Detetecting Earnings Manipulation and Fraud A Light Tutorial on Probit Analysis
Post on: 30 Апрель, 2015 No Comment
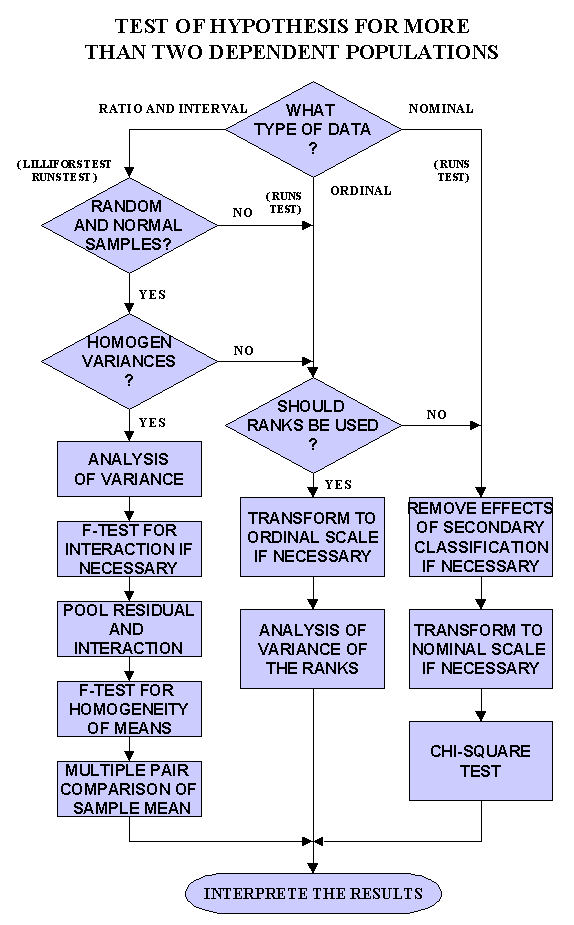
Lies, damned lies, and earnings management. If 20% of firms misrepresent economic reality through earnings management. analysts and portfolio managers must protect themselves by knowing how, why, and when individuals lie. Quantitative methods (e.g. probit analysis) with forensic formulas, such as the Beneish model (developed by Messod D. Beneish ), offer part of the necessary skills to distinguish earnings manipulation from earnings management.
Before we move on, a word about probit. Because so much of finance involves binary variables (even the buy/sell decision is binary), it is surprising that probit is not more widely explored. Knowledge of the basics of probit analysis and Beneishs choices and assumptions can help analysts and portfolio managers get value from his model. Cookbook use of what is termed the Beneish M -model misses changes in the world and can be misleading .
With eight variables, Beneish profiled manipulators as: (1) growing quickly, (2) experiencing deteriorating fundamentals, and (3) adopting aggressive accounting practices. The first two are the incentive variables — admitting to quick but unsustainable growth would surely hurt stock price. For his goal of detecting manipulators, Beneish used a probit model.
Why a probit model? In his sample, there are manipulators and innocent firms — coded as one and zero respectively. Estimating a probability by regressing a binary variable against his eight independent variables would not work, mainly because of the possibility of generating probabilities greater than one and less than zero.
Probit is short for probability unit and is simply another name for a Z -score from the normal distribution. But what does the normal distribution have to do with the binary case of manipulator or not? Well, we assume that the probability of manipulation remains low at first, then increases rapidly, and then levels off as the eight variables go further into red-flag territory. This creates an S-shaped (sigmoidal) curve that can be represented by the cumulative normal.
Although called probit regression, probit analysis is not really a regression. No regression is run with a dependent variable. Rather, probit analysis uses the cumulative normal and finds the coefficients on the eight independent variables that maximize the probability of generating the observed sample.
Beneishs eight independent variables can be divided into a manipulation group and a motivation group, and were structured so that an increase in the variable means a higher probability of manipulation:
- Manipulation signals are. days sales in receivables index (DSRI) for revenue inflation; asset quality index (AQI) for expenditure capitalization; depreciation index (DEPI) for declining rate; and total accruals to total assets (TATA) for accounting not supported by cash. Motivation signals are. gross margin index (GMI) for deteriorating margins; sales growth index (SGI) for sustainability concerns; selling, general, and administrative index (SGAI) for decreasing efficiency; and leverage index (LEVI) for tighter debt constraints.
Motivation to rationalize about unsustainable earnings is driven by the market, and opportunity is provided by financial statement choices. In a 2003 cover story for CFA Magazine. Cynthia Harrington mentions two of the three pegs of what is known in fraud circles as the fraud triangle: opportunity and the ability to rationalize the rightness of actions. Rationalization easily creeps into lying. Aldert Vrijs definition of lying is relevant to earnings management and manipulation: a successful or unsuccessful deliberate attempt, without forewarning, to create in another a belief which the communicator considers to be untrue.”
In misrepresenting economic reality, earnings managers operate within the bounds of accepted accounting principles, but earnings manipulators violate the rules. As a manipulator detector, the Beneish method famously helped Cornell students unmask Enron one year before Enrons collapse. Gray and Carlisle also featured this method in Chapter 3 of Quantitative Value and augmented it with an approach of buying stocks with low-scaled total accruals and low-scaled net operating assets. Joined by collaborators, Beneish updated his work in a recent Financial Analysts Journal article .
Beneish incorporates incentives in his model as he goes beyond the abnormal discretionary accruals that play the main part in the modified Jones approach. Beneishs risk-analytic model is important for investment practitioners and parallels the increasingly quantitative approach of the SEC with its accounting quality model. The SEC model augments factors that indicate earnings management with factors that induce earnings management.
Beneishs probit model (forensic equation) gives an estimated Z -score (he calls it M ):
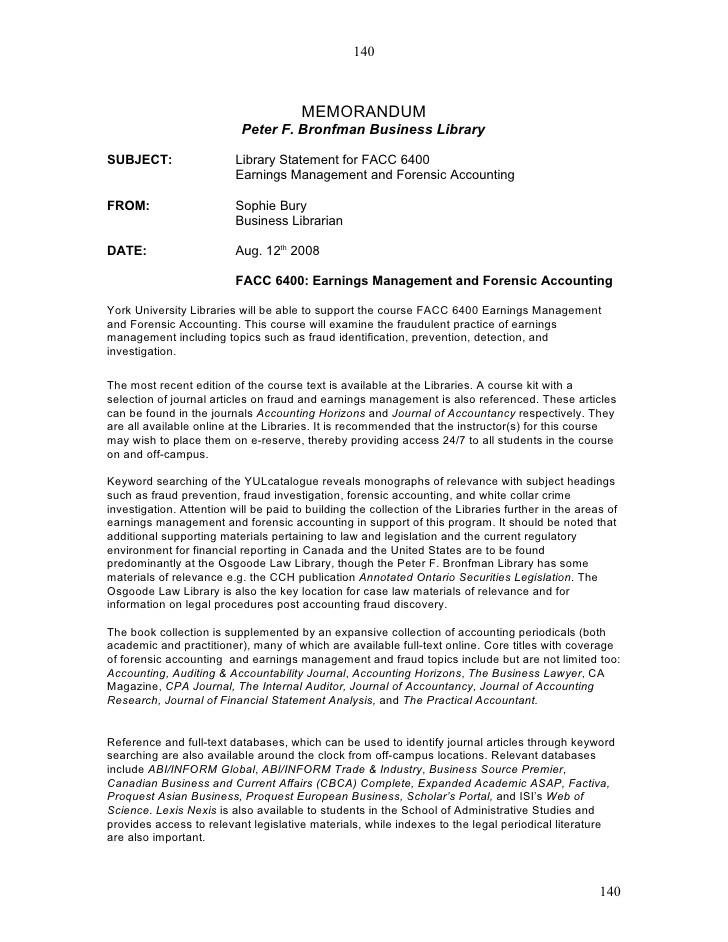
Z -score = −4.84 + 0.92(DSRI ) + 0.404 (AQI ) + 0.115(DEPI ) + 4.679(TATA ) + 0.528(GMI ) + 0.892(SGI ) − 0.172(SGAI ) − 0.327(LEVI )
In their recent FAJ article, Beneish and his colleagues used −1.78 as their cutoff to classify a firm as a manipulator. Firms with Z -scores greater than or equal to −1.78 will be classified as manipulators. Using the cumulative normal table, one can see that a Z -score of −1.78 corresponds to a probability of 0.0375 that the firm is a manipulator. Call it a 0.04 probability. For a firm above but close to the cutoff, and hence classified as a manipulator, the probability of its innocence is about 0.96, twenty-four times the probability of guilt.
Can we gain intuition on the Beneish cutoff? Claiming that manipulators lose about 40% of their value in the quarter when discovered and innocent firms earn 2% in a quarter, Beneish wants to protect portfolios by making it 20 to 30 times easier to call an innocent firm guilty than to call a guilty firm innocent — a ratio consistent with our simple odds calculation above.
On a holdout sample, the model misclassified 50% of the manipulators and 7.2% of the innocent. Although the 7.2% figure seems low, there are actually two kinds of innocent firms: (1) economic earnings communicators, and (2) within-the-rules managers. Only earnings managers and manipulators are likely to venture near red flag territory and be above the cutoff.
We expect that future quantitative models will use probit (or its cousin logit) analysis with more recent data sets and incorporate more detection signals. For example, fraud-detection research based on Benfords Law uses the distribution of digits as a flag; in reports, oddly enough, 30% of first digits in numbers should be one. Word-analysis software now helps the SEC with lie detection. As motivated manipulators become more crafty, portfolio detectives need to keep up.
Please note that the content of this site should not be construed as investment advice, nor do the opinions expressed necessarily reflect the views of CFA Institute.
Photo credit: ©iStockphoto.com/retrorocket