smaller sample
with various biases in terms of data availability. However, a useful avenue for future research is
to analyze the role of governance, compensation, insider trading, short-selling, incentives to meet
and beat analyst forecasts, etc. and to determine the relative importance of these variables over
financial statement information in detecting overstatements of earnings.
3. DATA AND SAMPLE FORMATION
3.1 Data Sample
The objective of our data collection efforts is to construct a comprehensive sample of
material and economically significant accounting misstatements involving both GAAP violations
Page 13
and the allegation that the misstatement was made with the intent of misleading investors. Thus
we focus our data collection on the SEC’s series of published Accounting and Auditing
Enforcement Releases.3
The SEC takes enforcement actions against firms, managers, auditors and other parties
involved in violations of SEC and federal rules. At the completion of a significant investigation
involving accounting and auditing issues, the SEC issues an Accounting and Auditing
Enforcement Release (AAER). The SEC identifies firms for review through anonymous tips and
news reports. Another source is the voluntary restatement of the financial results by the firm
itself, since restatements are viewed as a red flag by the SEC. The SEC also states that it reviews
about one-third of public companies’ financial statements each year and checks for compliance
with GAAP. If SEC officials believe that reported numbers are inconsistent with GAAP, then
the SEC can initiate informal inquiries and solicit additional information. If the SEC is satisfied
after such informal inquiries, then it will drop the case. However, if the SEC believes that one or
more parties violated securities laws, then the SEC can take further steps, including enforcement
actions requiring the firm to change its accounting methods, restate financial statements, and pay
damages.
There are a number of conceivable alternative sources for identifying accounting
misstatements. They are discussed briefly below, along with our reasons for not pursuing these
alternatives.
3 The Accounting and Auditing Enforcement Release series began on May 17, 1982 with the SEC’s issuance of
AAER No. 1. The SEC states in the first AAER that the series would include “future…enforcement actions
involving accountants” and “enable interested persons to easily distinguish enforcement releases involving
accountants from other Commission releases” (AAER No 1). Although the AAERs often directly involve
accountants, the AAER series also includes enforcement actions against non-accountant employees that result from
accounting misstatements and manipulations.
Page 14
The GAO Financial Statement Restatement Database. This database consists of
approximately 2309 restatements between January 1997 and September 2005. This database was
constructed through a Lexis-Nexis text search of press releases and other media coverage based
on variations of the word ‘restate.’ There is some overlap between the AAER firms and the
GAO restatement firms since a) the SEC often requires firms to restate their financials as part of
a settlement, and b) restatements often trigger SEC investigations. The GAO database covers a
relatively small time period but consists of a relatively large number of restatements. The reason
for the large number of restatements is that the GAO database includes all restatements relating
to accounting irregularities regardless of managerial intent, materiality and economic
significance. Consequently, it includes a large number of economically insignificant
restatements. In addition, the results in Plumlee and Yohn (2008) suggest that many
restatements are a consequence of misinterpreting accounting rules rather than intentional
misstatements. Another shortcoming of the GAO database is that it specifies only the year in
which the restatement was identified in the press and not the reporting periods that were required
to be restated.4
Stanford Law Database on Shareholder Lawsuits. Shareholder lawsuits typically result
from material intentional misstatements. However, shareholder lawsuits can also arise for a
number of other reasons that are unrelated to financial misstatements. Shareholder lawsuits
alleging misstatements are also very common after a stock has experienced a precipitous price
decline, even when there is no clear evidence supporting the allegation. In contrast, the SEC
issues an enforcement action only when it has established intent or gross negligence on the part
of management in making the misstatement.
4 For example, while Xerox is included in the GAO database in 2002, the restatements in question relate to Xerox’s
financial statements for 1997, 1998, 1999, 2000 and 2001.
Page 15
Using the SEC’s Accounting and Auditing Enforcement Releases as a sample of
misstatement firms has several advantages relative to other potential samples. First, the use of
AAERs as a proxy for manipulation is a straightforward and consistent methodology. This
methodology avoids potential biases induced in samples based on researchers’ individual
classification schemes and can be easily replicated by other researchers. Second, AAERs are
also likely to capture a group of economically significant manipulations as the SEC has limited
resources and likely pursues the most important cases. Relative to other methods of identifying a
sample of firms with managed earnings, such as the modified Jones abnormal accruals model,
using misstatements identified in AAERs as an indicator is expected to generate a much lower
Type I error.
Despite the advantages of using AAERs to identify accounting misstatements, there are
caveats. We can investigate only those firms identified by the SEC as having misstated earnings.
The inclusion of the misstatements that are not identified by the SEC in our control sample is
likely to reduce the predictive ability of our model. Therefore, our analyses can be interpreted as
joint tests of engaging in an accounting misstatement and receiving an enforcement action from
the SEC. If it is assumed that the SEC selection criteria are highly correlated with our prediction
variables, then another criticism is that identified variables could reflect SEC selection.
However, as noted above, the SEC identifies firms from a variety of sources and not just from its
own internal reviews, and many cases are brought to its attention because the firm itself either
restates or take a large write-off. Thus, selection choices are unlikely to be a complete
explanation for our findings. In addition, from a firm’s perspective, being subject to an SEC
enforcement action brings significantly negative capital market consequences (Dechow et al.,