Hypothesis Testing in Finance Concept & Examples_2
Post on: 8 Июнь, 2015 No Comment
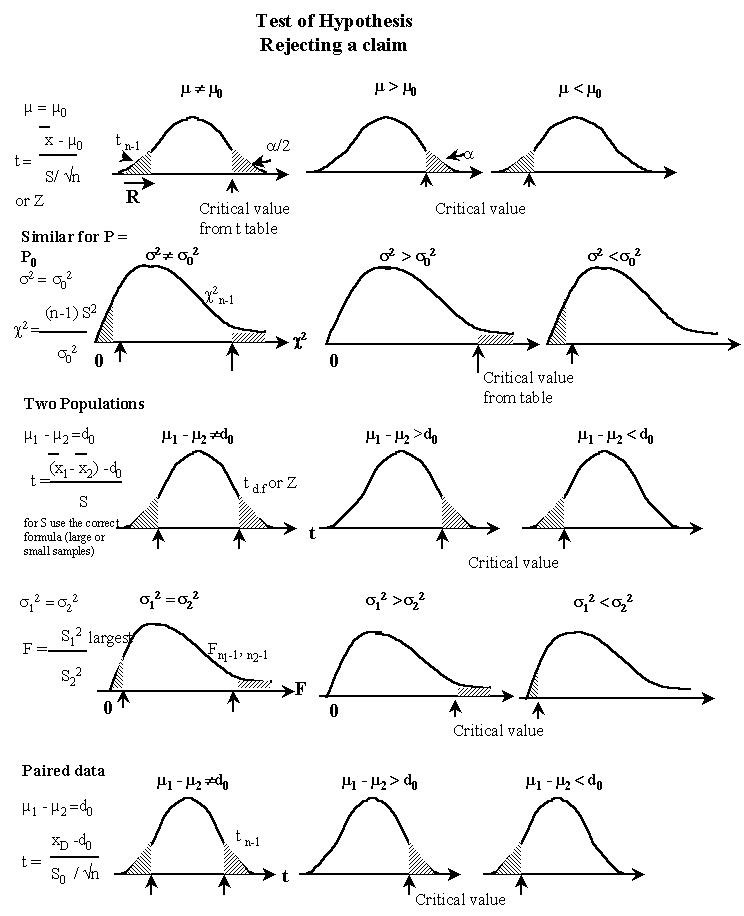
Best Results From Wikipedia Yahoo Answers Youtube
From Wikipedia
Financial statement analysis
Financial statement analysis (or financial analysis ) refers to an assessment of the viability, stability and profitability of a business. sub-business or project .
It is performed by professionals who prepare reports using ratios that make use of information taken from financial statements and other reports. These reports are usually presented to top management as one of their bases in making business decisions. Based on these reports, management may:
- Continue or discontinue its main operation or part of its business;
- Make or purchase certain materials in the manufacture of its product;
- Acquire or rent/lease certain machineries and equipment in the production of its goods;
- Issue stocks or negotiate for a bank loan to increase its working capital ;
- Make decisions regarding investing or lending capital;
- Other decisions that allow management to make an informed selection on various alternatives in the conduct of its business.
Goals
Financial analysts often assess the firm’s:
1. Profitability — its ability to earn income and sustain growth in both short-term and long-term. A company’s degree of profitability is usually based on the income statement. which reports on the company’s results of operations;
2. Solvency — its ability to pay its obligation to creditors and other third parties in the long-term;
3. Liquidity — its ability to maintain positive cash flow. while satisfying immediate obligations;
Both 2 and 3 are based on the company’s balance sheet. which indicates the financial condition of a business as of a given point in time.
4. Stability — the firm’s ability to remain in business in the long run, without having to sustain significant losses in the conduct of its business. Assessing a company’s stability requires the use of both the income statement and the balance sheet, as well as other financial and non-financial indicators.
Methods
- Past Performance — Across historical time periods for the same firm (the last 5 years for example),
- Future Performance — Using historical figures and certain mathematical and statistical techniques, including present and future values, This extrapolation method is the main source of errors in financial analysis as past statistics can be poor predictors of future prospects.
- Comparative Performance — Comparison between similar firms.
These ratios are calculated by dividing a (group of) account balance(s), taken from the balance sheet and / or the income statement. by another, for example :
n / equity =ROE Net income / total assets = return on assets Stock price / earnings per share = P/E-ratio
Comparing financial ratios is merely one way of conducting financial analysis. Financial ratios face several theoretical challenges:
- They say little about the firm’s prospects in an absolute sense. Their insights about relative performance require a reference point from other time periods or similar firms.
- One ratio holds little meaning. As indicators, ratios can be logically interpreted in at least two ways. One can partially overcome this problem by combining several related ratios to paint a more comprehensive picture of the firm’s performance.
- Seasonal factors may prevent year-end values from being representative. A ratio’s values may be distorted as account balances change from the beginning to the end of an accounting period. Use average values for such accounts whenever possible.
- Financial ratios are no more objective than the accounting methods employed. Changes in accounting policies or choices can yield drastically different ratio values.
- They fail to account for exogenous factors like investor behavior that are not based upon economic fundamentals of the firm or the general economy (fundamental analysis ) .
Financial analysts can also use percentage analysis which involves reducing a series of figures as a percentage of some base amount. For example, a group of items can be expressed as a percentage of net income. When proportionate changes in the same figure over a given time period expressed as a percentage is known as horizontal analysis. Vertical or common-size analysis, reduces all items on a statement to a “common size� as a percentage of some base value which assists in comparability with other companies of different sizes .
Another method is comparative analysis. This provides a better way to determine trends. Comparative analysis presents the same information for two or more time periods and is presented side-by-side to allow for easy analysis..
Truth value
In logic and mathematics. a logical value. also called a truth value. is a value indicating the relation of a proposition to truth .
In classical logic. the truth values are true and false. Intuitionistic logic lacks a complete set of truth values because its semantics, the Brouwer–Heyting–Kolmogorov interpretation. is specified in terms of provability conditions, and not directly in terms of the truth of formulae. Multi-valued logic s (such as fuzzy logic and relevance logic ) allow for more than two truth values, possibly containing some internal structure.
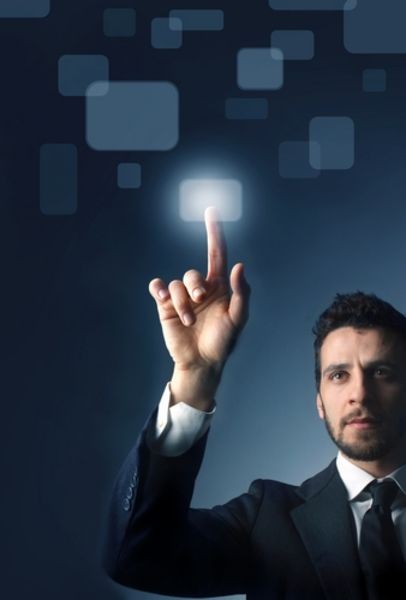
Even non-truth-valuational logics can associate values with logical formulae, as is done in algebraic semantics. For example, the algebraic semantics of intuitionistic logic is given in terms of Heyting algebra s.
Topos theory uses truth values in special sense: the truth values of a topos are the global element s of the subobject classifier. Having truth values in this sense does not make a logic truth valuational.
P-value
In statistical significance testing, the p-value is the probability of obtaining a test statistic at least as extreme as the one that was actually observed, assuming that the null hypothesis is true. The lower the p-value, the less likely the result is if the null hypothesis is true, and consequently the more significant the result is, in the sense of statistical significance. One often rejects the null hypothesis when the p-value is less than 0.05 or 0.01, corresponding respectively to a 5% or 1% chance of rejecting the null hypothesis when it is true (Type I error ).
A closely related concept is the E-value. which is the average number of times in multiple testing that one expects to obtain a test statistic at least as extreme as the one that was actually observed, assuming that the null hypothesis is true. The E-value is the product of the number of tests and the p-value.
Coin flipping example
For example, an experiment is performed to determine whether a coin flip is fair (50% chance, each, of landing heads or tails) or unfairly biased ( 50% chance of one of the outcomes).
Suppose that the experimental results show the coin turning up heads 14 times out of 20 total flips. The p-value of this result would be the chance of a fair coin landing on heads at least 14 times out of 20 flips. The probability that 20 flips of a fair coin would result in 14 or more heads can be computed from binomial coefficient s as
begin
This probability is the (one-sided) p-value.
Because there is no way to know what percentage of coins in the world are unfair, the p-value does not tell us the probability that the coin is unfair. It measures the chance that a fair coin gives such result.
Interpretation
Traditionally, one rejects the null hypothesis if the p-value is smaller than or equal to the significance level. often represented by the Greek letter α (alpha ). If the level is 0.05, then results that are only 5% likely or less, given that the null hypothesis is true, are deemed extraordinary.
When we ask whether a given coin is fair, often we are interested in the deviation of our result from the equality of numbers of heads and tails. In such a case, the deviation can be in either direction, favoring either heads or tails. Thus, in this example of 14 heads and 6 tails, we may want to calculate the probability of getting a result deviating by at least 4 from parity (two-sided test ). This is the probability of getting at least 14 heads or at least 14 tails. As the binomial distribution is symmetrical for a fair coin, the two-sided p-value is simply twice the above calculated single-sided p-value; i.e.. the two-sided p-value is 0.115.
In the above example we thus have:
- null hypothesis (H0 ): fair coin;
- observation O: 14 heads out of 20 flips; and
- p-value of observation O given H0 = Prob(≥ 14 heads or ≥ 14 tails) = 0.115.
The calculated p-value exceeds 0.05, so the observation is consistent with the null hypothesis — that the observed result of 14 heads out of 20 flips can be ascribed to chance alone — as it falls within the range of what would happen 95% of the time were this in fact the case. In our example, we fail to reject the null hypothesis at the 5% level. Although the coin did not fall evenly, the deviation from expected outcome is small enough to be reported as being not statistically significant at the 5% level.
However, had a single extra head been obtained, the resulting p-value (two-tailed) would be 0.0414 (4.14%). This time the null hypothesis – that the observed result of 15 heads out of 20 flips can be ascribed to chance alone – is rejected when using a 5% cut-off. Such a finding would be described as being statistically significant at the 5% level.
Critics of p-values point out that the criterion used to decide statistical significance is based on the somewhat arbitrary choice of level (often set at 0.05). Furthermore, it is necessary to use a reasonable null hypothesis to assess the result fairly, but the choice of a null hypothesis often entails assumptions.
To understand both the original purpose of the p-value p and the reasons p is so often misinterpreted, it helps to know that p constitutes the main result of statistical significance testing (not to be confused with hypothesis testing ), popularized by Ronald A. Fisher. Fisher promoted this testing as a method of statistical inference. To call this testing inferential is misleading, however, since inference makes statements about general hypotheses based on observed data, such as the post-experimental probability a hypothesis is true. As explained above, p is instead a statement about data assuming the null hypothesis; consequently, indiscriminately considering p as an inferential result can lead to confusion, including many of the misinterpretations noted in the next section.
On the other hand, Bayesian inference. the main alternative to significance testing, generates probabilistic statements about hypotheses based on data (and a priori estimates), and therefore truly constitutes inference. Bayesian methods can, for instance, calculate the probability that the null hypothesis H0 above is true assuming an a priori estimate of the probability that a coin is unfair. Since a priori we would be quite surprised that a coin could consistently give 75% heads, a Bayesian analysis would find the null hypothesis (that the coin is fair) quite probable even if a test gave 15 heads out of 20 tries (which as we saw above is considered a significant result at the 5% level according to its p-value).
Strictly speaking, then, p is a statement about data rather than about any hypothesis, and hence it is not inferential. This raises the question, though, of how science has been able to advance using significance testing. The reason is that, in many situations, p approximates some useful post-experimental probabilities about hypotheses, such as the post-experimental probability of the null hypothesis. When this approximation holds, it could help a researcher to judge the post-experimental plausibility of a hypothesis.
Even so, this approximation does not eliminate th
From Yahoo Answers
Question: Need help symbolizing these statements using propositions and connectives ,v, and. stating what each proposition represents. a) Regular work is sufficient to pass this course b) Regular work is not necessary to pass this course. c) If Brazil wins its 1st match only if Germany wins its 1st match, then France doesn’t win its 1st match. I would sincerely appreciate any help. Thanks..
Answers: Hi (a) ‘Regular work is sufficient to pass this course’ in effect says that you will pass the course so long as you do regular work. Let ‘R’ = ‘You do regular work’ and ‘P’ = ‘You will pass the course’. Then we can symbolize your sentence as ‘R P': if you do regular work, then you will pass the course. (b) ‘Regular work is not necessary to pass this course’ in effect says that you can pass the course without doing regular work. Again, let ‘R’ = ‘You do regular work’ and ‘P’ = ‘You will pass the course’. Then we can symbolize your sentence as ‘
(P R)': your doing regular work is not necessary for your passing the course. (c) ‘If Brazil wins its first match only if Germany wins its first match, then France doesn’t win its first match’ says that France’s not winning its first match is necessary for Brazil’s winning its first match to suffice for Germany’s winning its first match. Let ‘B’ = ‘Brazil wins its first match’, ‘G’ = ‘Germany wins its first match’, and ‘F’ = ‘France wins its first match’. Then we can symbolize your sentence as ‘(B G)
F': Germany’s winning its first match is necessary for Brazil’s winning its first match only so long as France does not win its first match. I hope that the following might help a little. ‘P Q’ says that P is a sufficient condition for Q; ‘Q P’ says that P is a necessary condition for Q. Within classical logic, the following expressions are all equivalent: ‘P is a sufficient condition for Q’ ‘Q is a necessary condition for P’ ‘P implies Q’ ‘If P, then Q’ ‘P only if Q’ ‘Q so long as P’ ‘Q provided that P’ Some people (Dorothy Edgington, for example) think that indicative conditionals (‘If. then. ‘ constructions) are not equivalent to material conditionals (expressions of the form ‘P Q’), but it’s still standard practice in logic to translate indicatives as material conditionals.
Question: Please explain about proposition in logic/mathematics and language. Also, please leave some examples as well. Thank you very much!
Answers: In mathematics, the term proposition is used for a proven statement that is of more than passing interest, but whose proof is neither profound nor difficult. The general term for proven mathematical statements is theorem, which also is used in a second, more particular sense, for a proven statement which required some effort, or is in some way a final result. In increasing order of difficulty, the names used for different levels of (general) theorems is approximately: corollary proposition lemma theorem (particular sense) Technically, since a proposition is sometimes followed by a proof, it is a theorem in the general sense, but when the word proposition is used the proof is not challenging enough to call the result a theorem in the particular sense. Propositions are minor building-blocks for major theorems, like lemmas. But the word lemma is used to describe proofs that establish statements that are stepping stones for further theorems when the proof is somewhat difficult. The term proposition is used for statements that are easy consequences of earlier definitions, possibly presented without proof; when a proposition is a simple consequence of a previous theorem, the term is a synonym of corollary (which is preferred). In mathematical logic, the term proposition is also used as an abbreviation for propositional formula. This use of the term does not imply or suggest that the formula is provable or true. The word proposition is also sometimes used to name the section of a theorem that gives the statement of fact that is to be proven.
Question: 1) Make truth tables for these formulas: (a) p IMPLIES f (b) NOT p OR q (c) NOT p IMPLIES q 2) Make truth tables for these formulas: (a) NOT(NOTp OR NOTq) (b) NOT(NOTp AND NOTq) (c) (p AND q) OR (NOTp AND NOTq) 3) Determine which of these formulas are tautologies: (a) (p IMPLIES q) OR (q IMPLIES p) (b) ((p IMPLIES q) IMPLIES p) p (c) (p IMPLIES (q IMPLIES r)) IMPLIES ((p IMPLIES q) IMPLIES (p IMPLIES r)) BTW, this is not homework. I’m very short in time!!
en.wikipedia.org/wiki/Truth_table which also explains them much better than I). Create columns in the chart for any sub-terms you need to use in order to build up the final formula. Build up the terms of the expression until you have the entire expression for all combinations of inputs. Fill in for all possibilities. Also, for some things you may need to do a slight translation. For example, 1a) p implies q I would think of as if P then Q which is written P Q Okay, I will try to start you off with one truth table but I don’t promise that yahoo will leave it readable. 1a) P implies Q P Q P Q T T T T F F F T T F F T 1b) not P or Q P Q
P V Q T T F T T F F F F T T T F F T T 1a) and 1b) are equivalent and have the same truth table.
Question: When the conclusion does not seem to follow the premise, but it isn’t negated by it either, what is the truth value of the statement, Specifically for statements that involve contraposition and obversion? For example the statement: All non frogs are snakes(Assume this were true) with a contraposition of all non snakes are frogs. Would the truth value of the new statement be true, false or undetermined?
Answers: Try a categorical claim that works: All non-even numbers are odd. The contraposition: All non-odd numbers are even Given the truth of a premise, the contraposition must also be true. It’s a fact of First Order Logic.
From Youtube
Excel Statistics 89: Hypothesis Testing With Critical Value & p-value : See the Excel functions NORMSINV for critical value and NORSDIST for p-value. Detailed description and examples for steps necessary to conduct a Hypothesis Test about a Population Mean. 1.Hypothesis Testing Test 2.Null Hypothesis 3.Alternative Hypothesis 4.Alpha Type 1 Error 5.Beta Type 2 Error 6.Tests Statistic z 7.Test Statistic z 8.Critical Value and p-value 9.Hypothesis Testing Test About a Population Mean Sigma Known (z Distribution) 10.Conclusions about Statements about whether they are reasonable or not 11.NORMSDIST 12.NORMSINV Busn 210 Business Statistical Using Excel Highline Community College taught by Mike Gel excelisfun Girvin
Principles of Boolean Algebra : Principles of Boolean AlgebraAlgebra of logic is a mathematical device used to record, calculat, simplify, and transform logical propositions. Algebra of logic was developed by George Boole and therefore was named Boolean algebra. It started as a speculative science, accessible only to a narrow circle of scientists. That was until Englishman George Boole bet in the nineteenth century he would create a science completely detached from reality and without any practical application. He turned mathematical logic into algebra of propositions. Boolean algebra is the algebra of two values — true and false. For example, the statement 6 is an even number is true, and the statement The Neva flows into the Caspian Sea is false. These 2 elements — true and false — are called constants and they are assigned, respectively, logical 1 and logical 0. In the algebra of logic, propositions can be combined to give new propositions. These new propositions are called functions of the initial propositions of the logical variables A, B, C, each of which can take 0 or 1.Depending on the values of variables and operations, a function can be either 1, or 0. The values of functions are determined by the truth table of this function. A truth table is a table used to establish a correspondence between all possible configurations of logical variables in a logical function and the values of these functions. All possible logical functions of variables can be formed with the help of 3 main operations .