FRB Finance and Economics Discussion Series Screen Reader Version Credit Spreads as Predictors of
Post on: 12 Май, 2015 No Comment
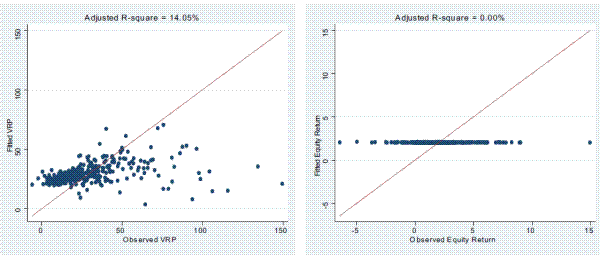
October 23, 2012
Forthcoming in the Review and Economics and Statistics
Keywords: Forecasting, real-time data, Bayesian Model Averaging, credit spreads
Employing a large number of financial indicators, we use Bayesian Model Averaging (BMA) to forecast real-time measures of economic activity. The indicators include credit spreads based on portfolios—constructed directly from the secondary market prices of outstanding bonds—sorted by maturity and credit risk. Relative to an autoregressive benchmark, BMA yields consistent improvements in the prediction of the cyclically-sensitive measures of economic activity at horizons from the current quarter out to four quarters hence. The gains in forecast accuracy are statistically significant and economically important and owe almost exclusively to the inclusion of credit spreads in the set of predictors.
JEL Classification: C11, C53
One area of agreement among economists at universities, central banks, and on Wall Street is that forecasting economic activity is hard. While the existing methods give us some ability to forecast economic developments for the current quarter and perhaps the quarter after that, their predictive power is modest at best and deteriorates rapidly as the forecast horizon extends beyond the very near term. Moreover, what little predictability there seems to be appears to be captured about as well by simple models—such as a univariate autoregression—as by the large number of complex statistical and DSGE forecasting methods that have been proposed in the literature; see, for example, Sims [2005] ; Tulip [2005] ; Faust & Wright [2009] ; and Edge & Grkaynak [2010] .
Economists have long sought to improve on this record by using information from financial markets. Because they are inherently forward looking, the argument goes, financial market prices should impound information about investors’ expectations of future economic outcomes. 5 From a theoretical perspective, default-risk indicators such as credit spreads—the difference in yields between various corporate debt instruments and government securities of comparable maturity—are particularly well suited for forecasting economic activity. Philippon [2009]. for example, presents a model in which the decline in investment fundamentals, owing to a reduction in the expected present-value of corporate cash flows, leads to a widening of credit spreads prior to a cyclical downturn. As emphasized by Bernanke et al. [1999] and Gilchrist & Zakrajek [2012]. increases in credit spreads can also signal disruptions in the supply of credit resulting from the worsening in the quality of corporate balance sheets or from the deterioration in the health of financial intermediaries that supply credit. 6
The empirical success of default-risk indicators as predictors of economic activity is decidedly mixed, however, with results varying substantially across various credit spread indexes and different time periods. For example, the paper-bill spread—the difference between yields on nonfinancial commercial paper and comparable-maturity Treasury bills—had substantial forecasting power for economic activity during the 1970s and the 1980s, but its predictive ability vanished in the subsequent decade. In contrast, credit spreads based on indexes of speculative-grade (i.e. junk) corporate bonds, which contain information from markets that were not in existence before the mid-1980s, did particularly well at forecasting output growth during the 1990s, according to Gertler & Lown [1999] and Mody & Taylor [2004]. Stock & Watson [2003]. however, show that the forecasting ability of this default-risk indicator is quite uneven.
In a recent paper, Gilchrist et al. [2009] (GYZ hereafter) argue that these mixed results may be due to the fact that the credit spread indexes used by researchers tend to be based on aggregates of returns on a mishmash of bonds with different duration, credit risk, and other characteristics. In part to address these problems, GYZ constructed 20 monthly credit spread indexes for different maturity and credit risk categories using secondary market prices of individual senior unsecured corporate bonds. 7 Their findings indicate that these credit spread indexes have substantial predictive power, at both short- and longer-term horizons, for the growth of payroll employment and industrial production. Moreover, they significantly outperform the predictive ability of the standard default-risk indicators, a result that suggests that using cleaner measures of credit spreads may, indeed, lead to more accurate forecasts of economic activity.
This paper extends the analysis of GYZ in several dimensions. Most importantly, we provide a thorough evaluation of the marginal information content of credit spreads in real-time economic forecasting. Given the extensive and ongoing search for consistent predictors of U.S. economic activity, the macroeconomics profession runs a substantial risk that results like those of GYZ are due to researchers stumbling on variables that just happen to fit the existing sample, but which, in reality, have no predictive power. The regular breakdown of new forecasting relationships soon after they are documented confirms that this risk is real. Thus, it is especially important that any such analysis takes into account model search and selection issues.
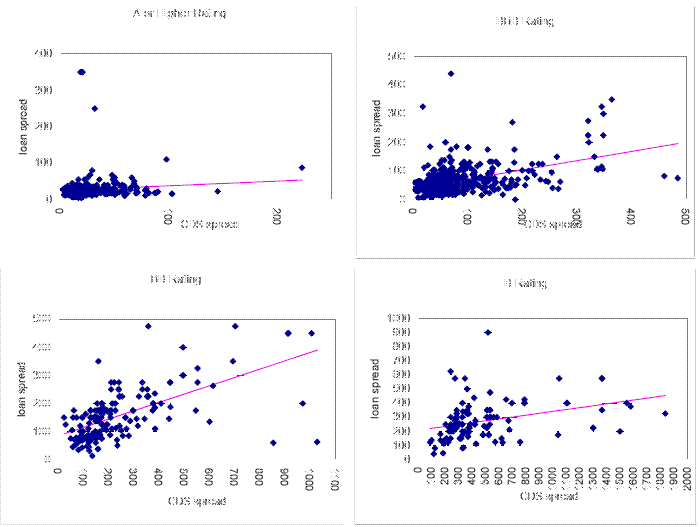
To guard against the problem of selecting financial indicators that just happen to fit our sample, we adopt a Bayesian Model Averaging (BMA) approach and evaluate it in a pseudo out-of-sample forecasting exercise. As explained more fully below, we add the new credit spread indexes to a predictor set containing over 100 asset market indicators, as well as a large number of real variables, and begin with a prior that each predictor is equally likely to be useful in forecasting future economic activity. The posterior weight assigned to each predictor in period is then based on a Bayesian updating scheme that uses only the information available at time . While our BMA scheme has, under certain conditions, a formal Bayesian justification, we follow a large and growing literature that takes a frequentist perspective and relies on the BMA framework as a pragmatic approach to data-based weighting of a large number of competing prediction models 8. The combination of the BMA framework and out-of-sample forecast evaluation mitigates—though does not completely eliminate—the problem of data mining.
While following GYZ’s basic approach for constructing credit spread indexes, we also improve on their methodology by adjusting the underlying micro-level credit spreads for the call option embedded in many of the underlying securities. As pointed out by Duffee [1998] and Duca [1999]. fluctuations in the value of embedded options—reflecting shifts in the term structure of risk-free rates—can substantially alter the information content of movements in corporate bond yields at business cycle frequencies.
Our results indicate that the new credit spread indexes have considerable marginal predictive power for real-time measures of economic activity, especially those of the cyclically sensitive nature. When using the entire predictor set to forecast a wide array of economic activity indicators, the gains in the root mean-square prediction error (RMSPE)—relative to a univariate autoregressive benchmark—are statistically significant and often substantial in economic terms. BMA forecasts consistently generate reductions in out-of-sample RMSPEs on the order of 10 percent when forecasting the cumulative growth of cyclically sensitive economic indicators four quarters into the future. Consumption growth is the main exception to this general result—there are no gains in predictive accuracy relative to our benchmark for this measure of economic activity.
When we omit the credit spread indexes from the predictor set and redo the analysis, we obtain the standard result, namely, that the predictive accuracy of the BMA method—like that of most other documented forecasting methods—is statistically indistinguishable from that of the univariate autoregressive benchmark. This result indicates that there is something different about the information content of credit spreads and that our BMA weighting scheme is able to pick out this difference in real-time from a large number of predictors, all of which were treated equally ex ante. Indeed, the analysis of the evolution of posterior weights that the BMA scheme assigns to various variables in the predictor set shows that it is economic downturns that lead to the majority of the posterior weight being placed on the credit spreads. This finding suggests that corporate bond spreads—when properly measured—may be one of the earliest and clearest aggregators of accumulating evidence of incipient recession.
The remainder of the paper is organized as follows. Section 2 describes our bond-level data and the construction of portfolios based on the option-adjusted credit spreads. In Section 3, we outline the econometric methodology used to combine forecasts by BMA. Section 4 contains our main empirical results. In Section 5, we compare the performance of BMA forecasts at different stages of the business cycle—that is, in economic recessions and expansions. And lastly, Section 6 concludes.