Second Moment Electricity Load and Price Forecasting Using Statistical Methods and Models
Post on: 16 Март, 2015 No Comment
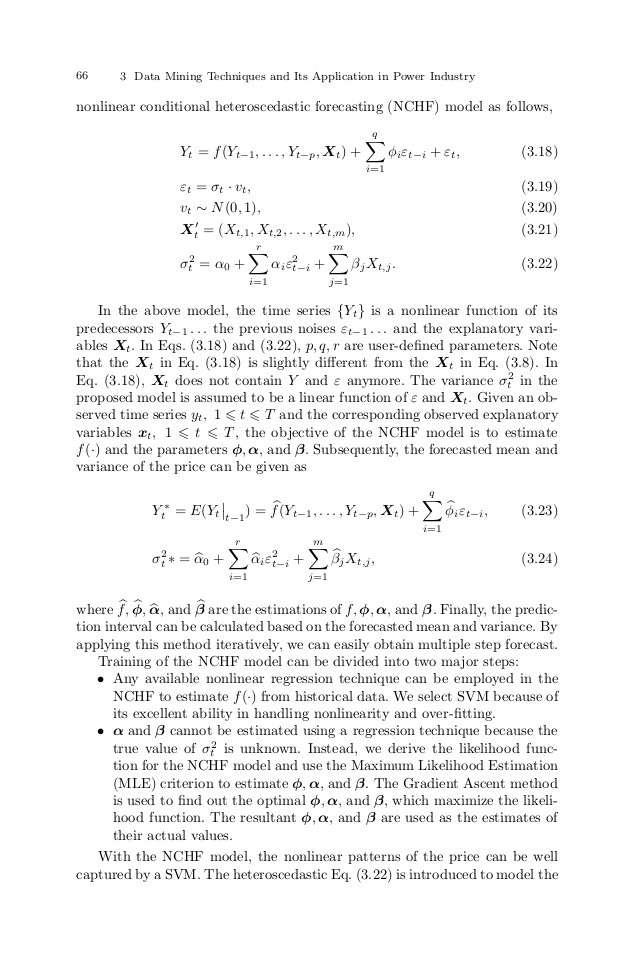
9/11 Remembered
Electricity Load and Price Forecasting Using Statistical Methods and Models
Posted by Dr. Michael Smith
Editor’s note: The following article, which deals with electricity load and price forecasting, was submitted to us by Dr. Michael Smith, a professor of Econometrics and Business Statistics at the University of Sydney. Coincidentally, with the deregulation of the California utility industry, San Diego, home of SecondMoment and the first city in the state to be subject to free-market electricity prices, has seen its electric bills double and even triple over the last two months. Although Dr. Smith’s article focuses on New South Wales, Australia, its implications for California and the many other parts of the world in the process of implementing utility deregulation are clear. Moreover, in keeping with the aim of SecondMoment, it underscores a situation in which the failure to apply appropriate analytical techniques leads not only to unanticipated statistical events, but very real financial burdens for entire communities—individuals, families, and businesses alike. (Our previously scheduled feature on neural networks will appear next month.)
Introduction Over the past decade, many western nations have begun major structural reforms of their electricity markets. These reforms are aimed at breaking up traditional regional monopolies and replacing them with several generation and distribution utilities that bid to sell or buy electricity through a wholesale market. While the rules of how various wholesale markets operate differ, in each case it is hoped that the end result is a decline in the price of electricity to end users and a price that better reflects the actual costs involved. To successfully operate in these new markets electricity utilities face two complex statistical problems: how to forecast both electricity load and the wholesale spot price of electricity. Failure to implement efficient solutions to these two forecasting problems can directly result in multimillion dollar losses through uninformed trades in the wholesale market. This article introduces the two problems and discusses statistical methods and models for their solution.
The electricity load forecasting problem
At any one time, to maintain a particular voltage throughout an electricity grid the amount of electricity drawn from the grid and the amount generated should balance. This figure is usually called the electricity load and, in the absence of blackouts or load-shedding, is equivalent to the demand for electricity. Even before the advent of wholesale markets, forecasting electricity load was important. Short-term forecasts (five minutes to one week ahead) are required to ensure system stability, medium term forecasts (one week to six months ahead) are required for maintenance scheduling, while long term forecasts (six months to 10 years ahead) are required for capital planning. Moreover, since the reforms, load forecasting has become even more important because demand is a major determinant of the electricity spot price.
It has long been known that electricity load is highly predictable as a result of its very strong daily, weekly, and yearly periodic behavior. For example, figure 1 (page 1 of attached pdf) plots the half-hourly electricity load for the Australian state of New South Wales for four weeks in 1992. Apart from the strong periodicity, one thing that can be noticed is that the “load profiles” also vary across season. Most short-term forecasters address this problem by using only a two or three week moving window of data to estimate their model, over which the periodic profile is effectively static. The most important source of variation in load (over and above the periodicity) is meteorology and, in particular, temperature. For a further discussion of the properties of load data, see Smith (2000) and Harvey and Koopman (1993).
Three popular load forecasting methods
Artificial Neural Networks
Historically, Artificial Neural Network (ANN) based solutions have been popular with electrical engineers to solve this problem. While there are a variety of ANN solutions, one of the most popular involves using back-propagation algorithms to select parsimonious radial basis function representations of the nonlinear periodic daily and weekly effects. A temperature input can also be used, although temperature forecasts must be available to operationalize forecasts outside the training sample. Because the level of noise in the data is low relative to the nonlinear signal, these interpolating methods produce quality forecasts. For example, in the Australian state of New South Wales, it is not unusual to produce short-term forecasts with a horizon of one week and average percentage errors of less than 5%, given accurate forecasts of temperature. This is not a statistical approach, however, and does not provide for the predictive distribution of load, including prediction intervals.
Semiparametric regression modeling
The statistical equivalent of the ANN solution is to estimate an additive semiparametric regression model, which also results in the estimation of smooth nonlinear periodic daily and weekly effects. If properly implemented, the forecasts are at least as accurate as those from comparable ANN methods, although there are two major additional advantages of using a statistical model. First, full predictive distributions of load are available. Second, time series models for the residuals can also be estimated, which is a distinct advantage because empirical evidence suggests that there is more structure in the data than just nonlinear periodic effects (Smith, 2000). There is a wide choice of methods for estimating such a semiparametric regression model and past approaches include employing periodic smoothing splines (Harvey and Koopman, 1993) or Bayesian semiparametric regression (Smith, 2000). Kernel smoothing methods with a global bandwidth do not work well because there is a high degree of local variability in the daily and weekly periodic effects. This problem can be overcome using wavelets, although inclusion of an additive temperature effect destroys the computational advantage of using a wavelet basis.
Multiequation models
Unlike electricity load, there are no generally accepted quality solutions for forecasting the electricity spot price. This is partly because the empirical properties of the price differ across markets, partly because ongoing market reform means there is continual fundamental change in the dynamics of prices, and partly because they exhibit complex volatility patterns. In the Australian New South Wales market some early forecasters modeled the spot price (either on a daily or half-hourly basis) using traditional financial geometric Brownian-motion-based models of asset prices. Such an approach ignores the nature of the good being priced. While it is possible to store electricity as potential energy by pumping water to a height and releasing it later, this incurs huge loss and is only possible in the Australian market with a small proportion of system load. Consequently, electricity is a commodity that is predominantly instantaneous and must be consumed at the time of generation. Therefore, the arbitrage arguments that underpin many of the financial time series models do not hold. Figure 2 (page 2 of attached pdf) plots the average daily electricity price in the Australian New South Wales market, with the corresponding average daily load below. This reveals that, in fact, the spot price of electricity is heavily influenced by the electricity load, which, as already pointed out, is known to be highly predictable.
Unfortunately, building a statistical model for the spot price is not straightforward. An economist might say that the price is set at the equilibrium of supply and demand. Therefore, a statistical model that incorporates the demand for electricity (i.e. load) and the available supply could be used. However, it is hard to get reliable supply side data (certainly in the Australian market). Even if historical data is obtainable, forecasting available supply is much harder than forecasting demand. Unanticipated equipment failure or strikes can result in insufficient supply to meet demandsuch events having produced spectacular intra-day highs for the spot in the past. To further muddy the waters, most analysts suspect that the spot price is also heavily influenced by ongoing cyclical bidding behavior of the market participants, most of whom maintain heavily hedged positions.

Towards a price forecasting model: a case for investing in statistical research
Nevertheless, it is commercially imperative that any signal in the level and volatility of prices is modeled. Here, ANN based approaches are not preferable, because it is essential for risk management purposes to forecast the entire distribution of prices, rather than just the mean. Despite a large amount of recent research, it is currently uncertain which statistical models for price are the most appropriate. One approach, however, is to employ a multiequation model of the type used for load. In such a framework, it is easy to incorporate electricity load as an explanatory variable for each intra-day period and the variance of prices can differ according to the time of day, something that is an empirical property of price data. A vector autoregression can be employed to further model the error process. Particularly useful in the Australian market would be a component in the model to capture spikes in the price. One such model is a mean-reverting Poisson jump process, something that has been used with daily price data. The objective of such a process would not be to predict jumps, but to improve the predictive distribution of prices. There is also some empirical evidence that time-varying volatility models (such as the popular econometric GARCH model) may also be useful in accounting for some of the variation in volatility in the mean-adjusted price process.
Conclusion
The recent electricity reforms have created a new market for statistical methods and models. Many electricity utilities are now trading entities and their commercial success is heavily dependent on their ability to address energy forecasting issues. While there are several existing quality solutions to the problem of load forecasting, there is no generally accepted approach to modeling and forecasting the spot price. Companies need to invest in developing models of spot prices and corresponding methods of estimation in an ongoing basis. Greater understanding of the spot price in a wholesale market would not only result in more informed bids and help companies to manage risk more effectively, but the potential downside for utilities of not addressing these problems is clearly substantial.
References
Bartels, R. and Fiebig, D. (2000), ‘Residential end-use electricity demand: Results from a designed experiment’, The Energy Journal. 21, 51-81.
Greene, W. (2000), Econometric Analysis. 4th International Edition, Prentice-Hall: New Jersey.
Smith, M. (2000), ‘Modeling and Short-Term Forecasting of New South Wales Electricity System Load’, Journal of Business and Economic Statistics. 18, 465-478.