Leading indicator properties of US highyield credit spreads_1
Post on: 17 Апрель, 2015 No Comment
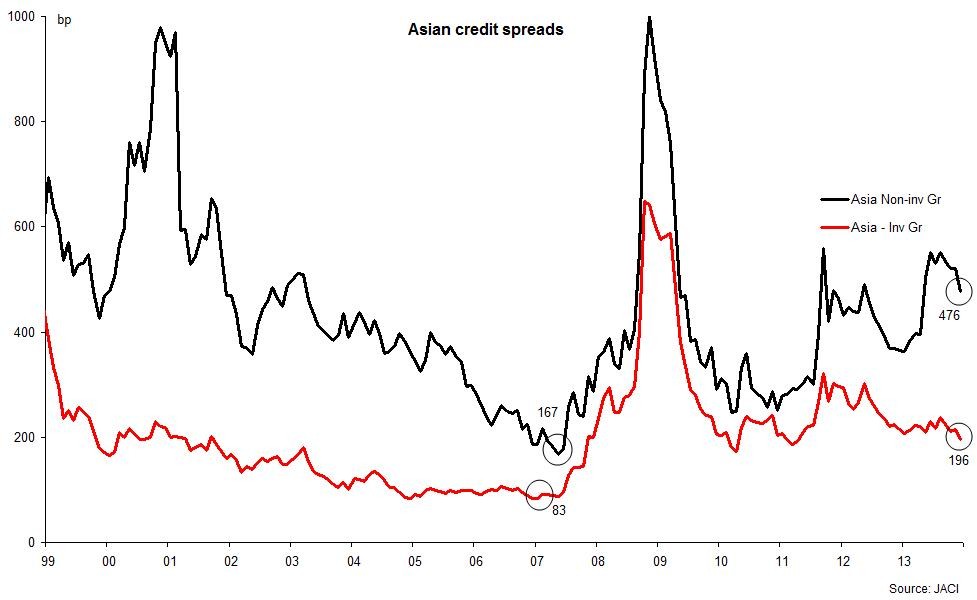
Wed, 07/07/2010 — 13:43 — puput
Previous literature that relates predictions of proxies for real economic activity to financial variables has focused mainly on the information from the government debt market, the corporate debt market and the stock market. The prominent financial leading indicators for policy makers are the inverse of the slope of the nominal yield curve (e.g. term spread, defined as the difference between the 10-year Treasury bill rate and the 3-month Treasury bill rate), the paper-bill spread (defined as the difference between yields on the commercial paper and the Treasury bill) and the return on stock market indices .
It has been documented that these financial indicators have lost considerable forecasting power in recent years. More specifically, a worsening in the term spread predictive content regarding the US recession in the early 1990s has been documented by Haubrich and Dombrosky (1996) and Dotsey (1998). More recently, Stock and Watson (2003b) find that although the term spread did turn negative in advance of the 2001 recession, this inversion, however, was small by historical standards. Furthermore, the study of Friedman and Kuttner (1998) shows a poor forecasting performance of the paper-bill spread. Finally, Fama (1981) and Harvey (1989) show that the linkage between stock market indicators and output growth is unclear, while Stock and Watson (1989, 1999) and Estrella and Mishkin (1998) find evidence of little marginal forecasting content in stock prices.
In this paper, in line with Gertler and Lown (1999), Mody and Taylor (2003, 2004) and Stock and Watson (2003b), we explore the leading indicator properties of high-yield corporate bond spreads regarding US employment and industrial production growth. The high-yield corporate bond spread is defined as the difference between yields on high-yield corporate bonds and the 10-year Treasury bill. Gertler and Lown (1999), and Mody and Taylor (2004) present evidence of strong in-sample predictive power of the aggregate high-yield credit spread. Mody and Taylor (2003), and Stock and Watson (2003b) find good out-of-sample forecasting performance of the aggregate high-yield corporate spread relative to the term spread and to an AR, respectively. This paper contributes to the small but fast growing literature on the leading indicator properties of credit spreads in the following two ways.
First, we are interested in assessing whether it is better to forecast economic activity using the aggregate high-yield spread (as previously done in this literature) or there is forecasting gain from pooling the information in a number of sector-specific high-yield spreads. For this purpose, we use the principal component method advocated by Stock and Watson (1998, 2002) to extract a handful of factors from a relatively large number of sector specific high-yield credit spreads documented in the Appendix. These factors are then used to produce point forecasts for the US real economic activity by using the h-step-ahead projection method. Other related applications of h-step-ahead forecast using factors extracted from a large dataset include those by Stock and Watson (2002) and by Forni, Hallin, Lippi and Reichlin (2003) and Artis, Banerjee and Marcellino (2005), among others.
Second, we are not only interested in point forecast accuracy (as the existing literature using credit spreads has done), but we also focus on the forecast accuracy regarding the 10% worst outcomes in US real economic activity (which includes the 2001 recession with its macroeconomic context) using Monte Carlo simulation. Our probability forecast exercise is related to the work by Anderson and Vahid (2001), Garratt et al. (2003) and Galvão (2006), which obtain probability forecasts of recessions using quarterly GDP data. Our study differs from these studies in that it uses monthly employment and industrial production data, and therefore, it is hard to argue that the obtained probability forecasts would correspond to recessions using this data. Instead, our focus is on forecasting bad outcomes in the two indicators of economic activity. Furthermore, in the aforementioned studies, the probability forecasts are obtained from a dynamic forecasting exercise, while we produce probability forecasts using the h-step-ahead projection method.
Our main findings suggest that the use of a principal component model fitted to a number of US sector-specific high-yield credit spreads seems to improve upon various benchmark models in forecasting (out-of-sample) the US industrial production and employment growth. This can be explained by interpreting the common component to sector-specific high-yield corporate spreads (obtained through the principal component method) as a good proxy of the “systemic” default risk, enhancing the forecasting capabilities of the model relative to different benchmarks under investigation.
The outline of the paper is as follows. In Section 2, we describe the direct forecasting method through different ARDL model specifications, including the one based upon principal components. Furthermore, in Section 2 we also describe the point forecast exercise. Section 3 shows how to obtain probability forecasts by stochastic simulation and how to evaluate their accuracy. Section 4 presents the empirical analysis. Finally, Section 5 summarises the main findings of this paper and concludes them.