Journal of Asset Management Optimal asset allocation for sovereign wealth funds
Post on: 10 Апрель, 2015 No Comment
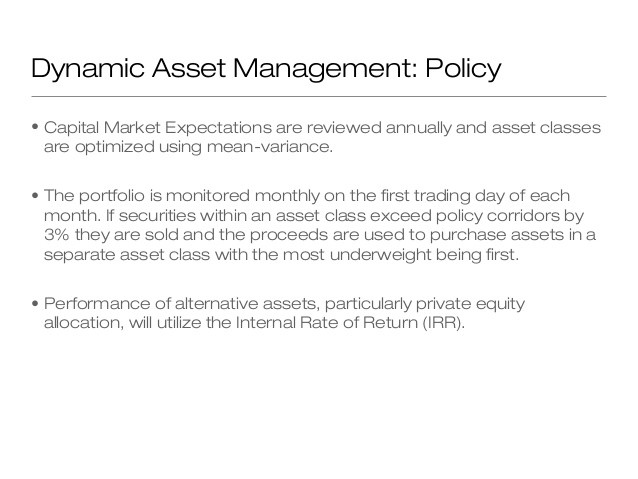
Journal of Asset Management (2008) 9, 215–238. doi:10.1057/jam.2008.19
Optimal asset allocation for sovereign wealth funds
Andreas Gintschel 1 and Bernd Scherer 2
Correspondence: Bernd Scherer, Morgan Stanley, Investment Management, 25 Cabot Square, Canary Wharf, Floor 07, London E14 4QA, UK. Tel: 44 20 7425 4016; E-mail: Bernd.Scherer@morganstanley.com
1 is an executive director at the investment banking arm of JPMorgan, advising European pension funds and insurance companies on strategic issues related to risk and capital management and asset management. Previously, he held various positions with Deutsche Bank’s Asset Management and Investment Banking Divisions, as well as Group Treasury, and was Assistant Professor of Finance at Emory University’s Business School. Andreas holds a MS and PhD in Finance and Accounting from the University of Rochester, NY.
2 is MD and global head of Quantitative GTAA at Morgan Stanley. Prior to joining the firm, Bernd worked at Deutsche Bank Asset Management as head of the Quantitative Strategies Group’s Research Center as well as Head of Portfolio Engineering in New York. He authored and edited six books on quantitative asset management and various articles in refereed journals. Bernd received Master’s degrees in Economics from the University of Augsburg and the University of London and a PhD in Finance from the University of Giessen. He is a visiting professor at Birkbeck College (London) as well as WHU (Koblenz) and external adviser to the Swiss Finance Institute.
Received 29 April 2008; Revised 29 April 2008.
Abstract
This paper develops a framework for partially hedging the market risk of oil reserves through appropriately allocating financial assets for Sovereign Wealth Funds, in particular so-called ‘oil revenue’ or ‘petroleum’ funds. Empirically, the hedge potential is substantial even when using relatively coarse partitions of the investment universe, such as Morgan Stanley Capital International (MSCI) country or industry indices. For example, if the market values of oil reserves and financial funds are equal, risk reduction is by as much as 50 per cent (10 per cent if short sales are not allowed) from original levels, translating into a certainty equivalent return of 3.26 per cent pa (48 basis points if short sales are not allowed). Moreover, choosing a portfolio along the efficient frontier, which is typically viewed as the key task in asset allocation, is relatively unimportant compared to the hedge decision.
Keywords:
sovereign wealth funds, asset allocation, nontradeable asset, conditional Value at Risk, portfolio optimisation, oil price
Introduction: Oil revenue funds as subclass of sovereign wealth funds
For the purpose of this paper, we define Sovereign Wealth Funds (SWF) as sovereign vehicles (returns enter the governments fiscal budget) with high foreign asset exposure, nonstandard liabilities (could be as simple as wage growth or as complicated as maintaining external purchasing power) with long (generation spanning) time horizon. 1 In this paper, we focus on SWF sourced by oil revenue as the currently most important (biggest) fraction of this class of new investors, as can be seen from Table 1 .
Among the ten biggest SWF we find eight funds that are sourced from oil revenues. Given an estimated market size of about 3tn dollars at the beginning of 2008, the three biggest oil revenue funds account for 52 of total SWF assets. Given the long-term mediocre performance of spot oil (underground wealth), SWF have been created to perform an oil to equity transformation to participate in global growth. The speed of this transformation will depend on the optimal patch of extraction, which in turn depends on the impact of increased supply on oil prices, extraction costs (technology) and oil price expectations. Given an estimated 40tn dollar value of underground oil, compared to 50tn dollars in global equities, SWF will have a major impact on global equity markets. It will also lead to a shift from traditional reserve currencies (dollar, yen) to emerging market currencies, where much of the global growth is expected.
For many oil exporting countries, crude oil or gas reserves are their single most important national asset. Any change in the value of reserves directly and materially affects these countries’ wealth, and thus the wellbeing of their citizens. Having recognised this, a number of oil exporting countries have been depositing oil revenues in funds dedicated to future expenditure. Devising optimal investment policies for such oil revenue funds is the aim of this paper. We analyse optimal allocations among standard partitions of the investment universe, taking into account that aggregate wealth consists of financial assets and oil reserves. We show that, in the absence of short sale constraints, the investment decision can be separated into two steps: determining (standard) efficient portfolios, and determining a zero investment, hedge portfolio, which is unique for all efficient portfolios. The positions in the hedge portfolio depend on the oil sensitivities and the covariance matrix of the assets under consideration. Empirically, we find considerable variation in oil sensitivities across assets for sufficiently fine partitions of the universe. At the global level, there is no significant variation for equity and only low variation for debt. At the country and industry level, however, we find statistically and economically significant variation in oil sensitivities. Consequently, the hedge potential is considerable, and volatility of aggregate wealth can be reduced substantially. If, for example, oil and financial assets each represent 50 per cent of aggregate wealth, the variance of aggregate wealth is nearly cut in half when using an industry stratification of the universe. This risk reduction implies 3.26 per cent in certainty equivalent return annually. In the presence of short sale constraints, however, the hedge potential is lower, and the average (across the efficient frontier) risk reduction is 6.69 per cent, implying a certainty equivalent return of 0.48 per cent. Overall, we show that the hedge potential is substantial, and produces certainty equivalent returns exceeding those of active portfolio management.
An example of an oil revenue fund is Norway’s State Petroleum Fund. The policy goals of the fund, as stated in the Norske Finansdepartementet’s (Norwegian Ministry of Finance) Summary, 2 is ‘ [ f ] irst, [ … to ] act as a buffer to smooth short-term variations in the oil revenues [ in the Fiscal Budget, … and second to ] serve as a tool for coping with the financial challenges connected to an ageing population and the eventual decline in oil revenues, by transferring wealth to future generations’. The second objective is operationalised as ‘ [ … ] invest [ ing ] the capital in such a way that the fund’s international purchasing power is maximised, taking into account an acceptable level of risk’. This suggests that the benchmark of the fund is future consumption in the form of imports. The same reason also motivates the inclusion of equity, which is expected to enhance the performance of the fund. 3 Concerning the definition of risk, it appears that the Finansdepartementet is mostly concerned with changes in the market value of the fund. We were not able to infer the Finansdepartementet’s views on operationalising the first objective, smoothing oil revenues in the short term. We believe that both objectives, smoothing revenues and maximising long-term welfare, suggest the more extensive definition of risk we propose in this paper.
As of December 2001, Norway’s Petroleum Fund had a market value of approximately USD 68bn. 4 At the same time, the estimated remaining petroleum resources were 3.7 billion tons of oil and 6,300 billion tons of gas, translating into a gross market value of approximately USD 1,143bn. During 2001, 251 million tons of oil equivalents were sold and delivered, which we value at an average price of USD 24 per barrel, or roughly USD 37bn in total. For the same period, the Norwegian Fiscal Budget shows a net cashflow of USD 27bn from petroleum activities. Thus, the government appears to be capturing about 70 per cent of revenues from oil extraction. 5 Therefore, we estimate the government’s claim on the remaining petroleum resources as 70 per cent of the gross market value, or USD 800bn as of December 2001. Thus, the value of the financial portfolio relative to the value of the claim on oil assets is currently about 8 per cent.
Other examples 6 of portfolios funded by revenues from natural resources include the Alaska Permanent Reserve Fund (funds of USD 23bn), the State Oil Fund of Azerbaijan (USD 0.5bn), Chad’s Revenue Management Fund, the National Fund of Kazakhstan (USD 1.2bn), Venezuela’s Investment Fund for Macroeconomic Stabilisation (USD 3.7bn), the Alberta Heritage Savings Trust Fund (CAD 3.7 billion), and the Nunavut Trust (CAD 0.5 billion). Furthermore, certain central bank funds of oil exporting countries, such as Iran, Kuwait, Oman, and Saudi Arabia, are defacto oil revenue funds. In general, stated investment objectives are similar to those of the Norwegian fund, that is, a favourable long-term trade-off of return and risk of the financial portfolio. The risk in aggregate wealth stemming from price changes in natural reserves is typically ignored.
More generally, our paper is an example of how risk stemming from nonfinancial assets can be hedged, at least partially, through financial assets. In other words, we talk about asset allocation with nontradeable wealth. The key is exploiting the correlation between financial and nonfinancial assets to reduce the overall risk of the portfolio, compared to an allocation that considers only the correlation structure of the financial assets. Although the general idea is straightforward, empirical or practical implementations are rare. An exception is asset / liability management, in which interest rate exposure on one side of the balance sheet is offset by interest rate exposure on the other side. This paper applies a similar idea to a more general problem.
Theoretical framework
The investor, in the present case a national government, is endowed with two assets: an oil asset, which is the investor’s claim to national oil resources, and a portfolio of financial assets. The investment policy for the portfolio is under government control. The oil asset is not necessarily under the government’s control, but a fraction of future revenues, through taxation, usually is.
Restricting the analysis to these types of assets is obviously an abstraction from reality. A more complete analysis would include additional real assets such as a country’s nonoil capital stock and human capital. In general, such an extension is straightforward. For most countries that have an oil revenue fund, however, the value of nonoil assets is small relative to the value of oil reserves, or nonoil assets are highly oil-related.
For the present, we assume that the value of oil assets and the fund changes only due to price changes of the underlying assets. In other words, we do not explicitly consider depletion of oil reserves, increases in oil reserves due to exploration, or cash flows into or out of the monetary funds. We do, however, consider a range of ratios of the value of oil resources to the value of the financial assets. Therefore, we present a dynamic, albeit myopic, asset allocation policy as oil resources are depleted and financial assets increase.
The current amount of oil resources is x 0. denominated in million barrels, and the value per barrel in U $ is p 0. The interpretation of this value depends on a variety of factors. In general, it reflects the fraction of the price per barrel that the government deposits in the financial portfolio. The total value of the government’s claim on oil resources is x o p o . The relative change in oil prices over the time period considered in the analysis is denoted as r 0 .
The current value of the monetary fund is v f . denominated in U $ million. The current relative portfolio weight on an individual asset or an asset class is w i such that ∑w i = 1. The return on a asset i over the period of the analysis is r i . The return on the portfolio is r p . Therefore, the change in total wealth, oil reserves and financial assets is r = ω r o + (1− ω )r p . where ω = x o p o / x o p o + v f . the value of oil reserves relative to aggregate wealth. For example, the Norwegian fund currently has a ratio of ω = 0.92. For ease of exposition, we assume that returns are distributed multivariate normal. This implies that expected return and volatility completely characterise portfolio return distributions. Together with constant relative risk aversion, normality implies that the standard mean-variance framework for preferences is applicable. We assume that the function u = E [ r t ] −(1 / 2) α   Var [ r t ] adequately describes the investor’s preferences, where E [ ] and Var [ ] denote the expected return and the variance, and α is the degree of risk aversion.
Oil exposure
We measure financial assets’ oil exposure by the oil sensitivity of the assets’ return, b i = Cov (r i .   r o ) / σ o 2. We estimate b i as the regression coefficient of historical asset returns on contemporaneous oil price changes and an intercept. We collect the assets’ oil sensitivities in a vector b. Accordingly, we define the oil exposure of a financial portfolio w as w T b. The variance of relative changes in aggregate wealth is
where Σ is the covariance matrix of the financial assets’ returns. The equation shows that the risk of aggregate wealth depends on the oil exposure of the financial portfolio. Since the portfolio’s oil exposure is the weighted average of the individual assets’ oil exposure, the risk of aggregate wealth depends immediately on the oil exposure of the individual assets. Holding constant the volatility of the financial portfolio, investing in assets with small oil beta reduces overall volatility.
The traditional measure of financial portfolio risk is only the last term, ignoring the weighting factor (1− ω ). Equation (1) shows that the traditional measure misestimates the risk of aggregate wealth, since it ignores the volatility of oil price and also the correlation between the prices of oil and financial assets. For any given financial portfolio, equation (1) allows us to compute the oil exposure of the portfolio by taking the average, weighted by the portfolio positions, of the individual assets’ oil betas.
Efficient portfolios
According to the standard definition, an efficient portfolio minimises the portfolio variance for a given expected return. There are, in the present application, however, two possible choices for the portfolio: the financial portfolio and the combined portfolio including oil reserves. Standard portfolio construction, as typically applied in practice, requires financial portfolios to be efficient, that is, to solve the program w T Σ w subject to achieving a target expected return μ. T w = μ. and a budget constraint 1 T w = 1. The solution, depending on the target return μ. we denote as w L (μ ). From a more comprehensive point of view, only the combined portfolio need be efficient. We call the financial portfolio, w L (μ ), which is efficient in isolation, ‘locally efficient’, while a financial portfolio w G (μ ) is ‘globally efficient’ if it yields an efficient combined portfolio, that is, solves the program
subject to the constraints 1 T w = 1 and T w = μ. These constraints do not ensure, however, that assets have generally positive weights, that is, we allow for unrestricted short positions in the financial portfolio. In our empirical analysis we relax this assumption, but we confine the theoretical discussion to the analytically tractable case of unrestricted short positions. We stress that we define the programs such that the solutions, which are financial portfolios, have the same expected return μ. Thus, for a given ratio of oil resources to value of financial assets, the combined portfolios, based on either w L (μ ) or w G (μ ), have the same expected return, independent of the expected oil price change. The advantage is that we need not make any assumptions on the expected oil price change. The return on the combined portfolio is, however, in general, different from μ. As Appendix A shows, the globally efficient portfolio for target return μ is
where
is a zero-net investment, zero-expected return hedge portfolio that does not depend on the expected target return μ. and Δ = ( T Σ −1 )(1 T Σ −1 1 )−(1 T Σ −1 )( T Σ −1 1 ). Thus, given a locally efficient portfolio for any target expected return, we can easily construct the corresponding globally efficient portfolio using the hedge portfolio w H . In other words, we have established a useful fund separation property.
The composition of the hedge portfolio is easily interpreted. The first term in equation (4) calculates the optimal hedge, the second ensures that expected portfolio returns are zero, and the third term scales portfolio weights such that the portfolio requires no net investment. Finally, equation (4) provides an answer to the important question of whether a locally efficient portfolio is also globally efficient. Inspection of equation (4) shows that necessary conditions are fairly complex. Sufficient conditions are, however, easy to state. The trivial sufficient condition is b = 0. that is, no financial asset exhibits any oil sensitivity. A more interesting condition is Σ −1 b = 0. which has the form of a system of linear equations in b. It follows that there is, for a given (nonsingular) covariance matrix, exactly one vector of oil sensitivities that satisfies the condition. In other words, the condition is unlikely to be satisfied in practice.
Measuring the benefit of reducing oil exposure
To gauge the effect of taking into account oil exposure when constructing financial portfolios, we employ two measures: the reduction in the variance of aggregate wealth, and the certainty equivalent return associated with the variance reduction. The first measure is preference free, while the second requires assumptions regarding the investor’s preferences. The variance reduction by taking into account financial assets’ oil sensitivity is
The first term is the difference between the variance of the locally efficient financial portfolio and the globally efficient financial portfolio, which is negative. The second term, after the minus sign, is the oil sensitivity of the hedge portfolio, which is also negative. Thus, the variance reduction is the result of trading off increasing volatility of the financial assets against decreasing oil sensitivity. The variance of well-diversified portfolios is typically not very sensitive to the exact portfolio composition. Thus, the first term in equation (5) is approximately zero, and, since the second term does not depend on μ.
The equation shows also that the hedge portfolio’s oil sensitivity is a useful summary measure of the risk reduction potential for a particular set of assets. The smaller the hedge portfolio’s oil sensitivity, the larger the reduction in overall, that is, financial and oil-related risk.
Since the economic importance of a variance reduction can be difficult to interpret, we present the gain in certainty equivalent return corresponding to the variance reduction. The gain in certainty equivalent return is the amount of riskless return the investor would be willing to give up in exchange for reducing risk by the specified amount, that is,
For the risk aversion parameter, we choose a moderate value of three.
Data
Here we give only a brief overview of the data and the assumption underlying the analysis. Further details are in Appendix B. As proxies for financial assets, we use standard, broad asset classes. Using widely diversified portfolios reduces estimation error in the covariance matrix of asset returns and in oil sensitivities. On a more practical level, restricting the investable universe to standard asset classes facilitates implementation within existing investment processes.
As examples, we work with four different investable universes, each comprising government debt and different stratifications of developed market equity. For global government debt, we use standard benchmarks for the three major economic blocks: EMU, Japan, and US. For equity, we use the MSCI developed market indices in four different stratifications: major economic blocks (Europe, North America, and Pacific), countries, industry sectors, and industries. As an estimate of the covariance matrix, we use the historical covariance matrix. Expected returns on government debt are the yields corrected for currency effects. We estimate the expected returns for equity as the expected return implied by market capitalisation weights. All return series are in local currency, which is equivalent to assuming that currency risk is perfectly hedged.
To model oil price risk, we rely on historical data for Dated Brent Crude Oil available from Independent Commodity Information Services (ICIS). In the next section, we describe the estimator of assets’ oil price sensitivities and discuss results. As we note in the theoretical development, we can dispense with estimating expected returns for oil.
Oil sensitivity
Table 2 contains evidence on the invidual asset classes’ oil sensitivity, which we estimate as the slope coefficients in univariate time-series regressions
where a i is the intercept and u i , t is the error term in the regression. Next to the estimate of the slope coefficient, we report standard errors. Slope coefficients that are significantly different from zero are typeset in bold face. From the theoretical analysis above, oil sensitivities that are large in absolute magnitude and widely dispersed indicate substantial hedging potential.
Scanning Table 1. panels A and B reveal that oil sensitivity for sovereign debt and regional equity is generally low, negative, and not very dispersed. At the global level, oil price increases affect financial assets’ prices negatively, as expected. The magnitude of the effect is surprisingly small, given the considerable importance that economists usually ascribe to oil price changes. Thus, allocating among these broad asset classes promises few advantages in hedging oil price risk. Turning to the country stratification in panel C, more interesting results emerge. For some countries, we find statistically and economically significant oil sensitivities. In particular, for Belgium, France, Ireland, and Spain oil sensitivities are significantly negative. For countries rich in natural resources, such as Australia, Canada, and Norway, we find positive, albeit statistically insignificant oil sensitivities. Overall, these results suggest that oil sensitivities are sufficiently dispersed as to have an impact on asset allocation. For the sector stratification in panel D, we find widely different oil sensitivities across industrial sectors. In particular, the energy and information technology sectors exhibit statistically and economically positive oil sensitivity, that is, these sectors’ equity prices move in the same direction as oil prices to a considerable degree. In contrast, sectors such as consumer staples or utilities have negative oil price sensitivity. Of course, the industry stratification, which is just a finer partition of the sector stratification, exhibits similar patterns. For some industries, oil sensitivities are surprising. For example, the automobile industry has virtually zero oil sensitivity (0.0009), while the media sector has a substantial, positive oil sensitivity (0.0792).
In Table 3. we summarise the information on oil sensitivity for the different asset classes. For each broad class, we show the arithmetic average of oil sensitivities and the corresponding standard deviation across asset classes. The standard deviation indicates the cross-sectional dispersion of the estimates, measuring the hedge potential of a particular partition of the universe. If a partition leads to only tightly dispersed oil sensitivities, reallocation among the members of this partition cannot change the oil sensitivity of the portfolio by much. Conversely, if a partition is widely dispersed, changing the allocation has a large impact on portfolio oil sensitivity.
Table 3 shows that the dispersion of oil sensitivities is, at 0.0013, very low for debt. Oil sensitivities for the regional stratification are more widely dispersed at 0.0172. Partitioning by individual country increases the dispersion to 0.0479. The dispersion of the partition based on industrial sectors is even greater at 0.0895. The industry stratification is slightly less dispersed at 0.0875. Table 2 also contains average oil sensitivities for the various asset classes and partitions. As a reference, we calculate the oil sensitivity of the market portfolio, that is, the value-weighted average of the asset classes’ oil sensitivities. Using the market values of the corresponding indices, we find an oil sensitivity of −0.0251 for the value-weighted portfolio of debt and equity. The oil sensitivity of the Norwegian Petroleum Fund is −0.0238. The last two columns show that the dispersion is statistically significant. For each asset class, we calculate the F statistic for the null hypothesis that the oil sensitivity of every asset is zero. The last column reports the corresponding p values. We cannot reject the hypothesis that the different classes of debt or regional equity have no oil sensitivity at conventional levels of statistical significance. In contrast, the oil sensitivities of the country, sector, and industry portfolios are significantly different from zero.
Hedge portfolios
In the absence of short-sale restrictions, we can easily determine globally efficient portfolios, that is, portfolios minimising overall risk for a given target expected return. As we show in the first section, for a given universe and any locally efficient financial portfolio w L (μ ) there is a unique hedge portfolio w H . A globally efficient portfolio w G (μ ) is the locally efficient portfolio w L (μ ) combined with the hedge portfolio w H . weighted according to the value of oil assets relative to financial assets.
In Table 4. we present the hedge portfolios for each investment universe. The first row contains the oil sensitivity of the hedge portfolio. The oil sensitivity of the hedge portfolio ranges from −0.0119 for the regional debt and equity stratification to −0.6162 for the industry equity universe. The oil sensitivity of the hedge portfolio for the sector stratification is, at −0.5004, slightly lower in absolute magnitude. The hedge portfolio’s oil sensitivity for the country stratification is at the intermediate level of −0.2840. Patterns of the hedge portfolio’s oil sensitivity are consistent with the dispersion of individual assets’ oil sensitivities of a particular stratification in Table 3. a wider dispersion of individual oil sensitivities translates into lower oil sensitivity of the hedge portfolio.
Table 4 also contains the weights of the individual assets in the hedge portfolio. We focus our discussion first on the simplest universe of regional debt and equity. After giving the interpretation of the numbers and describing general features for this example, we will highlight salient features for other stratifications. For regional debt and equity, the weights are −33 per cent on European debt, −4 per cent on Japanese, 40 per cent on US debt, 44 per cent on European equity, −32 per cent on North American equity, and −16 per cent on Pacific equity. It is easy to check whether this is a zero net investment portfolio. Overall, the hedge portfolio is tilted slightly towards debt, with an aggregate weight on debt of 3 per cent. 7 The aggregate weight on equity is −3 per cent, since the hedge portfolio is zero net investment. Intuitively, one might expect a direct relation between individual assets’ oil sensitivities and the weights in the hedge portfolio; holdings of assets with positive oil sensitivity, increasing overall risk, should be reduced, and holdings of assets with negative sensitivity should be increased. Interestingly, this is not necessarily the case. For example, despite having exactly the same oil sensitivity (−0.0168), displayed in the first column of Table 3. the weights on European debt (−33 per cent) and US debt (40 per cent) have the opposite sign. Similarly, the magnitude of the short position in Japanese debt (−4 per cent) is smaller than the short position in European debt (−33 per cent), although Japanese debt has the lower oil sensitivity (−0.0146). The reason for these apparently counterintuitive weights is the existence of cross-effects from the covariance matrix. Fundamentally, a financial asset in the hedge portfolio has two roles: to act as a hedge against oil risk and to hedge the financial risk stemming from another financial asset. An asset with a counterintuitive weight acts in the second capacity, hedging risk stemming from an asset that acts as a hedge against oil. This dual role of the financial assets is also highlighted in the dramatic increase, in absolute magnitude, of the hedge portfolio’s oil sensitivity as the partition of the investment universe becomes finer.
We turn now to the structure of the hedge portfolio for the other stratifications of the universe. For the country and industry stratification, the hedge portfolio is tilted towards equity, with debt weights ranging from −6 to −43 per cent. The country stratification leads to a hedge portfolio with moderate weights, the smallest of −104 per cent on the Netherlands and the largest of 69 per cent on Belgium. The sector equity universe yields more extreme portfolio weights, ranging from −280 per cent on industrials to 140 per cent on utilities. The industry stratification also delivers large weights, albeit less extreme. In general, we find hedge portfolio weights in line with oil sensitivities. Countries such as Belgium or Switzerland that have a negative exposure to oil prices receive positive weights in the hedge portfolio. On the other end of the spectrum, countries such as the Netherlands or Norway, in which natural resources companies make up a substantial fraction of market capitalisation, receive negative weights in the hedge portfolio. As we point out, however, the relation between oil sensitivity and hedge portfolio weight is not necessarily monotone. Italy, for example, has a negative oil sensitivity, yet receives a negative weight in the hedge portfolio. Similar patterns emerge for the sector and industry stratifications. Not surprisingly, energy producers receive negative weights in the hedge portfolio, while energy users, such as utilities, have positive weights. In Table 4. we also report the aggregate short positions as a measure of the hedge portfolio’s leverage. For the combination of debt and regional equity, the aggregate short positions is 84 per cent. For the industry stratification, the aggregate short position is 745 per cent. In other words, the hedge portfolios are highly geared at ratios between, roughly, one and seven.
Globally efficient portfolios
Combining the hedge portfolio and the locally efficient financial portfolio delivers the globally efficient portfolio, that is, a financial portfolio that minimises the risk of total wealth (oil and financial assets). In this section, we present and discuss the reduction in variance and the additional certainty equivalent return that switching from locally efficient to globally efficient allocations can attain. These statistics quantify the advantage of taking into account the correlation between oil and financial assets in the asset allocation process.
We determine the locally efficient frontier, ranging from 4.4 per cent expected return (the yield of debt) to 7.5 per cent expected return (the highest expected return for the coarsest partition) in increments of 10 basis points, and combine it with the hedge portfolio, weighted by the appropriate ω. delivering the globally efficient portfolio. For both the locally and the globally efficient portfolio we calculate the variance of the combined portfolio (oil and financial assets). As an example, Figure 1 contains both frontiers for ω = 50 per cent and the country stratification. On the vertical axis, we plot the expected return of the financial portfolio, and on the horizontal axis, we plot the volatility of the combined (oil and financial assets) portfolio. By construction, the globally efficient frontier plots strictly to the left (has a strictly lower volatility) of the locally efficient frontier. What is surprising, however, is the magnitude of the difference, which is roughly 3 per cent in this particular example. The graph also shows that choosing an allocation along the frontier is far less important than choosing the relevant frontier, that is, whether to hedge or not. Allocating along the locally efficient frontier, volatility of aggregate wealth ranges roughly from 20.5 to 21.5 per cent, compared to approximately 3 per cent volatility reduction moving to the globally efficient frontier.
Locally and globally efficient frontiers. Country stratification and ω = 50 per cent
Full figure and legend (41K )
From these two frontiers we proceed to calculate the relevant statistics. As an example, Figure 2 contains the gain in certainty equivalent return for the country stratification of the universe and a value of ω = 50 per cent. The gain in certainty equivalent return, around 1.7 per cent, barely varies along the efficient frontier. This is true in general, not only in this particular example. Therefore, we restrict ourselves to reporting averages of variance reductions and added certainty equivalent returns. Figure 3 contains the graph of the variance reduction scaled by the total variance of the locally efficient portfolio. Given that the variance reduction itself is stable across the frontier, not surprisingly the relative (or percentage) variance reduction varies with the variance of the portfolio. For consistency as well as brevity, we also report only the average across the frontier.